Michael DeMaria with Fluke Reliability
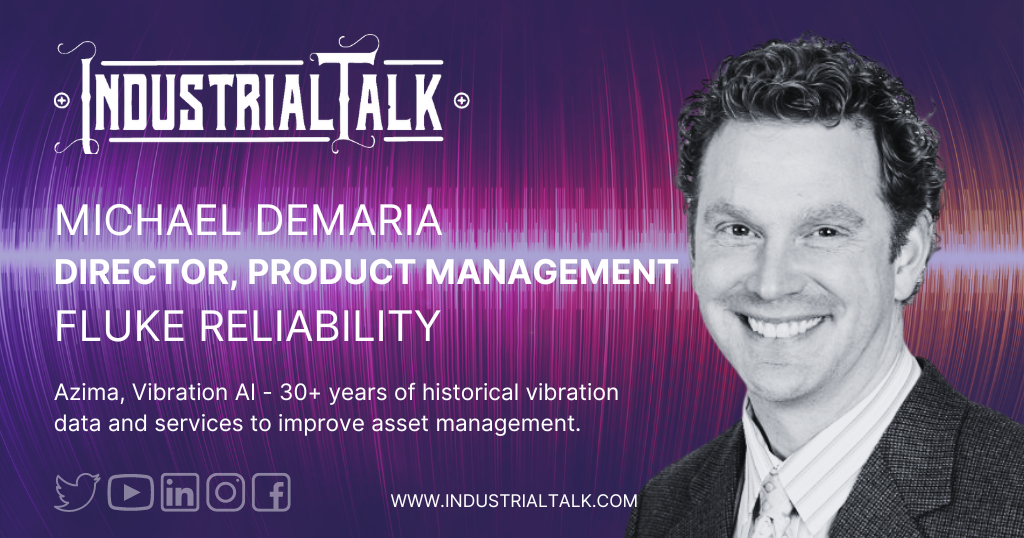
Industrial Talk is onsite at Xcelerate 24 and talking to Michael DeMaria, Director, Product Management with Fluke Reliability about “Azima, Vibration AI – 30+ years of historical vibration data”.
Scott MacKenzie and Michael DeMaria discussed the importance of predictive maintenance in the industrial sector, highlighting challenges in vibration analysis and the integration of AI. Michael emphasized the role of Azima's vibration analysis software in identifying patterns and faults in industrial machinery, while Scott MacKenzie stressed the critical nature of predictive maintenance and the need for timely decision-making. Both speakers emphasized the importance of simplifying data collection and integrating AI to improve machine health and efficiency. Later, Michael de Maria joined the conversation and highlighted the need for persistence logic and ethical considerations in automation. The speakers discussed the potential of predictive analytics in reducing downtime and the importance of a balanced approach combining AI and human analysis to mitigate risks and ensure effective predictive maintenance.
Action Items
- [ ] Interested customers should reach out to Michael de Maria at Azima to learn more about their vibration monitoring solutions.
- [ ] Scott will provide Michael's contact information on the industrial talk website so listeners can connect with him.
- [ ] Michael will continue engaging with customers to help them optimize asset maintenance using Azima's software and expertise. (Michael DeMaria) [Throughout]
Outline
Industrial maintenance and predictive analytics.
- Product manager for Azima shares insights on industrial innovation.
- Michael describes their experience working on aircraft carriers and nuclear power plants, highlighting their background in plant operations and maintenance.
- Michael discusses their work in developing an automated diagnostic system for vibration analysis, focusing on how the software identifies patterns and faults, and prioritizes actions to mitigate them.
Simplifying vibration analysis for industrial machines to improve efficiency and reduce downtime.
- Michael discussed the importance of vibration analysis in predicting machine failure, highlighting the potential for machines to fail quickly without proper maintenance.
- Michael and Scott discussed the genesis of vibration analysis, with Speaker 2 explaining how the method was initially unknowingly developed in the 70s and 80s.
- Developing a data collection system for non-analysts to quickly capture and send data to analysts geographically diverse.
- Michael discusses managing spare parts inventory, highlighting the importance of predicting faults and ordering parts in advance.
- Michael trends the development of faults and plans for repairs to minimize downtime and optimize maintenance cycles.
Using AI to analyze vibration data in aircraft maintenance.
- Michael emphasizes safety and preparation in maintenance, while Scott MacKenzie highlights data-driven decision-making and human analysis.
- Data lake with 100 trillion data points and analyst commentary enables more informed problem-solving and reporting.
- Michael: AI can analyze vibration data, but human validation is crucial (12 words)
- Human component in AI-driven vibration analysis is important (14 words)
AI and data collection, potential risks and benefits.
- Scott MacKenzie: AI tool leverages human knowledge, skills, and directs to right data (0:16:34)
- Michael: AI captures data, but uncertainty on what to do with it (0:17:03)
- Michael argues that more data doesn't always equal better results.
Using AI in vibration analysis for predictive maintenance.
- Michael discusses the importance of AI in predictive maintenance, highlighting its ability to analyze large amounts of data and identify potential issues before they become major problems.
- Michael emphasizes the need for human oversight in vibration analysis, as AI can only provide so much insight and may overstate or understate the severity of a machine's condition.
- Michael DeMaria discusses Azima's product, Fluke, and its ability to analyze vibration data to make better decisions.
If interested in being on the Industrial Talk show, simply contact us and let's have a quick conversation.
Finally, get your exclusive free access to the Industrial Academy and a series on “Marketing Process Course” for Greater Success in 2024. All links designed for keeping you current in this rapidly changing Industrial Market. Learn! Grow! Enjoy!
MICHAEL DEMARIA'S CONTACT INFORMATION:
Personal LinkedIn: https://www.linkedin.com/in/michaeldemaria98370/
Company LinkedIn: https://www.linkedin.com/company/fluke-reliability/
Company Website: https://reliability.fluke.com/
PODCAST VIDEO:
OTHER GREAT INDUSTRIAL RESOURCES:
NEOM: https://www.neom.com/en-us
Hexagon: https://hexagon.com/
Siemens: https://www.siemens.com/global/en.html
Palo Alto Networks: https://www.paloaltonetworks.com/ot-security-tco
Palo Alto Networks Report HERE.
Hitachi Digital Services: https://hitachids.com/
CAP Logistics: https://www.caplogistics.com/
Industrial Marketing Solutions: https://industrialtalk.com/industrial-marketing/
Industrial Academy: https://industrialtalk.com/industrial-academy/
Industrial Dojo: https://industrialtalk.com/industrial_dojo/
We the 15: https://www.wethe15.org/
YOUR INDUSTRIAL DIGITAL TOOLBOX:
LifterLMS: Get One Month Free for $1 – https://lifterlms.com/
Active Campaign: Active Campaign Link
Social Jukebox: https://www.socialjukebox.com/
Industrial Academy (One Month Free Access And One Free License For Future Industrial Leader):
Business Beatitude the Book
Do you desire a more joy-filled, deeply-enduring sense of accomplishment and success? Live your business the way you want to live with the BUSINESS BEATITUDES…The Bridge connecting sacrifice to success. YOU NEED THE BUSINESS BEATITUDES!
TAP INTO YOUR INDUSTRIAL SOUL, RESERVE YOUR COPY NOW! BE BOLD. BE BRAVE. DARE GREATLY AND CHANGE THE WORLD. GET THE BUSINESS BEATITUDES!
Reserve My Copy and My 25% Discount
Transcript
SUMMARY KEYWORDS
machines, data, vibration, ai, analyst, plant, human, industrial, put, fault, analysis, happened, capture, run, talk, validate, identify, repair, Xcelerate, Azima
Well, we're gonna do that one again, just because I didn't have the camera set up right
welcome to the Industrial Talk Podcast with Scott MacKenzie. Scott is a passionate industry professional dedicated to transferring cutting edge industry focused innovations and trends while highlighting the men and women who keep the world moving. So put on your hard hat, grab your work boots, and let's go. Alright,
oadcasting on site, Xcelerate:Yeah, he's doing good. Scott. Good. Good meeting. Yeah, definitely.
Yeah. You were here last year?
I was. Yeah. We didn't connect with you. Last year. We did. When did we somewhere under that? Yeah. Under the stairwell and we?
Yeah. How come? I don't remember.
We were we were a partner. At that point. Philippine, in conjunction. Luke said, we should just bring on this company. Right. So it was Yeah. Because Azima came into the fold. Fits perfectly in between protecting your
you were Azima. Yeah. Doesn't sound like an adult beverage.
Yeah, there's something like that a Azima. Yeah. Never stop with one. So it's gotta be several
more CMOS Azima. Before we get into that topic, can you provide a little insight and
Oh, yeah. Huge when I was in:and gathers? Is this true? Yes. Aircraft Carrier.
So five, 5 million gallons of jet fuel that you carry around? pumps, compressors, refrigeration? Several rescue restaurants, if you will? Yeah. Right. So yeah, all the operations that you would typically see in a city, all the things that you would typically see in a in a power plant. Those are the same, same type of machines.
So how much do the power plants produce? How many mags? What's the size? Because I don't know. Because of my backgrounds, power and utilities, I just want to know,
I'd have to, I'd have to thank you know, it's kind of a because I don't know, go sort of, I wouldn't want to make it up because
somebody's gonna say, You know what?
I gotcha. Yeah. Don't make it up. Yeah. That's sorry, that's classified.
Here's 30. That's even better classified.
But yeah, so moving on from that I ran all the the training department, you know, so educating customers on how to use our solution. And the key thing here is that ours is not. Here. We're going to teach you how to do vibration analysis. Here's how you do vibration analysis. When people look at our solution, it's we'd have to teach them on how how do you train an automated diagnostic system? But the whole background behind our software is the fact that we want the software to do all the heavy lifting of vibration analysis. So how does it identify the patterns? How does it identify what faults? How do they translate into faults? And then most importantly, what action do you have to do in order to mitigate that? So what's what's my priority? And these are the machines that are going to fail, you know, quickly, next week or so. And these are the machines that are super, super healthy. I don't have to do anything.
What was the genesis of it? Like? Was that conversation about why this was an important project to deploy? Well, outside of the obvious, but it's just an interesting, it's been around for 30 plus years. Yeah, what
was what the I think the genesis of it was, we had we proved it out, unknowingly at the beginning. So plants have struggled to have their own technical staff to do vibration analysis at this scale. You know, this is at the end, the 80s. Sure, we started doing vibration analysis in the 70s, with the big carts and cables, tape, yeah, finding the the, the technical things of what's going on. And our our model to it, the way that we approach it is drastically different than everybody else. And so we would write a report, and we have to, you know, a receptionist that would type it all up, they get mailed back to, you know, the plant the chips. And in it, we said, hey, these are the machines that have a critical issue, it's a mandatory recommendation, you have to do this repair, and this is the repair. And these are machines that have serious that are, that are, you should be really thinking about, these are the ones that are, you know, it's a moderate issue is desirable that you do something, but you should plan for them. These are things that are just a marker, a slight fault. It's an indicator as an analyst to find root cause start figuring out the trend, how fast is it propagating? And these are your these are your health emissions? Don't Don't touch them. And what happened was, the plants received that it was like, you know, a month by the time the mail made it to a ship. And what happened was all the machines that we said, hey, you should do these right away, because they're gonna fail. It
failed. Yeah, because it took a month to get the information. It's like, Yeah, no kidding. Yes, right there. Yeah, it did. Wow.
That's No, no other better way to validate your credibility? Yeah, by having you know, that's what you said. And this is what happened. So then, the series went well, and it took certain very seriously, we have to do something with them. But what that got them thinking was, well, what about all these ones that have a moderate issue, something that's starting to develop? Can we do something more? To understand? When do we need to do that? We can't wait another year or a year and a half until another big survey comes by some? Group, right? So can we develop something that we can put in the plant hands of non analysts by technicians, operators, people who know the machines the most, and that they can capture the data. And then we can send the data to an analyst, we don't have to send the analyst, the expensive analyst out to the plant, we can send the data to an analyst geographically diverse anywhere in the world. And so that's that was the first model simplification of the, the data acquisition part. And then the second part is, can we then deliver some immediate understanding? So here's this data, we run it through some sort of diagnostic engine, can you develop a rule rules based system that says that technician or operator on the plant floor could get a immediate understanding of what needs to be done? And so that's, that's where we, that's where we started in?
Did you compress the time from one month to how, how efficient was that, that? That process? Yeah, so
now that there's a simplified data collection system, that you don't have to have a lot of training, a ship's crew turns over all the time. So you have if you have a plant that you can't keep your staff on hand, especially the very technical vibration analysts that are in high demand. So how do we simplify that in a way that a technician or operator this the ones that are more stable within your plant, who know the most about the machines anyway? How can they collect the data very quickly and simply so yeah, instead of once every year, year and a half? Getting a snapshot? Yeah. Now you have it at least once a month, somebody's capturing data. And so now you see that slight fault. And now you can grab it, how fast is that progressing? What's What's the trend? And you could always go fat, you can do it faster. Hey, I met machines that healthy. I don't need to look at it every day. But if a machine starts developing a fault, well now let me see if I can extend that. So if you think about the operations I need to get To the next availability period, the next time I'm going to have a repair cycle are machines. That's what you're trying to get to, can I extend, extend the operation itself? This in a way? Maybe limited? Its, its time in its cycle, I switch to my backup machine, when I can things like this, and say, yeah, now, now here, in six months, I can make it six months, I'll start monitoring it more frequently, because I can just simplification of data. Nowadays, you know, of course, I can put sensors on the machine that will do that for us. But can I get to that next availability? So one of the I think the cool thing is we were talking about managing spare parts inventory. Yeah. Right. And we'll talk more about that in our sustainability sections tomorrow. Yeah. Is, in this example, a plant, in this case, where I came from, carried a tremendous amount of inventory on spare parts, you're out in the middle of nowhere, right? So how do we, how do we ensure that we can repair anything, all the unknowns because you're just wandering reactive, so you have to carry a lot of spare parts inventory. Now, you don't have to, if you can see a fault that's developing? Maybe you're critical machines, obviously, you'd want to be able to be safe and have those type of parts. But you're randomness of all the different bearings you have in a planet that how many machines you have in a plant, you know, how many bearing types? Yeah, okay. Yeah, yeah. So order it in time, right. So I have, I have months of warning that I have a fault that's developing, I can trend it and see how fast that's propagating. So yeah, let's order order the parts. Now, let's plan for the repair, is figure out when is it going to get into the cycle? Again, it's the safest approach, right? They have an understanding about what we need to do, you can have your briefing, and you can have everything lined up ready to go. So the amount of time that it takes to get the machine back on the surface is relatively short. I don't have to wait for the machine to fail and then start figuring out what to do. Yeah.
The goal would be to try to pinpoint it right? Right before its failure. So you sweat the asset in a way that you optimize it, you don't spend the the maintenance dollars or whatever, but you spend it at the right moment at the right time. Exactly. With the with the right solution, right. Now, it's something we think it's this
the right attention to the right person to the right time, with the right parts. You, you've lined everything up to as best as you can for the success.
So with that said, though, so we understand the history and where it came from. And those are critical assets on on a aircraft carrier, they don't want to be out in the middle of nowhere and things happen. And and I would imagine there's some real strategy behind inventory, the parts that you want to carry on a on it, you know, these are critical, this is not so whatever it might be at ease, we can sweat that. But with all that said, it's evolved. It's grown. It's changed, right? Sure. So where do we stand today with it? What what makes it so unique? Well,
the the big difference is now how much data that we have, right, so we have amassed a massive data lake, we have 100 trillion data points in our data lake. And it's all tagged. So make model machines, results, no actual findings. And whether it's cool thing about it is every time we identify when we deliver a result of report to our customer, if we've identified something that is a serious problem or extreme problem, we have analysts commentary, we don't just blindly say hey, that system says this Do it. We have justification, a human review of that data that says hey, this is why this is what's been going on. This is our personal evaluation from the professional analysts that we have. So that's all captured. So the couple of things that we can do, I think it's really kind of fascinating. Especially when you start thinking about how we can put AI into all of us Yeah, is filling in all those gaps. So how many veins do you have on your pump? I don't know if I'd have to pull up the manual how many teeth are on the gear? It's probably not even in the manual. I've had you know customers who've said we want the info and so they're opening up the covers try to count no kidding you you need that info if you don't have it, you can't really analyze for those types of machine faults so you'd have to have those those details and so that's that's things that we can then leverage let's run all of that through you know through our data lake see if we can identify using logic can add two holes
so in the data lake I let's say I have a ball door buffer and or a Baldwin Well, motor and It's this particular brand or make a model Hagan model. here's the, here's the nameplate on it. With that many points, you probably have seen it.
Yeah, right, or something very similar to it. Yeah. So run that through our diagnostics tool, our AI tools, and it will gently it'll spit out, Hey, we think you have 56 motor bars with a probability of you know, this one, this might be this one, this is 30% probability, it could be censored. So then you can start, you know, the human would come in at this point, make sure it's validated, be more, you know, supervised, as opposed to just blindly accepting what it comes up with. I mean, to get there,
I think that's cool. That human component,
I think, at this point in where we are in the AI world, it's important to make sure that you still have an human component in the mix somewhere. And then we see this a lot where people are looking at a vibration solution. And it's, you know, you hear AI and ML on everything. But you, you don't want to make a million dollar repair decision based on just some output, you know, some human should come in and say, Oh, look, you have a steep slope, that's not even good data. Right? Or the machine is not running. So you have a, you're putting a lot of autonomy and vibration analysis now with sensors and how data can get just kind of travel and flow and try to get into systems. But at some point along along that path, somebody should absolutely look at the data before making, making decisions.
I think that that's, that is that is a great example of how AI and and, and the human component work together. What it also does, from what I understand, makes my job the human side more efficient. Yes, absolutely. So it leverages my knowledge, my skills, and it directs me to the right. Well, it's like here, look at this, this is what we got, look at it, validate that it's that way.
Yeah, I think when people talk about AI, you have kind of two camps of the ones that are kind of pushing back a bit. One is, it's being forced upon us and I don't think we're ready. We don't know if we even know what it is, I can spell it. So that's a plus. But beyond that, I'm not sure what I'm going to do with it. And the other one is, this is all going to come take our jobs. Now. And so we're that's not where it's gonna go, I believe it's going to be something more an enabler for you to be more effective in what you do. So
you can remove the AI title from it, just say this is a tool. This is a data lake, this is information that is valuable data valuable to you and your organization. And what we'll do is like we've got that history, boom, you can at least begin the process of being able to pull that information. It doesn't have to be AI, it just has to be the fact that we're able to leverage that information in a way that makes you more operate more effectively. Yeah, makes sense. Right, exactly. So where do you see it going? The what's the next shoe to drop? What what is it? I mean? This is relatively new, by the way?
Oh, yeah. I mean, we've malware and industrial 5.0 coming in. Right. But see,
that was fast. I know. Right? For was last year, it was? Yeah. Really,
I was thinking of it as like, we've been in a like a hunter gatherer stage for a little while, you know, you're putting, there's the IoT, which is kind of the precursor to four ish. Yeah, is now what we want to capture day, we definitely know that data is very valuable, the more data you have to show, we don't necessarily know what to do with it. So but we need to capture it. So you can start out with, we're going to do something with it unless you have. But now that we have it, well, then I think that's it, I try to break down four versus five, I think four was coming up with what you're going to do with it. And five is just putting this new, powerful thing. AI and ML.
Right. Yeah, you know, it's because data is, is everything and everybody's collecting data, and there's gold in that data, whether I, whether it proves to be that or not, it doesn't matter. I'm just going to take everything I can possibly take. Yeah, you know, and I'm gonna bring it in. It's, it's the storage, it's the power associated with how do you how do you continue to pull in all of this information, this data, store it someplace? The the cloud is huge, the server farms and other they're just massive because they're just, it's phenomenal. And then in the case of the vibration, you're pulling in tons of data. Oh, sure. There's tons.
If you're doing it someplace if you're doing it right, it should be sends the data. And there's a there's a risk here where we've started kind of dumbing down the kind of data that you're getting. Yeah. And I've I've asked, I've asked this question with within my audiences to say, does more data equal better results? And that's, that's not true. If I'm not capturing it in a way that doesn't capture a frequency range, for example, that can see the gear mesh frequency. Well, I'm blind to it. No, I'm blind to that information. We were having a table discussion earlier. And the question was kind of being asked, Would you rather have kind of this false information or no information at all? And that it was clear answer? Nobody, nobody wants to be have the false information. If you feel like you're way more at risk, because you're just kind of, I don't know, if you've been kind of led along, or, or the like, or you're gonna make a bad judgment at this point. Wow. So you've, you've seen a lot of systems that want to just give you a quick indicator. But that's not helpful in a plan, you really need to have, you know, an understanding about what I need to do, what's the priority? What's the actual action that I need to take,
see that I think the secret sauce to is, of course, that human component, but But it's taking that tsunami of data, vibration data, and then being able to clean it up a little bit and saying, Okay, this chunk of data, you don't need to see, you know, it just it's yeah, this chunk, go over there, this little slice, this is important, we see it, that it's important, based off of whatever our algorithms, whatever the criteria is, and again, making my life more efficient. But you have to have to continue, you have to keep all that data, because that data is important. But I only have to see this. Right, right. But all of this eventually will be valuable in some way, shape, or form.
So you have 100 100 machines and a plant, how many of them are going to be faulty? Yeah, I don't know what the percentage 15 ish 50%. So that means 85% of your machines. If you're doing manual analysis, you're consuming some resource that has to look at that data. So that's an AI tool, right? So anything that's just mundane and repetitive. Great. automate that. So our systems just push that along. If we're going to, we're automating if we're going to fail, we want to fail as a false positive and not a false negative. So there's no no exact science and vibration. Right? Yeah, it's, it's still, there's a lot of different ways machine can fail. And lots of variables that can lead into it. So yeah, you're you're you will have to plan that you're going to fail one way or the other. So we fail positive. So we don't want to we don't want a machine to fail without us saying something. So often, most time, our analysts will just kind of steer the course a little bit. It overstated if that you have a really bad bearing problem. It's a bearing problem. But you still have a little bit of time, or it came up with driving bearing. And it's actually they're free and bearing I don't know something like this. So we've identified, hey, something's going on. The system might overstate it. But that's where your you human comes in. And let's, let's make sure we put this into context with everything else that's going on in the plan to make sure that it's right. And Nicole, so stuff, so yeah, it's tons and tons of and if you go into the new world of now we put, we're capturing data every day, as opposed to every month, you you still need to have some human look at those 15% of the machines you do. So again, if it's a repetitive task, a mundane repetitive task machine has a fault today, and tomorrow, we're going to capture more data, we didn't do anything. So the machine still has a fault. But now I don't need a human still, assuming I already did his or her job, and steer the course. And so now we can just use automation to say, yeah, if the data looks the same, and no changes in the conditions, and the analyst said, Hey, you should do this. Instead, we follow suit. That's our we have something called persistence logic, and persistently look at what was happening before. What has the human kind of steer the course before and make sure that nothing has changed and then continue that forward.
Cool stuff. How do they get a hold of you? Yeah, how do they get a hold of you? They say, hey, I want to get a hold of you. Oh,
cardiac deaths that flip Reliability.
Are you out onto LinkedIn?
I'm on LinkedIn. You
e broadcasting from Xcelerate:You're listening to the Industrial Talk Podcast Network
duct, the event was Xcelerate: