Alok Aggarwal with Scry Analytics
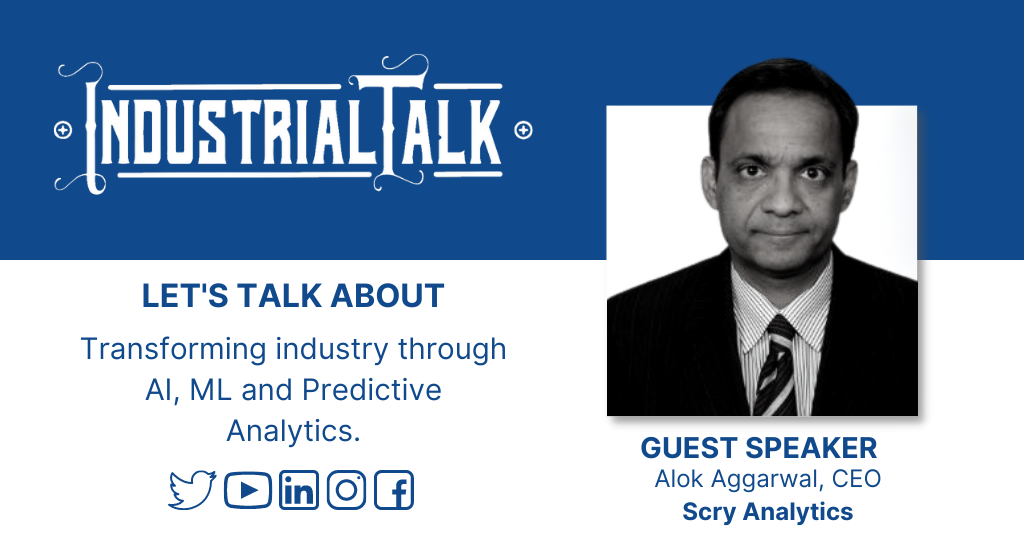
On this week's Industrial Talk we're talking to Alok Aggarwal, CEO of Scry Analytics about “Transforming industry through AI, ML and Predictive Analytics”. Get the answers to your “AI/ML” questions along with Alok's unique insight on the “How” on this Industrial Talk interview!
Finally, get your exclusive free access to the Industrial Academy and a series on “Why You Need To Podcast” for Greater Success in 2022. All links designed for keeping you current in this rapidly changing Industrial Market. Learn! Grow! Enjoy!
ALOK AGGARWAL'S CONTACT INFORMATION:
Personal LinkedIn: https://www.linkedin.com/in/alok-aggarwal-0a521/
Company LinkedIn: https://www.linkedin.com/company/scry-analytics/
Company Website: https://scryanalytics.ai/
PODCAST VIDEO:
THE STRATEGIC REASON “WHY YOU NEED TO PODCAST”:
OTHER GREAT INDUSTRIAL RESOURCES:
NEOM: https://www.neom.com/en-us
Hitachi Vantara: https://www.hitachivantara.com/en-us/home.html
Industrial Marketing Solutions: https://industrialtalk.com/industrial-marketing/
Industrial Academy: https://industrialtalk.com/industrial-academy/
Industrial Dojo: https://industrialtalk.com/industrial_dojo/
We the 15: https://www.wethe15.org/
YOUR INDUSTRIAL DIGITAL TOOLBOX:
LifterLMS: Get One Month Free for $1 – https://lifterlms.com/
Active Campaign: Active Campaign Link
Social Jukebox: https://www.socialjukebox.com/
Industrial Academy (One Month Free Access And One Free License For Future Industrial Leader):
Business Beatitude the Book
Do you desire a more joy-filled, deeply-enduring sense of accomplishment and success? Live your business the way you want to live with the BUSINESS BEATITUDES…The Bridge connecting sacrifice to success. YOU NEED THE BUSINESS BEATITUDES!
TAP INTO YOUR INDUSTRIAL SOUL, RESERVE YOUR COPY NOW! BE BOLD. BE BRAVE. DARE GREATLY AND CHANGE THE WORLD. GET THE BUSINESS BEATITUDES!
Reserve My Copy and My 25% Discount
PODCAST TRANSCRIPT:
SUMMARY KEYWORDS
ai, industrial, hype, people, electricity, repurpose, world, industry, devices, listeners, create, iot, conversation, apps, motors, analytics, alok, big, article, sensors
Hey, industrial Talk is brought to you by CAP Logistics. You want to minimize downtime. Absolutely. increase reliability, you bet ensure operational profitability. Yes you do. That means you need 24/7 365 insights into your supply chain. Look no further CAP Logistics, go to Capitol Logistics.com Or just call them. They're great people 800-227-2471 and AI Dash Now we all know about satellites. Yep, they're up in the air and they're looking down. How about AI Dash combining AI technology to be able to give us greater insights into our vegetation management into hazards that exist out there in our industrial space? Yeah, that's powerful. That's tactical. That is insightful. That's AI Dash go out to AiDash.com Find out more. They're doing great things in the world of AI.
Welcome to the industrial talk podcast with Scott Mackenzie. Scott is a passionate industry professional dedicated to transferring cutting edge industry focused innovations and trends while highlighting the men and women who keep the world moving. So put on your hardhat grab your work boots, and let's go Alright, once
again, thank you very much for joining industrial talk number one place that celebrates you, the industrial heroes all around the world. You know why we celebrate you? Yeah, you got to ask that question. Why we celebrate you here on industrial talk. It's because you are bold. You're brave. You dare greatly. Absolutely. You're solving problems. You're changing lives in a positive way. You're changing communities, of course, you're changing the world. That's why this platform is here for you. It's a celebration. All right. hotseat time. The gentleman's name is a Alok Agarwal. Now he is the CEO and chief was was a chief data scientist at Scry Analytics. That's S C R Y. And this is an important topic. And you know, it is it's not out of reach. We've got to talk about AI, machine learning. And of course, predictive analytics. That's what a look brings to this particular conversation. Let's get cracking. Yeah, yeah, let's get cracking. You ever talk to somebody. And you realize, Wow, I don't know a fraction of what that individual is talking about. Now a Alok does a great job. He, he brings the history of that is around AI machine learning the history which which I'm passionate about, how did we get here, you always got to look at the history. And of course, a look brings all that. But it was just that you're going to enjoy this particular conversation. All right, he just are because he brings the lumber and the truth. And it's just a great conversation. And hopefully we'll do more. All right. So I'm out walking the dog. Because that's what I do every morning. My dog keeps me young. So I'm out walking the dog and always thinking I'm thinking about things. This time, I'm thinking about all of the I guess blogs or white papers or articles that exist out there today. It's AI. It's a lot, right. And in there in all of those are, if you look at him, you stack them all up, right? There's just tremendous solutions, tremendous wisdom, tremendous insights, all buried in there. But they're buried there. They're deep in the bowels of these blogs and white paper. And I was thinking if you're doing and I don't mean to be provocative. Yeah, I do a little bit but let's just say I'm, I have a certain thought about all of these blogs in these white papers. Because I think we're at a point where I know that I'm inundated by just information coming out of that terminal. It's like hitting me right in the face and it's all this other information and I'm just me, I wish I wish I was a computer because I would soak it all up, but I'm not right and it's hard to to consume it. So I think that there has to be a shift because right now I don't think people maybe a few maybe if I'm dedicated to a specific location, I'll read the articles. But I know that for me, I'm being changed for the DNA out right. And I I'm one that likes to be see it be entertained, talk about it. And I think a lot more people are doing that as we speak. So with that said, walking the dog, and I came to the conclusion that, wouldn't it be great to dust off these, these blogs, white papers, whatever, repurpose them into a real human centric conversation, record it, look at it. So the format would be, hey, hey, you wrote this article, you got street cred, man, you've got that thought leadership going on. There's the article, let's talk about it. Let's talk about the key points, it's sort of the cliff note version, but at least it gets to it. And then if that if the listener, because that's easy, right? I don't have to pick anything I have to do is just sort of listen, if I'm the listener, I don't have any friction, I could go back and say, you know, I want to read that article, I want to get that or I want to, you know, whatever action call to action there is. So I just,
I really would like you and others to think about how you can repurpose these wonderful, absolutely brilliant articles, or blogs, or reports, or white papers, and repurpose them into a conversation. And no matter how you do that, that would be great. It'd be great on the industrial talk podcast. But the reality is, if I'm, if the industrial talk is here, specifically to educate, okay, and to collaborate, and to innovate, and all of the things that are happening within industry, that has to be part of it. And and we have to get that message out this, this idea that the article will somebody might might find it or whatever it might be, or, or we'll send it into an email marketing campaign, whatever it might be. We're just inundated. So we've, we industry have to really think about how we repurpose those, those rich content, Insight driven, reports, some blogs, but repurpose them, figure out how to do that. And, and come on the industrial talk podcast, because I'll definitely, that makes me want to read them and makes me want to talk about it makes me want to share as much as I possibly can. Because the listeners and industry as a whole, we got to keep on learning we got to keep on a grove and all right. So I'm at a Alok in California. Again, he's smarter than me, which is a lot, don't be offended by it. But that's not a very hard bar, but you're smarter than me. And what you'll gain for this particular conversation is the fact that you'll see him and his passion and his desire to be able to utilize data in a way that is predictive learning, and all of the stuff that's associated with that, and, and be able to help us in the world of industry in the world of manufacturing in the world of utilities, you name it. Help us do our jobs better. And and locusts is just an absolute delight. Enjoy the conversation with my friend. And now your friend. Oh, look. Alright, look, welcome to industrial talk. How are you
doing today? Great. Thank you for having me.
I am so excited about having this conversation. I mean, you bring a wealth of knowledge. But instead of me telling the listeners the wealth of knowledge, your stat cards impressive out on LinkedIn, give us a little background, a little 411 on who a Alok is.
So thank you, I get my PhD in Computer Science from Johns Hopkins in 1984, which included algorithms part of basics of machine learning computer vision, something called computation geometry, which is goes at some basics, joined IBM Watson Research Center, which is about 40 miles north of New York City worked for them for 16 years during this period, taught at MIT for a year came back we used actually machine learning in 1991 to 95 timeframe for improving paper mills steel mills, so actually put it in practice during that period. There were no open source. So we actually wrote our own algorithms, then became the Director of Strategy and after that started the IBM India research laboratory 230 PhD study masters during 1998 and 2000. Then I quit to start another company called evaluator which has not much to do with AI and machine learning. The co founder is still running it we started in essentially in January 2001 December 2000. And it's about 3000. 500 people worldwide, but I wanted to go back to my roots and started scrying. It's in 2014 in February 2014. Basically, doing machine learning AI and building products and what we call enterprise software apps. We are built, we have built about 26 of their apps. Scry the word itself is an interesting word. It means you can use it in Scrabble, you can actually
yeah, it's a powerful word. I kept screwing it up. But now I know it's Scry
Yeah, you can convert practice crying, calm Scrabble, and people will be looking at you, especially your wife and kids that you've gone crazy. And, but it means crystal ball gazing or fortune telling. So Nostradamus is considered the biggest crier. And since we're doing a lot of predictive analytics, we are essentially predicting the fortune misfortune of companies, individuals, entities and so on. And hence the words cry. The company is based on a thesis that actually unlike the thesis of 1950s, in AI, the hype in the bus which was they will create a general purpose AI machine often called artificial general intelligence, which Hal 9000 was portrayed as in Odyssey 2001. Space Odyssey, were one of the founders, one of the advisors to Space Odyssey 2001. Space Odyssey was Marvin Minsky was one of the actually considered as one of the three fathers of AI. He, this was kind of his imagination of Hal 9000 as the artificial general intelligence computer, and those of you would have seen it wouldn't understand what he was talking about. The hype went bust in 1977, and one of the other fathers of AI, McCarthy, he's one of the New York Times reporter asked him 1977. So what do you think of artificial general intelligence? When do you think it will actually happen? The hype had gone bust. And he said very nicely, maybe in five years, maybe in 500 years, what we need is 1.7, Einsteins and 0.3. Manhattan projects. For those of you who may recall, Manhattan Project was the one, which basically helped us create atom bomb to end the Second World War. For better or worse, I mean, that was what Manhattan Project was. So this time around our thesis is that there will be a Cambrian explosion of AI apps, very much like what happened with pumps and motors after the Industrial Revolution. And today, motors are pervasive. In fact, in my room, there are at least three motors running one,
I have to interrupt you when you said we had this conversation a couple of days ago. And he's, and I didn't follow up on it when you said you got a couple of motors in your room. Because you got to know where I'm going. I'm just sort of thinking motor. What do you mean by that?
Yeah, you have a motor and a fan, right? Your motor in your PC, your motor in your laptop? So three motors running, right? I mean, we don't even think about it. That's what the whole point is, right? What do you mean by that we have motors running. Yes, there are three motors running. And that is what will happen in the according to our thesis in 2035, there'll be 1 million different apps running all over the world, which will be commercialized, which will actually be providing useful. The utility useful KPIs, reduce costs, reduce energy, and so on. So with that, as the thesis, we decided in 2014, that we will create a bunch of apps to be more precise, two dozen apps, and we are pretty much there. And we're not selling them. And working with some of the top tier firms, providing them very high end services and selling our products, or what we call enterprise applications, because they all work with using API's. So that the people do not have to destroy their current solution. They can just put it, plug it in. And that's why these are That's why they're called apps.
So what industry do you tip at Scry? What industry do you normally focus in on?
So two industries we are very big on one is related to financial services, particularly banks, we're gradually going into insurance also banks. asset managers are our biggest industry so to speak. Second is related to IoT particularly Industrial Supply Chain Management, smart cities, that's all where IoT gets used. In fact, one product is quite, quite horizontal called Malaysia which comes a Latin word for coalition and putting together it's putting together a diverse set of data sets, which is sold we're selling to banks for mortgage operations for small medium business lending for KYC know your customer and ID checks and verification for onboarding, etc. The second one is called consensual again, a Latin word for consensus, often called out concordance or harmony. And that is all related to enterprise apps related to IoT, smart cities, and so on. So those are the two big ones. And then, of course, technology Telecom, ends up using quite a bit of IoT. So we will gradually, we are already selling to a few companies in technology and telecom. But we'll probably sell much more,
are you are you considering the IoT solution, the app in a manufacturing type of environment,
we are already selling it to manufacturing environments. So in particular, one of the very important apps, which people often forget, in IoT world is that the devices or sensors may actually go back to the data coming in itself may not be good. And if the data incoming data is bad, then obviously all the analytics that can be done will be bad. So one of the sub products of consensual is called IoT doctor. And basically it is for the health, checking the health of the sensors and devices, because sometimes the sensors and devices may go bad themselves. For example, unfortunately, somebody lights us cigarette close to a sensor, the temperature will go up, the smoke will go up, carbon dioxide will increase. And if these are some of the things that the sensor is measuring, or the device is measuring, obviously, it will have a spike. Similarly, maybe there is a pipe in a manufacturing plant, which is emitting steam just to let the pressure off every two minutes. Then during that period, the device which is close by could have strange readings, the other part where sensors and devices may not go back, but where they may not be able to communicate effectively with the cellular station is because of weather and so on. So many things caused that. And that's the first thing that you want to make sure is that the data is at least 95%. Correct.
That's interesting, because I never really thought about it. There's been a plethora of of IoT, the conversation, IoT, everything that's going out there splitting the devices out on these assets, all of that fantastic by digital transformation. Got it. But I've never really spoken to anybody that talks about, hey, we, the device eventually could fail,
right? Yep, the device could fail, the battery could go down a single failure as the battery goes down, right? But the device could fail in many, many different ways. I mean, we know all the equipment fails sooner or later. So how do you know it hasn't failed? And it may feel temporarily or common?
And senior? You're compressing that time, right? You might, and that's where the AI solution is to say, Hey, we got a problem. Compressor time somebody goes out, replaces it does whatever it has to do. Yeah. And affect the change quicker.
Which Exactly. And if it's a smart water meter, for example, I mean, San Jose Water Company doesn't have to go out, they can just tell me Hello, can you please do us a favor? This is where the battery goes? Can you please put it in? Because it's good for you? It is good for us. It's good for you. Because if you have a burst or a leak in your pipe, a smart water meter will immediately tell you as an alert. That's what has happening. It's good for us because we're getting data in real time using the cellular device.
Yeah, yeah. And then when we start talking about API's, right, you don't have really any limitations API's allow you to take your apps and be able to plug and play to. And that's
the reason why we call them enterprise apps. I mean, it's very much like, I mean, I would say a smartphone app and iPhone app, but the difference is that these are for enterprise is not for individuals, and you can literally plug in there and and get going in a matter of a day or two with the whole process new process.
And from from my perspective, what are we talking about from an ROI perspective, what what are we are we are we looking at a an expensive solution or something that is economically viable
move, these things are extremely economically viable for two reasons. One is what I would call Moore's law, which basically, Gordon Moore who was the founder of Intel, in 1965 hypothesized that the price of semiconductors will go half in two years or the number of semiconductors on the chip may double every two years, which would I mean, this is an exponential thing right? If it implies that in 20 years, the price will go to the 10 times I mean the number of chips or semiconductors which is on 1000 times and of course we have not been in this game for almost 60 years. So it's Not surprising that it has gone up at least a million or 10 million times, if not a million times. So this obviously reduces the price of computation reduce the price of devices substantially. The second reason why these things are also quite inexpensive is there is a lot of open source software. So I was talking about 1991 to 1995, which is when we had to build our own machine learning algorithms and code them, the algorithms were there, we had to code them, right. So everyone had to code their own algorithms, there was no open source, which was literally free of cost, where you can start from literally not from ground zero, but probably from cloud to going up. So all of this has made the systems quite inexpensive. I mean, even for industrial systems, where we sell a platform or the product together, for IoT, Dr. It starts as low as $30,000. For a year for, for small, for a small manufacturing plant. I mean, that's $2,500 a month, roughly 1/3 or 1/4, the cost of the operator who's running it,
and see what's interesting, what you're talking about is constantly being driven down. Because yeah, as greater adoption happens, right? More people are gonna say I need I need it. And that whole, that whole juggernaut that wants to move forward definitely will continue to drive down the prices,
absolutely. And open source will continue to drive the prices. And I mean, if you look 15 years ahead, not five years, quantum computing will be the next thing which will be after Moore's law because more employees saturating now, but that will begin to drive down the prices, because you can do much more computing using quantum computer. So I think there is a lot positive that's going on, for the humanity worldwide, because suddenly, it's not only us, or Canada, or OECD countries that can use it. I mean, countries like India, China, and China, agencies are still much better. But India and other poorer countries are full time using it.
So that's a beautiful thing alone. That's that's, that's transformative. And that's not out of the reach of small companies, big companies, it doesn't really matter. It's, it is truly transformative. And we better the world as a result of that. And I yeah,
I mean, I'll give you one example, which is very, very interesting. There is a company which has set up a $2 billion plant in India, which is going to create 2 million electric powered scooters. Now India is a pretty dense place. I mean, scooters, motorcycles are used all the time. And these will be all electric powered scooters by 2025. If they continue on the path, the gas gasoline powered scooters will be and motorcycles will be all gone on our ability literally replaced. And all of this is happening. If you look at it, it is all comes down to the ability of Moore's law. And I mean, the ability to produce things much cheaper and better. both hardware and software.
So that's, that's, that's pretty cool. Come on. I mean, listeners, you gotta you gotta sort of sit back and say, Yeah, that's the future is pretty god. Cool. I like it. Now. You had me, it's all cool. The conversation that we're having right now it is really bitchin. Now. What are the what are the what's the big problem? What is that? You know, this is all great. But you know, there's always robots. What is it? Yeah,
exactly. All hypes eventually come hate against a wall, right. I mean, I mean, I'm sure the three founders are the fathers of AI. Turing. McCarthy and Minsky are turning in their graves and saying, what, what did we do wrong? Because they're five hit the wall in 1975. We are on a course where this hype could also it wouldn't go bust. Even the previous hype had a lot of positives we wouldn't have. We wouldn't be working on where we are working, if it weren't for that height. So I'm a very big believer that hypes have very positive things to do for the society. For example, people think deep learning networks came recently. Soviets as part of that hype in 1965 Eva Hankel in Soviet Union now in Russia, actually already created an eight layer deep learning network with his student Lapa, they already gave an algorithm in 1970 How to Train such a network. Now why were they not able to do it again comes down to the price of semiconductors and the price of these right? It was so expensive in 1975. If you had to create 86 billion neurons which artificial neurons which exist in a typical human, it would have cost the entire GDP of United States, which was $3 trillion. Right? So that's why that hype fell apart. Now what is going to slow down this hype, or what we'll need to think through how to break through that barrier, is that as these machines at the more we train them, and the more accurate they become, then rely on more and more variables or parameters, as we call them. And to train on those parameters, you need much bigger, deep learning networks, and therefore you need much more electricity, because eventually it comes down to servers. So some of these things, I mean, we're already seeing in Bitcoin is a very good example, where you have a blockchain and it basically ruined one of the cities close to Quebec completely, because miners when they're Quebec, the city said, Okay, we'll give you electricity for rather cheap rates, all miners went there. And literally, they had to raise the rates after a few years, because they did not have electricity to give to the locals. China has same problem. They also realize, Oh, my God, we because a lot of these miners in Bitcoin, were working in China. And they suddenly realize, Oh, my God, we are working, we're not creating electricity from fossil fuels. And here we go. So that's one example. But that's the one can argue that a standalone example. But I'll give you another example. GPT three, which is a natural language processing, natural language generation. These guys actually trained it, they spent about $4 million that we know of, I mean, we don't know the exact numbers, they trained it for such a long time, the amount of electricity that was useful so much, that they actually made a small mistake in their algorithm. And they didn't want to they said we didn't want to retrain it because it would have been just too expensive, both timewise money wise, and electricity wise, it said, I mean, in parenthesis, they did not say it out loud. But that was. So here are some of the examples. I mean, Google never tells us as to how much it is training. But but our estimate is that it is screening for the Google ads and what people are, they're using at least two to 4 billion variables, which means they have a farm, which is running in easily in 100 million servers, or there abouts. Now imagine how much electricity that's going to take. So So you have a climate change where AI can be very helpful, you have just like motors and pumps, which use energy, you will have aI motors and pumps equal. And
this is so interesting, because I, I remember that all of a sudden had a few years ago that I never I get it, somebody says, hey, just stored in the cloud. And of course, that's a learning curve for me and going, what the heck does that mean? Right stored in the cloud, and then all of a sudden, everything is in the cloud. And then I just, I've just thinking to myself, Who the heck is providing the power for all of this, and it must be just absolutely insane. Because, you know, every time I take a picture, video, cloud, everything's in the cloud.
And that's where it will do a lot of innovation will have to be done. I mean, something some of the good news is that in theoretical computer science and math in physics, some of this has been going on. That's why I'm a very big proponent of basics. theoretical work basic sciences, like how do you call these machines? What do you do? Okay, when you call these machines, you have hot water, then you recently this hot water? How do you get all this energy? Electricity? I mean, wouldn't be surprising if this continues if the world requirement for energy for AI machines will already exceed that us by all air conditioners in the world. So So there we have it. I mean, we'll have an interesting, a cycle where it will hype will hit reality.
Yeah, because you're sitting there, you're pulling on the thread, you go, Hey, this is great. This is great. This is great. And all of a sudden, somebody like that story you told us about that learning, and they just did they made a problem with the code. Yeah, code back. It's like, can you imagine sitting around, it's a darn it. I mean,
honestly, I mean, because we do a lot of research and advanced development in Scry, one of our PhDs he came to me and I said, Hey, he said, Look, we made a small error here. And I said, Why don't you train it? He says, I love really, you want me to train it for this small error? It will take me two and a half weeks. I don't know whether a small error is fixed at this point of time. So this is already happening with us. I mean, imagine you multiply that million times 10 million times and particularly contract I mean cities, giga cities like Neo, and other mega cities coming up with our smart cities, where this is what they will be based on. And you suddenly say, yes, the good news is neum, as a smart city is in a desert, they get sun all the time. But they use that sun very efficiently, and very cheaply or inexpensively to
produce all this electricity. It's true. And then there's the physical characteristics of power. And there's the force, there's, there's things that are just a part of power, that keep and keep a community, like, like, I look at the lights here, and there's just, it's, it's power that's pushing, it keeps it stable. There's a force there, you know, I mean, these are, these are difficult questions.
These are interesting questions. I mean, that's exactly how interesting paradigms get created, how hypes get created, how they hit the wall. And it's no different than what happened with physics, right? I mean, we hit the wall before Newton came around, Newton gave Newtonian mechanics and that continued for a few years for a couple of centuries, hit the wall again, then Einstein came around. So it's all up and down. But the overall curve is, is on the positive and direction. And I gotta
tell you, that you're the first one that really addressed the fact that this is all great. But it requires power, it requires energy to do this, right, whatever this is, it requires, and it's, it's hot. So it requires a lot of power. And as you continue to scale more, and more and more, and and, and so we wrestle with, we want a sustainable future, and use sustainable and renewable type power. And yet we want this innovation to, and it's a balance. I mean, I'm glad you're smart. And I'm not
nothing. I think this happened, the same thing happened in the Industrial Revolution. That time, of course, we were unaware of its effects on climate. But we all realized that it required fossil fuel and energy to run pumps and motors, engines and all that good stuff. I mean, these are the new pumps, motors and engines, which are, I mean, in some sense, you can I say their software, but they still running on the hardware underneath, which still requires energy, which still requires cooling.
Yeah, yeah, you're absolutely. Yeah, I'm gonna tell you, it's like it. You know, you had the Industrial Revolution. And then we had all these talking about all the industrial and now we're at four Dotto, right. And, and it's still it's just it's a, it's a energy centric type of solution. Great thinking, great minds. Gotta have it. Gotta have that energy, some way, shape, or form. Brilliant. Brilliant. We got to wrap this up a look over my time limit.
Thank you so much for having me. I mean, I love to be part of whatever you folks are doing, because you're great. I mean, absolutely. It's exciting
times out there. And you're leading the way. And I and I could geek out on this stuff all day long and talk to people to go, oh, my gosh, that's cool. That's cool. And for me, it's hard to sort of maintain my composure. How do people get a hold of you? Look, what's the best way?
I think email is the best my first name dot last name at scribe linux.com. I would say that is I actually respond much faster and quicker to email than to my whatsapp or text messages.
Yeah, you're pretty. You're good at that. I have to. I have to admit you're very good at that. And that way Scry SC, r y. So you listeners out there ascribe analytics, a lope? How do you say your last name?
Agarwal? A? I mean, if my parents knew how to pronounce these things properly in English, they would have used you rather than me. So it should have been UGR AWS, but I'll look at what
yeah, you gotta reach out to him. Yeah, listeners, you're not going to be disappointed. You got a lot of passion, man. I like it.
Thank you. Alright, let's
do this. We're gonna have to wrap it up on the other side sphere not you're gonna have all the contact information for my friend. Look at anything else that you'd and don't come to me and say, Scott, I can't get ahold of the load because he is responsive. All right. Thank you. Staying Scott really enjoyed it. Joe fires there, my friend. Stay. We'll wrap it up on the other side.
You're listening to the industrial talk Podcast Network.
All right. Once again, thank you very much for joining industrial talk and celebrating industrial heroes and learning from the very best in industry. Thank you to a loake for being on The industrial talk you go reach out to his stat card out on LinkedIn, I'm telling you will not be disappointed. Scry analytics SCR y analytics is the company. Come on facing. You need trusted individuals that know what they're talking about in the world of AI, ml and of course, predictive analytics. Alo, all right, I'm gonna challenge you. You're gonna look at those blogs, you're gonna look at those white papers, you're gonna say to yourself, how can I repurpose them? And I'm telling you right now, you give it a human component. Talk about it, interact, bring those articles, bring those things to life. That's what I'm talking about. Go out to industrial talk. Always find out more from the great people in industry. Industrial talk is where it's at. People, be brave, dare greatly hang out with a look. You're going to change the world. Thank you very much. Again, we're going to have another great interview right around the corner.
Transcript
Welcome to the industrial talk podcast with Scott Mackenzie. Scott is a passionate industry professional dedicated to transferring cutting edge industry focused innovations and trends while highlighting the men and women who keep the world moving. So put on your hardhat grab your work boots, and let's go Alright, once
again, thank you very much for joining industrial talk number one place that celebrates you, the industrial heroes all around the world. You know why we celebrate you? Yeah, you got to ask that question. Why we celebrate you here on industrial talk. It's because you are bold. You're brave. You dare greatly. Absolutely. You're solving problems. You're changing lives in a positive way. You're changing communities, of course, you're changing the world. That's why this platform is here for you. It's a celebration. All right. hotseat time. The gentleman's name is a Alok Agarwal. Now he is the CEO and chief was was a chief data scientist at Scry Analytics. That's S C R Y. And this is an important topic. And you know, it is it's not out of reach. We've got to talk about AI, machine learning. And of course, predictive analytics. That's what a look brings to this particular conversation. Let's get cracking. Yeah, yeah, let's get cracking. You ever talk to somebody. And you realize, Wow, I don't know a fraction of what that individual is talking about. Now a Alok does a great job. He, he brings the history of that is around AI machine learning the history which which I'm passionate about, how did we get here, you always got to look at the history. And of course, a look brings all that. But it was just that you're going to enjoy this particular conversation. All right, he just are because he brings the lumber and the truth. And it's just a great conversation. And hopefully we'll do more. All right. So I'm out walking the dog. Because that's what I do every morning. My dog keeps me young. So I'm out walking the dog and always thinking I'm thinking about things. This time, I'm thinking about all of the I guess blogs or white papers or articles that exist out there today. It's AI. It's a lot, right. And in there in all of those are, if you look at him, you stack them all up, right? There's just tremendous solutions, tremendous wisdom, tremendous insights, all buried in there. But they're buried there. They're deep in the bowels of these blogs and white paper. And I was thinking if you're doing and I don't mean to be provocative. Yeah, I do a little bit but let's just say I'm, I have a certain thought about all of these blogs in these white papers. Because I think we're at a point where I know that I'm inundated by just information coming out of that terminal. It's like hitting me right in the face and it's all this other information and I'm just me, I wish I wish I was a computer because I would soak it all up, but I'm not right and it's hard to to consume it. So I think that there has to be a shift because right now I don't think people maybe a few maybe if I'm dedicated to a specific location, I'll read the articles. But I know that for me, I'm being changed for the DNA out right. And I I'm one that likes to be see it be entertained, talk about it. And I think a lot more people are doing that as we speak. So with that said, walking the dog, and I came to the conclusion that, wouldn't it be great to dust off these, these blogs, white papers, whatever, repurpose them into a real human centric conversation, record it, look at it. So the format would be, hey, hey, you wrote this article, you got street cred, man, you've got that thought leadership going on. There's the article, let's talk about it. Let's talk about the key points, it's sort of the cliff note version, but at least it gets to it. And then if that if the listener, because that's easy, right? I don't have to pick anything I have to do is just sort of listen, if I'm the listener, I don't have any friction, I could go back and say, you know, I want to read that article, I want to get that or I want to, you know, whatever action call to action there is. So I just,
I really would like you and others to think about how you can repurpose these wonderful, absolutely brilliant articles, or blogs, or reports, or white papers, and repurpose them into a conversation. And no matter how you do that, that would be great. It'd be great on the industrial talk podcast. But the reality is, if I'm, if the industrial talk is here, specifically to educate, okay, and to collaborate, and to innovate, and all of the things that are happening within industry, that has to be part of it. And and we have to get that message out this, this idea that the article will somebody might might find it or whatever it might be, or, or we'll send it into an email marketing campaign, whatever it might be. We're just inundated. So we've, we industry have to really think about how we repurpose those, those rich content, Insight driven, reports, some blogs, but repurpose them, figure out how to do that. And, and come on the industrial talk podcast, because I'll definitely, that makes me want to read them and makes me want to talk about it makes me want to share as much as I possibly can. Because the listeners and industry as a whole, we got to keep on learning we got to keep on a grove and all right. So I'm at a Alok in California. Again, he's smarter than me, which is a lot, don't be offended by it. But that's not a very hard bar, but you're smarter than me. And what you'll gain for this particular conversation is the fact that you'll see him and his passion and his desire to be able to utilize data in a way that is predictive learning, and all of the stuff that's associated with that, and, and be able to help us in the world of industry in the world of manufacturing in the world of utilities, you name it. Help us do our jobs better. And and locusts is just an absolute delight. Enjoy the conversation with my friend. And now your friend. Oh, look. Alright, look, welcome to industrial talk. How are you
doing today? Great. Thank you for having me.
I am so excited about having this conversation. I mean, you bring a wealth of knowledge. But instead of me telling the listeners the wealth of knowledge, your stat cards impressive out on LinkedIn, give us a little background, a little 411 on who a Alok is.
Science from Johns Hopkins in:yeah, it's a powerful word. I kept screwing it up. But now I know it's Scry
actually unlike the thesis of:I have to interrupt you when you said we had this conversation a couple of days ago. And he's, and I didn't follow up on it when you said you got a couple of motors in your room. Because you got to know where I'm going. I'm just sort of thinking motor. What do you mean by that?
he according to our thesis in:So what industry do you tip at Scry? What industry do you normally focus in on?
So two industries we are very big on one is related to financial services, particularly banks, we're gradually going into insurance also banks. asset managers are our biggest industry so to speak. Second is related to IoT particularly Industrial Supply Chain Management, smart cities, that's all where IoT gets used. In fact, one product is quite, quite horizontal called Malaysia which comes a Latin word for coalition and putting together it's putting together a diverse set of data sets, which is sold we're selling to banks for mortgage operations for small medium business lending for KYC know your customer and ID checks and verification for onboarding, etc. The second one is called consensual again, a Latin word for consensus, often called out concordance or harmony. And that is all related to enterprise apps related to IoT, smart cities, and so on. So those are the two big ones. And then, of course, technology Telecom, ends up using quite a bit of IoT. So we will gradually, we are already selling to a few companies in technology and telecom. But we'll probably sell much more,
are you are you considering the IoT solution, the app in a manufacturing type of environment,
we are already selling it to manufacturing environments. So in particular, one of the very important apps, which people often forget, in IoT world is that the devices or sensors may actually go back to the data coming in itself may not be good. And if the data incoming data is bad, then obviously all the analytics that can be done will be bad. So one of the sub products of consensual is called IoT doctor. And basically it is for the health, checking the health of the sensors and devices, because sometimes the sensors and devices may go bad themselves. For example, unfortunately, somebody lights us cigarette close to a sensor, the temperature will go up, the smoke will go up, carbon dioxide will increase. And if these are some of the things that the sensor is measuring, or the device is measuring, obviously, it will have a spike. Similarly, maybe there is a pipe in a manufacturing plant, which is emitting steam just to let the pressure off every two minutes. Then during that period, the device which is close by could have strange readings, the other part where sensors and devices may not go back, but where they may not be able to communicate effectively with the cellular station is because of weather and so on. So many things caused that. And that's the first thing that you want to make sure is that the data is at least 95%. Correct.
That's interesting, because I never really thought about it. There's been a plethora of of IoT, the conversation, IoT, everything that's going out there splitting the devices out on these assets, all of that fantastic by digital transformation. Got it. But I've never really spoken to anybody that talks about, hey, we, the device eventually could fail,
right? Yep, the device could fail, the battery could go down a single failure as the battery goes down, right? But the device could fail in many, many different ways. I mean, we know all the equipment fails sooner or later. So how do you know it hasn't failed? And it may feel temporarily or common?
And senior? You're compressing that time, right? You might, and that's where the AI solution is to say, Hey, we got a problem. Compressor time somebody goes out, replaces it does whatever it has to do. Yeah. And affect the change quicker.
Which Exactly. And if it's a smart water meter, for example, I mean, San Jose Water Company doesn't have to go out, they can just tell me Hello, can you please do us a favor? This is where the battery goes? Can you please put it in? Because it's good for you? It is good for us. It's good for you. Because if you have a burst or a leak in your pipe, a smart water meter will immediately tell you as an alert. That's what has happening. It's good for us because we're getting data in real time using the cellular device.
Yeah, yeah. And then when we start talking about API's, right, you don't have really any limitations API's allow you to take your apps and be able to plug and play to. And that's
the reason why we call them enterprise apps. I mean, it's very much like, I mean, I would say a smartphone app and iPhone app, but the difference is that these are for enterprise is not for individuals, and you can literally plug in there and and get going in a matter of a day or two with the whole process new process.
And from from my perspective, what are we talking about from an ROI perspective, what what are we are we are we looking at a an expensive solution or something that is economically viable
was the founder of Intel, in:and see what's interesting, what you're talking about is constantly being driven down. Because yeah, as greater adoption happens, right? More people are gonna say I need I need it. And that whole, that whole juggernaut that wants to move forward definitely will continue to drive down the prices,
absolutely. And open source will continue to drive the prices. And I mean, if you look 15 years ahead, not five years, quantum computing will be the next thing which will be after Moore's law because more employees saturating now, but that will begin to drive down the prices, because you can do much more computing using quantum computer. So I think there is a lot positive that's going on, for the humanity worldwide, because suddenly, it's not only us, or Canada, or OECD countries that can use it. I mean, countries like India, China, and China, agencies are still much better. But India and other poorer countries are full time using it.
So that's a beautiful thing alone. That's that's, that's transformative. And that's not out of the reach of small companies, big companies, it doesn't really matter. It's, it is truly transformative. And we better the world as a result of that. And I yeah,
electric powered scooters by:So that's, that's, that's pretty cool. Come on. I mean, listeners, you gotta you gotta sort of sit back and say, Yeah, that's the future is pretty god. Cool. I like it. Now. You had me, it's all cool. The conversation that we're having right now it is really bitchin. Now. What are the what are the what's the big problem? What is that? You know, this is all great. But you know, there's always robots. What is it? Yeah,
they're five hit the wall in:this is so interesting, because I, I remember that all of a sudden had a few years ago that I never I get it, somebody says, hey, just stored in the cloud. And of course, that's a learning curve for me and going, what the heck does that mean? Right stored in the cloud, and then all of a sudden, everything is in the cloud. And then I just, I've just thinking to myself, Who the heck is providing the power for all of this, and it must be just absolutely insane. Because, you know, every time I take a picture, video, cloud, everything's in the cloud.
And that's where it will do a lot of innovation will have to be done. I mean, something some of the good news is that in theoretical computer science and math in physics, some of this has been going on. That's why I'm a very big proponent of basics. theoretical work basic sciences, like how do you call these machines? What do you do? Okay, when you call these machines, you have hot water, then you recently this hot water? How do you get all this energy? Electricity? I mean, wouldn't be surprising if this continues if the world requirement for energy for AI machines will already exceed that us by all air conditioners in the world. So So there we have it. I mean, we'll have an interesting, a cycle where it will hype will hit reality.
Yeah, because you're sitting there, you're pulling on the thread, you go, Hey, this is great. This is great. This is great. And all of a sudden, somebody like that story you told us about that learning, and they just did they made a problem with the code. Yeah, code back. It's like, can you imagine sitting around, it's a darn it. I mean,
honestly, I mean, because we do a lot of research and advanced development in Scry, one of our PhDs he came to me and I said, Hey, he said, Look, we made a small error here. And I said, Why don't you train it? He says, I love really, you want me to train it for this small error? It will take me two and a half weeks. I don't know whether a small error is fixed at this point of time. So this is already happening with us. I mean, imagine you multiply that million times 10 million times and particularly contract I mean cities, giga cities like Neo, and other mega cities coming up with our smart cities, where this is what they will be based on. And you suddenly say, yes, the good news is neum, as a smart city is in a desert, they get sun all the time. But they use that sun very efficiently, and very cheaply or inexpensively to
produce all this electricity. It's true. And then there's the physical characteristics of power. And there's the force, there's, there's things that are just a part of power, that keep and keep a community, like, like, I look at the lights here, and there's just, it's, it's power that's pushing, it keeps it stable. There's a force there, you know, I mean, these are, these are difficult questions.
These are interesting questions. I mean, that's exactly how interesting paradigms get created, how hypes get created, how they hit the wall. And it's no different than what happened with physics, right? I mean, we hit the wall before Newton came around, Newton gave Newtonian mechanics and that continued for a few years for a couple of centuries, hit the wall again, then Einstein came around. So it's all up and down. But the overall curve is, is on the positive and direction. And I gotta
tell you, that you're the first one that really addressed the fact that this is all great. But it requires power, it requires energy to do this, right, whatever this is, it requires, and it's, it's hot. So it requires a lot of power. And as you continue to scale more, and more and more, and and, and so we wrestle with, we want a sustainable future, and use sustainable and renewable type power. And yet we want this innovation to, and it's a balance. I mean, I'm glad you're smart. And I'm not
nothing. I think this happened, the same thing happened in the Industrial Revolution. That time, of course, we were unaware of its effects on climate. But we all realized that it required fossil fuel and energy to run pumps and motors, engines and all that good stuff. I mean, these are the new pumps, motors and engines, which are, I mean, in some sense, you can I say their software, but they still running on the hardware underneath, which still requires energy, which still requires cooling.
Yeah, yeah, you're absolutely. Yeah, I'm gonna tell you, it's like it. You know, you had the Industrial Revolution. And then we had all these talking about all the industrial and now we're at four Dotto, right. And, and it's still it's just it's a, it's a energy centric type of solution. Great thinking, great minds. Gotta have it. Gotta have that energy, some way, shape, or form. Brilliant. Brilliant. We got to wrap this up a look over my time limit.
Thank you so much for having me. I mean, I love to be part of whatever you folks are doing, because you're great. I mean, absolutely. It's exciting
times out there. And you're leading the way. And I and I could geek out on this stuff all day long and talk to people to go, oh, my gosh, that's cool. That's cool. And for me, it's hard to sort of maintain my composure. How do people get a hold of you? Look, what's the best way?
I think email is the best my first name dot last name at scribe linux.com. I would say that is I actually respond much faster and quicker to email than to my whatsapp or text messages.
Yeah, you're pretty. You're good at that. I have to. I have to admit you're very good at that. And that way Scry SC, r y. So you listeners out there ascribe analytics, a lope? How do you say your last name?
Agarwal? A? I mean, if my parents knew how to pronounce these things properly in English, they would have used you rather than me. So it should have been UGR AWS, but I'll look at what
yeah, you gotta reach out to him. Yeah, listeners, you're not going to be disappointed. You got a lot of passion, man. I like it.
Thank you. Alright, let's
do this. We're gonna have to wrap it up on the other side sphere not you're gonna have all the contact information for my friend. Look at anything else that you'd and don't come to me and say, Scott, I can't get ahold of the load because he is responsive. All right. Thank you. Staying Scott really enjoyed it. Joe fires there, my friend. Stay. We'll wrap it up on the other side.
You're listening to the industrial talk Podcast Network.
All right. Once again, thank you very much for joining industrial talk and celebrating industrial heroes and learning from the very best in industry. Thank you to a loake for being on The industrial talk you go reach out to his stat card out on LinkedIn, I'm telling you will not be disappointed. Scry analytics SCR y analytics is the company. Come on facing. You need trusted individuals that know what they're talking about in the world of AI, ml and of course, predictive analytics. Alo, all right, I'm gonna challenge you. You're gonna look at those blogs, you're gonna look at those white papers, you're gonna say to yourself, how can I repurpose them? And I'm telling you right now, you give it a human component. Talk about it, interact, bring those articles, bring those things to life. That's what I'm talking about. Go out to industrial talk. Always find out more from the great people in industry. Industrial talk is where it's at. People, be brave, dare greatly hang out with a look. You're going to change the world. Thank you very much. Again, we're going to have another great interview right around the corner.