Buddy Lee with Mesa Associates
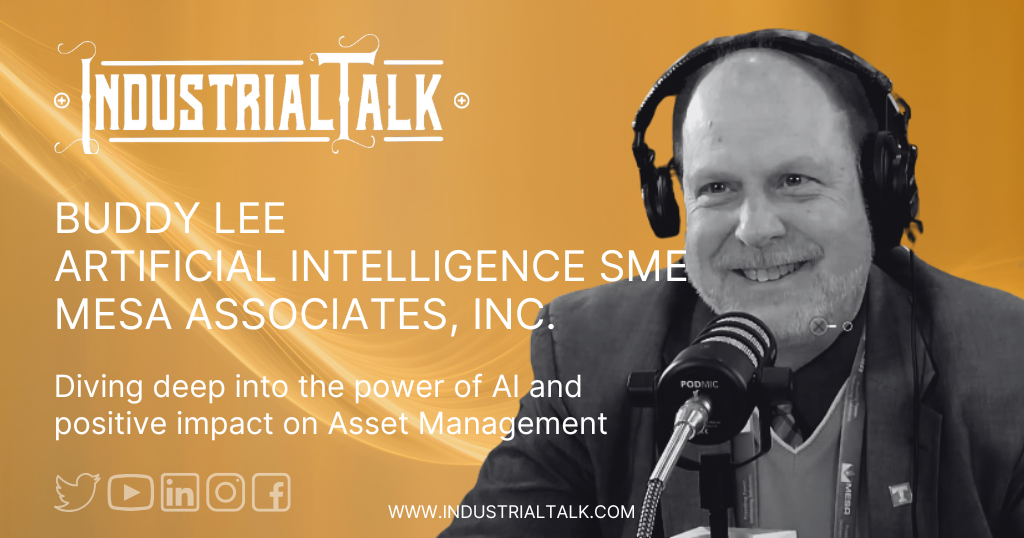
Industrial Talk is onsite at PowerGen 2025 and talking to Buddy Lee, Artificial Intelligence SME at Mesa Associates about “AI and Asset Management“.
Scott MacKenzie hosts an industrial podcast from Power Gen in Dallas, Texas, celebrating industry professionals and discussing innovations. Buddy Lee from Mesa Associates, an AI subject matter expert, shares his background in electrical engineering and reliability engineering. Buddy discusses the application of AI in asset management, particularly in detecting anomalies and predicting failures in industrial machinery. He highlights the importance of having a good data set for AI models, emphasizing the need for accurate and reliable data. Buddy also touches on the cultural challenges of implementing AI in industrial settings and the potential for AI to improve operational efficiency and reduce maintenance costs.
Action Items
- [ ] Reach out to Buddy Lee to discuss further details on Mesa's AI-powered asset management solutions.
- [ ] Explore opportunities to instrument industrial equipment and collect relevant sensor data for AI/ML model development.
- [ ] Assess current maintenance practices and failure modes to determine if an FMEA-based AI approach could provide value.
Outline
Introduction and Welcome to Industrial Talk Podcast
- Scott MacKenzie introduces the Industrial Talk Podcast, emphasizing its focus on industry professionals and their innovations.
- Scott welcomes listeners to the platform, highlighting the importance of industry professionals in solving global problems.
- The podcast is broadcasting from Power Gen in Dallas, Texas, showcasing the latest innovations in the power generation industry.
- Scott mentions the excitement of the event and the opportunities to explore new technologies on the show floor.
Introduction of Buddy Lee and His Background
- Scott introduces Buddy Lee from Mesa Associates, who has previously been a guest on the show.
- Buddy shares his background, mentioning his education at the University of Tennessee and Carson Newman College.
- Buddy discusses his degrees in church music, electrical engineering, MBA, and Masters of Engineering in reliability engineering.
- Scott notes Buddy's enthusiasm for Tennessee and his support for the University of Tennessee's football team.
Discussion on Power Generation and AI in the Industry
- Buddy talks about his positive experience at the Power Gen conference, highlighting the technical information and new equipment available.
- They discuss the advancements in small modular reactors and AI in the hydro industry.
- Scott and Buddy touch on the role of AI in driving power demand, particularly in data centers.
- Buddy explains his role as the AI subject matter expert at Mesa Associates, focusing on industrial applications.
Buddy's Thesis on AI Models and Data Sets
- Buddy shares his thesis on using AI models with limited data sets, specifically vibration analysis from a paper machine.
- He explains the process of comparing AI models to human analysts using a seven-year database of vibration data.
- Buddy highlights the accuracy of five AI models in predicting bearing failures, achieving 95% accuracy.
- Scott and Buddy discuss the differences between large language models and small data sets in AI applications.
AI Applications in Industrial Asset Management
- Buddy describes the use of Failure Modes and Effects Analysis (FMEA) templates in AI models to detect anomalies in industrial assets.
- He explains how AI can predict the efficiency of boilers and identify early signs of failures, such as bearing issues.
- Buddy emphasizes the benefits of early detection in terms of cost, labor, and supply chain management.
- They discuss the importance of having a human in the loop to validate AI predictions and ensure accurate maintenance planning.
Deployment of AI Models in Industrial Plants
- Buddy explains the rapid deployment of AI models, which can take days to a week or two, depending on the data availability.
- He addresses the trust issues and cultural challenges in implementing AI in industrial plants.
- Buddy highlights the importance of vetting data for noise and signal-to-noise ratios to ensure accuracy.
- They discuss the need for historical data and the challenges of obtaining reliable data from various sources.
Pilot Programs and Data Collection
- Buddy talks about the process of selecting a pilot asset for AI deployment, such as a hydro turbine or a specific machine.
- He explains the importance of having a FMEA and a good data set to develop effective AI models.
- Buddy emphasizes the need for continuous training and updating of AI models based on new data.
- They discuss the regional differences in failure mechanisms and the importance of tailoring AI models to specific locations.
Digitalization and Instrumentation in Industrial Plants
- Buddy discusses the ongoing efforts in digitalization and instrumentation in industrial plants, particularly in the steel industry.
- He highlights the importance of securing data and keeping it on-premises to avoid external threats.
- Buddy explains the role of IT and OT teams in managing data and ensuring its integrity.
- They discuss the potential of AI to improve operational efficiency and reduce human errors in industrial plants.
Conclusion and Contact Information
- Scott wraps up the conversation, emphasizing the importance of leveraging AI to manage assets more effectively.
- Buddy provides his contact information, including his email, phone number, and LinkedIn profile.
- Scott encourages listeners to reach out to Buddy for more information on AI applications in the industry.
- They highlight the benefits of attending events like Power Gen to stay updated on the latest innovations and technologies.
If interested in being on the Industrial Talk show, simply contact us and let's have a quick conversation.
Finally, get your exclusive free access to the Industrial Academy and a series on “Why You Need To Podcast” for Greater Success in 2025. All links designed for keeping you current in this rapidly changing Industrial Market. Learn! Grow! Enjoy!
BUDDY LEE'S CONTACT INFORMATION:
Personal LinkedIn: https://www.linkedin.com/in/buddylee/
Company LinkedIn: https://www.linkedin.com/company/mesa-associates-inc/
Company Website: https://www.mesainc.com/
PODCAST VIDEO:
THE STRATEGIC REASON “WHY YOU NEED TO PODCAST”:
OTHER GREAT INDUSTRIAL RESOURCES:
NEOM: https://www.neom.com/en-us
Hexagon: https://hexagon.com/
Arduino: https://www.arduino.cc/
Fictiv: https://www.fictiv.com/
Hitachi Vantara: https://www.hitachivantara.com/en-us/home.html
Industrial Marketing Solutions: https://industrialtalk.com/industrial-marketing/
Industrial Academy: https://industrialtalk.com/industrial-academy/
Industrial Dojo: https://industrialtalk.com/industrial_dojo/
We the 15: https://www.wethe15.org/
YOUR INDUSTRIAL DIGITAL TOOLBOX:
LifterLMS: Get One Month Free for $1 – https://lifterlms.com/
Active Campaign: Active Campaign Link
Social Jukebox: https://www.socialjukebox.com/
Industrial Academy (One Month Free Access And One Free License For Future Industrial Leader):
Business Beatitude the Book
Do you desire a more joy-filled, deeply-enduring sense of accomplishment and success? Live your business the way you want to live with the BUSINESS BEATITUDES…The Bridge connecting sacrifice to success. YOU NEED THE BUSINESS BEATITUDES!
TAP INTO YOUR INDUSTRIAL SOUL, RESERVE YOUR COPY NOW! BE BOLD. BE BRAVE. DARE GREATLY AND CHANGE THE WORLD. GET THE BUSINESS BEATITUDES!
Reserve My Copy and My 25% Discount
Transcript
SUMMARY KEYWORDS
AI in asset management, power generation, Mesa Associates, Buddy Lee, industrial innovation, reliability engineering, data analysis, machine learning, Failure Modes and Effects Analysis (FMEA), anomaly detection, digitalization, operational efficiency, data security, pilot programs.
Scott. Welcome to the industrial talk podcast with Scott. MacKenzie. Scott is a passionate industry professional dedicated to transferring cutting edge industry focused innovations and trends while highlighting the men and women who keep the world moving. So put on your hard hat, grab your work boots and let's go all right
once again. Welcome to industrial talk. Thank you very much for joining this particular platform that celebrates you, industry professionals all around the world. You are bold, brave, you dare greatly, you innovate, you collaborate, you solve problems, you you you are making the world a better place. That's why we celebrate you on the number one industrial related podcast in the universe that's it's all backed up by data, and we are broadcasting from power Gen here in Dallas, Texas. It is a great, great event. It is the last day. It is starting to wind down, but still, there's a lot of opportunities to be able to sort of kick the tires of all this wonderful innovation that is on the floor. It is, if you want to get into a profession, you need to look into anything dealing with power generation. It is a really fantastic market to get involved in. All right, he's been on the show. It was a number of years ago, number of years ago. He just wandered up to my broadcast booth. Buddy Lee. He is with Mesa Associates, and we're going to be talking about everything, nothing. We're going to talk about everything. So let's get cracking. Hi buddy.
Good morning. It's good to see you again. Yeah? It's good to see you, Scott, yeah.
It brings back a lot of good memories. I think we were in Florida when we first connected, and you know, that was, that was a great conversation. Yeah, all to do around reliability, asset management, you know, maintenance and all of the strategies associated with that, sure can't complain. Coffee in hand, coffee in hand. Yes, all good. Ready to Roll early morning. Yeah,
it's a complaint. Let's, open it up for artificial intelligence and asset, man. I mean, to tell you, I love these
topics, yeah, because here's the funny thing, he is, he is the AI subject matter expert, industrial application. It's a mouthful for Mesa associates, but it's a, it's, it's a big title, but he can handle it. So yeah, before we get into that and all of this stuff, because AI is a real, you know, interesting topic, absolutely, give us a little background on who buddy is, sure
llege. Got my first degree in:front door. And by the way, as you can tell, if you're out on the video, you can tell that he's got, he's a big fan of Tennessee because he's got the colors going in the pin hair and
all good. Rocky Top, baby. Rocky Top, yeah, best fat song in the USA. Yes,
it is. I'll go with that. I'll support you on that one. So let's talk. You're having a good conference. Yes, sir. Have you been to this one before?
I have. It's been a number of years since I've been to it. But it's a huge conference. It's an excellent conference. If you want some good technical information, and more or less you want to get in the show on the show floor, particularly, and learn about all the new equipment and technologies that are coming out. This place is huge, and it is a great place to come to if you're in the power industry, which is kind of a stayed industry. It's been around for a while, but boy, we're seeing some really nice new things. And, you know, small modular reactors coming out in the nuke side, we're seeing developments happen. And even, you know, in in hydro, my gosh, there's some nice AI stuff happening in hydro these days. And so it's, it's an exciting time to be back in the power industry, and coming to this conference. I'm thrilled to be here. I
have to, have to sort of go off on a tangent real quick. Is somebody doing a small modular reactor out here? Have you seen it? I haven't seen that. No, I have not either, but I know about it. I mean, it's, we
have them in Oak Ridge just near me, yeah, actually, and that's a big topic of conversation around the DOE and those, those in those areas. And since Mesa, what we do is design electrical things. You know, we are involved in some of the. Those, I think, interconnects and things like that that they're doing. But again, that's not my particular area at Mesa. But you know, it's an exciting time with all this new stuff that's happening in the data centers are driving a lot of that, so AI is actually driving the power demand curve up, and we're seeing some. It's just an exciting time to be back in power. It is,
yeah, it is. So let's, let's talk about AI. We might as well just recognize the elephant in the room right now and talk about, what's your role with Mesa and being the AI. Shmi, yeah.
w many models were you using?:it's still sort of funny, because that's considered a small language model. It's still compared to the large language models, which is just, well, yeah, there's a
little bit of confusion in terms there, yeah, this is a large data set. Yeah, not a large language model, like you train against chat, GPT, yeah. Okay, okay, so those are separate things. Now we do use a small language model in terms of what I'm doing right now with Mesa, in terms of this, what we're doing is we're using Failure Modes and Effects Analysis FMEAs. They're called, sometimes you put criticality analysis on the end of that. It's a famica. But we use those studies that are done out there, and we say, Okay, we got a template, and we kind of know for a particular machine how it's going to fail, and what the effect of that is. And so we can take the data from all the various data sources, very disparate data sources, maybe vibration, temperature, pressure, flow, etc, current, draw. We can take all of that and put it into a system and say, Okay, have the relationships between all of these sensor values changed? And if so, that's an anomaly, and that will give us earlier detection than waiting for a set point to actually fail from, say, a vibration data point. And so we can look at those anomalies, and we can say, Okay, now if this is giving me earlier detection, does it fit within my failure modes and effects, and I can actually ask the system questions, and it's a limited, large language or a small language model against those FMEA templates with all the data inputs that are coming in, all the data against that, and I can say, okay, predict For me the efficiency of a boiler for the next three months. Or I can ask it a question like, What happened when this bearing went out on the thrust bearing? And we found cases where we're seeing anomalies four months prior to a set point failing on a vibration, you know, system, for example. So we're getting earlier detection, which is a good thing from a, I mean, gosh, even from just a supply, supply chain standpoint, much less labor you know, that you got to put in the field to fix the problem. Getting that earlier is just a fantastic thing, because I can plan for it better. I can buy it cheaper. I can do a lot of things that I'm not reacting to a problem in real time, which is absolutely the worst thing. We should call that run to failure maintenance, you know, but that's that's still done appropriately on some equipment. But, you know, in heavy asset industry, like we have in power, we. Really want to do that in advance, and this anomaly detection from the AI engines that are running, and the machine learning, and then just being able to use that like a chat GPT interface to say, okay, predict for me when the next failure is going to happen on my thrust bearing because my oil temperatures are up, okay? And then it will give us an idea, and we can from that, have a human in the loop, so it's a supervised system, but he can say, Okay, I look like I have about three months of remaining life on this before I have to actually do something that set points violated. And so that gives me a lot better idea of how to get all my pieces and parts together to get it fixed.
Are you? Are you taking these models and are they? Is it in, in production, right now? Yes, okay. And are you seeing accuracy there? Like saying three months to failure, you've you see it. It's happening. You, you have the data. Is it accurate? Is it yes,
it's extremely accurate. In fact. I mean, you know, we're seeing, if you want to get out of the realm of industry for a second and see where it could conceivably go, look at what Tesla is doing. Tesla is collecting millions of data points from every single car that they have on the road all the time, and they're trying to react to a much bigger model than we're in. It's huge. So it's huge. And what they're doing in real time is driving a car. Okay? So, yes, it works. It works well. And it works at lightning speeds that a human can't approach, okay? What a human can do is be the fail safe in this if you ever want to put it in production, okay, in an industrial plant, that will be slower for us than driving. Okay, Tesla's pushed that boundary, so it'll be slower for us. And a lot. And frankly, there's a trust problem still. There's still the culture issue with AI, you know, it scares a lot of people. They think Terminator, and, you know, the old movies and all that we're not talking about that. We're talking about machine learning. We're talking about a subset of, you know, the generative AI that everybody knows, chat, GPT and the generative AI. We're really talking about a subset of that called machine learning, where we actually use the data that's been generated by the machine and people out there collecting all kinds of data. We're getting data out to wazoo and it's going into a historian mostly never to be heard from again. But what we can do is we can go back into those historical data banks with the AI and use it to train models and then apply those in real time to what the incoming data is looking like, and we get a much better idea of how, basically, how to operate our machines. Frankly, most of the problems that are out there, and this is very true in the nuclear industry, most of the problems that happen out there are human caused. Yeah, I
was just going to say, once you open that asset up, then you have the potential of introducing another Well, I'm really
speaking, yes, that's very true. I'm really speaking more about operations on the operation side. I mean, look at what happened, at what was the one in Ukraine, the nuclear plant at Chernobyl. Yeah, Chernobyl was caused by somebody going off the rails and doing tests that they weren't supposed to be doing and violating their own procedures and their own I don't know if you saw that. Ever saw that Chernobyl special on HBO, but basically, that was a human caused event. That whole meltdown was caused by a human and so we Fukushima that that wasn't, that was weather related, you can't but, you know, there's a lot of human operations problems that happen out there in industrial plants, and that's an extreme example, you know, at that plant in Ukraine, but it's, it's a very important lesson to learn. You know, as we are operating these machines, they have a specific sweet spot that they like to, you know, they call it best efficiency point, for example, on a pump where they like to be operated, and lot of times they aren't operated near that, and they cavitate, and they cause all kinds of issues, especially as they empty a tank and the suction pressure goes down, they start cavitating. They start eating up the impeller, and before you know it, you've got a pump that's not operating anywhere near its best efficiency point because it's probably oversized in the first place by the engineers that designed it. So you have some design problems that come in, but mostly it's operational problems that happen out there in industrial machinery. And we, you know, I'd like to help clean that up, and I think that's what the promise of AI really helps us do. Operators are fantastic people. This is not to denigrate them at all. It's just in the way we operate, it on day to day, and trying to meet goals where we're trying to get, you know, product out the door and things like that. These market pressures come into play. My gosh, there's just so many things that can go wrong that AI can help us, you know, be the gutter guards in a bowling alley, where it keeps us out of the rails. AI can keep us in the center spot where we hit. Few pins. So
here's the question that everybody asked. Sure, that sounds great. That's all wonderful. How fast? How can I? How fast can I begin to deploy an AI strategy at my facility to be able to manage my assets more effectively? Yeah, and, and
for let me tell you, fast enough to make you uncomfortable. Okay, yeah, I'm talking about we can do a model and deploy it in days. Honestly, if we get the data in now, to get the data in might take a week or two, but to start getting some good ideas of how your machines are operating, what those what those anomalies are that the system can detect is extremely rapid. Some models depends on how complex it is for doing weather patterns. That's a hugely complex model, right? But if you're just talking about a machine and its operation, it's supposed to be at a certain temperature, pressure, flow, you know, certain balance of mass going through a machine. We know where that's supposed to be in most design applications and industrial plants, particularly in power, because we're so regulated. So, you know, I really think that these systems can be brought up fast enough to make people uncomfortable. And that's where we get back to the culture question of, oh my gosh, is this thing taken over for me? And frankly, no, it's another tool in your toolbox is, all this is, but
what do you get the data if I, if I'm, if I'm a new, yeah, new combo company, and you come on in and you just say, All right, let's, let's do it. I I see the benefits. I want to be able to deploy this. Where do you get the data that that you feel confident with?
Yeah, that that can be part of the problem. Okay, now, you just said the important word confidence,
I'm telling you, yeah. So
we do vet the data considerably and ask questions like, Is there too much noise in the data? What's the signal to noise ratio? You know, we ask a lot of questions of the data, and that's probably the first day or two, maybe even longer, just depends on how big the data set is. People are recording this data, they're sending it to a story, and all we do is pull it out, and then we use a lot of statistical tools to tell us is this in the right range for what it should be. If it's not, then was an anomaly detected during that time. And so we do historical look backs at the data to see if there are problems already in it. And that sometimes involves us going to the computerized maintenance management system to say, okay, was a work order issued against this and to say, the fan was balanced, and all of a sudden we're back to good things. We don't want to train on a model that's already got bad data in it,
yeah, because that's always, that's, that's it. It's just when somebody says, hey, I want to be able to collect the data. I want to be able I have data. I have data everywhere. And yeah, and everybody gets down to the point, but of how much confident do I have in that data? How do I scrub that data? If I want to scrub that, how far do I go back to scrub that data? Yeah, and from what I understand, buddy, we're talking about, there's not a need to go way back, right? You can begin starting to
if you, on Earth look, if you've got six months of data, that's good. That's approaching the right number we need. If you've got two years, we'd prefer it okay, if you got a couple of years of data, if going back much further than that, you're really into the unknowns. People haven't documented things that well, going back much more than five years, you know. I mean, they have data, but the documentation may not be as good. Some people have data going back to late 90s, you know, and stuff like that that they have in their pie systems, their plant information systems, and other, you know, historians. But you know, I think if we can get, you know, 12 to 24, months of data, we can do a real fine job in getting a model put together in a very short period of time. Now, is that a deployable model? Maybe, maybe not. We may need to fine tune that model. It may take some extra time, but is it good for a pilot program to get, you know, a win in quickly for you so that you can expand the program. Yes, we can do that sort of thing all day long, twice on Sunday. Speaking
of pilot, if you look at a particular organization, a business, power generation, whatever, whatever you're looking at, do you take a pilot and you just say, Hey, what's your, which? What's your one of your most important assets? Let's, let's drive a pilot here.
How we do it all the time? Yeah, we actually look at a particular asset. Say it's a hydro turbine, yeah? Well, we might look at the vibration sensors. Maybe they have a Bentley system on it, or something like that. We'll get some vibration data out of that. We may have an iris system giving us the air gap, you know, reading, we may have oil temperatures, we may have a bunch of different stuff, and we may have to run it through several models to see what the parameters are that we can draw out that give us good insight into the developing problems. If we can get your FMEA, if you got one now, we can develop one too. You. Okay, but if we can get that, if you've got it already, and we can get a couple years of data, then the deployments go extremely rapidly, and I think it could be very useful for people. And of course, you always trained and retrained and retrained and retrained. You know, you're always updating your
Yeah, because you're always getting more data and more information. And I would imagine, here's a GE motor here, down here in Dallas, versus a GE motor, the same GE motor, but it's up in Maine, and you're just able to really continue to sort of hone Yeah, and bring that data together. Now you're
gonna do it, yeah, and you're absolutely correct. Now you're into seasonality data, you know. And there's, you know, down here on the on the Gulf Coast, you're going to suffer a lot more corrosion than you are up in, you know, Maine, and so this is where your sneeze come in. Your subject matter. Experts that are in the plant have to know what the predominant failure mechanisms are, and what they've done for their FMEAs and such. And if they've got that data, then, you know, we can do a real effective job of giving them another tool in their tool belt that helps them understand what's going on inside the machine in their region. And I think that's really important to do that, because you don't want to train a model in Maine for something you're going to run in Houston. No, you
make any sense? Yeah, yeah. But, but that that information is all feeds into that model, right? That gives you just a greater insights into how to manage that asset, right,
right? So we're seeing the OT guys, the operations technology guys, and the IT guys, they're they're basically instrumenting plants across now, there's still a lot of work to do and instrumentation, of putting the sensors out there and that sort of thing. And that brings up the whole concept of digitalization. Seeing a ton of work going on in that in the steel plants that are out there, not related to power what we're doing here, but I'm seeing a ton of work there. I really want to, if I can, in any way, influence the folks in power to copy the model that the steel the steel industry is doing in terms of getting their equipment instrumented. We're seeing some of that in some of the hydro plants out in the northwest. They're doing a lot of instrumentation right now, spending a fair amount of money on that, and they're going to be getting much more data in than they've ever got before. So this is getting bigger now, the IT guys and the OT guys have a little bit different perspectives on what to do with that data, but they still have to get the same data kind of in. And the idea here is that they will have security concerns, particularly in the power generation industry. They don't want somebody shutting down a turbine, you know, external, bad actor, right? So there's a lot of that going on, and you will probably have this particular industry looking at doing everything on premise, as far as their data. So that means we're going to push the computing, yeah, we're going to push the computing down to the edge of the machine, and then we're going to get the parameters out that give us the story, and we're going to pop that up and populate it into a dashboard somewhere on some operator's desk. And that's all going to be internal to the specific, say it's TVA, you know, Tennessee Valley Authority, or somebody like that, or Duke, you know, you're going to have all of that stay within their air gapped systems that don't go out to the internet and don't do anything, which makes a lot of sense from a security point of view. So all of the AI vendors, including us, are aware of the issues that are happening there. And so the IT guys are influencing the OT guys, and they're putting their SCADA systems out there, their DCS systems, individual sensors running all that data in and into their internal historians and all that's going to stay on prem. And so a good AI vendor was going to understand all that and help you keep it on prem. They there's very few that I think will actually stick it in the cloud because way, yeah, yeah, yeah. That's a whole other conversation. That's a whole nother conversation. Yeah, not one. I really, you know, I can get into it today if you want to, but it's kind of external to this particular conference. Well,
we got to wrap it up, just because sure has been chirping really well. I mean, this has been a real interesting conversation. How do people get a hold of you? They want to know more about this whole AI stuff?
-:that's always the easiest way, yeah, because it's easy for me to just put a link on my website, yeah. Which brings me to the point. Thank you very much, buddy for popping in. It was great to see you. Yes, you're in, man. Great to see you. Scott, excellent. All right. Right? We're gonna have all the contact information for Buddy out on industrial talk, So fear not reach out to him. AI is happening, whether you like it or not, figure out how you can leverage this tool to help you manage your assets more effectively. It's it's there, it's happening. But you need, you need a Sherpa like buddy to help you along with that journey. So connect with him out on LinkedIn. All right. We're broadcasting from power Gen, Dallas, Texas. Is the location. And again, you get incredible professionals like buddy, if you didn't, if you didn't attend at this time around, make it a point to attend it and get into the power industry. It is a fabulous interesting All right, we're going to wrap it up on the other side. Stay tuned. We will be right back.
You're listening to the industrial talk Podcast Network.
Two letters a and I. It is going to impact you in some way, shape or form. It don't you can't be afraid. That's Buddy Lee. You need individuals like Buddy Lee to help you along with that journey. Yeah, it's, it's it's here to stay, whether you like it or not. You can have positions like, I really just want to have that human component, whatever. But if you're in the world of trying to collect data, analyze that data, refine that data, gain insights into that data. That's a whole AI value proposition that is a must. So learn it, figure it out, get your team on it, talk to us. We'll help you along too as well. And speaking of which, industrial talk is here for you. You need to amplify that message. You need to market more. You need to create that attention that is necessary for you to succeed. Go out to industrial talk. Connect with our team, and you will not be disappointed. Be bold, be brave. Dear greatly. Hang out with Buddy. Change the world. We're gonna have another great conversation shortly. So stay tuned.