Chuck Byers with OMG
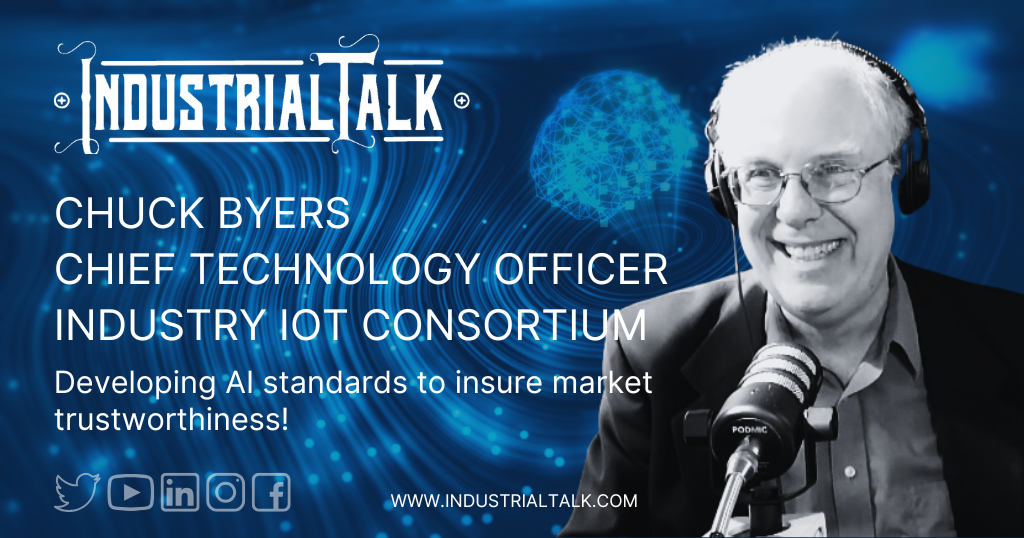
Industrial Talk is onsite at OMG, Q1 Meeting and talking to Chuck Byers, CTO with Industry IoT Consortium about “Developing AI standards to insure market trustworthiness!”
Chuck Byers, a seasoned industry professional, discussed the integration of AI and IoT technologies in the context of the Object Management Group (OMG) and the Digital Twin Consortium. Byers highlighted the importance of AI in real-time systems, emphasizing the need for prompt engineering and meta AI to ensure trustworthiness. He detailed the computational requirements of training large language models, noting that retraining the ChatGPT 3 model would require 195 NVIDIA DGX servers, costing $80 million and consuming 2.5 million watts. Byers also stressed the importance of sustainable energy sources and efficient cooling solutions for future data centers.
Action Items
- [ ] Explore ways to detect when AI results are not as trustworthy as they need to be, and develop methods to validate AI outputs
- [ ] Investigate the use of small modular nuclear reactors to power data centers for AI model training
- [ ] Promote the work of OMG and the Digital Twin Consortium in addressing the challenges of AI and the Internet of Things
Outline
Introduction and Welcome
- Scott MacKenzie introduces the podcast and its focus on industry innovations and trends.
- Scott welcomes listeners and mentions the broadcasting location at the OMG SQ1 meeting in Reston, Virginia.
- Scott introduces Chuck Byers, highlighting his extensive background in electrical engineering and his contributions to Bell Labs and Cisco.
- Chuck Byers joins the conversation, expressing his pleasure in being invited and his admiration for Scott's hosting style.
Chuck Byers' Background and Contributions
- Chuck Byers shares his educational background, including a Master's degree in electrical engineering and teaching at the University of Wisconsin.
- He discusses his work at Bell Labs and his invention of a US patent for a device that alerts users to approaching badness.
- Chuck talks about his tenure at Cisco, where he worked on computer control, IoT, edge computing, and drone technology.
- He mentions his current role as Chief Technical Officer of the Industry IoT Consortium and its recent integration into the Digital Twin Consortium.
Digital Twin Consortium and Industry IoT Consortium
- Chuck explains the Digital Twin Consortium's focus on pairing simulation models with real-time physical systems.
- He describes the Industry IoT Consortium's focus on sensors, actuators, and the computation necessary for IoT systems.
- Chuck highlights the synergy between the two consortiums, emphasizing their combined potential to sense, network, and actuate the physical world into the digital world.
- He provides an example of using simulation models to optimize oil refinery processes without physical experimentation.
Challenges and Opportunities in AI
- Scott MacKenzie and Chuck Byers discuss the challenges of AI, particularly the lack of standards and trustworthiness.
- Chuck explains the different types of AI, including machine vision and generative AI, and the unique challenges of generative AI.
- He describes the use of large language models in generative AI and their applications in tools like ChatGPT and Microsoft 365.
- Chuck emphasizes the importance of prompt engineering and fact-checking to ensure the accuracy of AI-generated outputs.
Meta AI and AI Trustworthiness
- Chuck introduces the concept of meta AI, which involves using multiple independent AI systems to cross-validate results.
- He explains how meta AI can help filter out biases and hallucinations by comparing results from different AI models.
- Scott mentions a platform called h2o.ai that queries multiple AI engines and displays results, though it still requires human oversight.
- Chuck discusses the potential of automating this process to achieve real-time, trustworthy AI results.
Computational Power and Energy Efficiency
- Chuck delves into the computational power required to train generative AI models, citing the example of the ChatGPT 3.5 model.
- He explains the role of GPUs in handling the massive computational requirements and the efficiency of NVIDIA's GPGPU technology.
- Chuck calculates the cost and energy consumption of training a generative AI model using NVIDIA DGX servers.
- He highlights the importance of sustainable energy sources and efficient cooling solutions for data centers.
Future of Data Centers and AI
- Chuck outlines the future of data centers, including the use of renewable energy, small modular reactors, and immersion cooling.
- He discusses the compact, fiber-optic interconnected data centers of the future and their potential cost.
- Chuck emphasizes the need for efficient energy use and cooling solutions to support the growing demand for AI computational power.
- He predicts that these advancements will make data centers more sustainable and cost-effective.
Conclusion and Contact Information
- Scott MacKenzie expresses his hope for the success of AI and the role of organizations like OMG in defining standards.
- Chuck Byers reiterates the importance of trustworthiness, sustainability, and affordability in AI development.
- Scott invites listeners to engage with OMG and contact Chuck for more information on AI and the consortium.
- The conversation concludes with Scott encouraging listeners to educate themselves and get involved in the industry.
If interested in being on the Industrial Talk show, simply contact us and let's have a quick conversation.
Finally, get your exclusive free access to the Industrial Academy and a series on “Marketing Process Course” for Greater Success in 2024. All links designed for keeping you current in this rapidly changing Industrial Market. Learn! Grow! Enjoy!
CHUCK BYERS' CONTACT INFORMATION:
Personal LinkedIn: https://www.linkedin.com/in/charlesbyers/
Company LinkedIn: https://www.linkedin.com/company/industry-iot-consortium/posts/?feedView=all
Company Website: https://www.omg.org/
PODCAST VIDEO:
OTHER GREAT INDUSTRIAL RESOURCES:
NEOM: https://www.neom.com/en-us
Hexagon: https://hexagon.com/
Siemens: https://www.siemens.com/global/en.html
Palo Alto Networks: https://www.paloaltonetworks.com/ot-security-tco
Palo Alto Networks Report HERE.
Hitachi Digital Services: https://hitachids.com/
CAP Logistics: https://www.caplogistics.com/
Industrial Marketing Solutions: https://industrialtalk.com/industrial-marketing/
Industrial Academy: https://industrialtalk.com/industrial-academy/
Industrial Dojo: https://industrialtalk.com/industrial_dojo/
We the 15: https://www.wethe15.org/
YOUR INDUSTRIAL DIGITAL TOOLBOX:
LifterLMS: Get One Month Free for $1 – https://lifterlms.com/
Active Campaign: Active Campaign Link
Social Jukebox: https://www.socialjukebox.com/
Industrial Academy (One Month Free Access And One Free License For Future Industrial Leader):
Business Beatitude the Book
Do you desire a more joy-filled, deeply-enduring sense of accomplishment and success? Live your business the way you want to live with the BUSINESS BEATITUDES…The Bridge connecting sacrifice to success. YOU NEED THE BUSINESS BEATITUDES!
TAP INTO YOUR INDUSTRIAL SOUL, RESERVE YOUR COPY NOW! BE BOLD. BE BRAVE. DARE GREATLY AND CHANGE THE WORLD. GET THE BUSINESS BEATITUDES!
Reserve My Copy and My 25% Discount
Transcript
SUMMARY KEYWORDS
AI innovation, digital twins, IoT Consortium, generative AI, prompt engineering, meta AI, computational power, GPU servers, immersion cooling, renewable energy, data centers, real-time systems, OMG standards, industry engagement, technological advancements
Welcome to the industrial talk podcast with Scott. Mackenzie. Scott is a passionate industry professional dedicated to transferring cutting edge industry focused innovations and trends while highlighting the men and women who keep the world moving. So put on your hard hat, grab your work boots and let's go all right,
once again. Welcome to industrial talk. Yes, I unmuted my mic this time around so everybody can hear me. And we are operating, we are broadcasting on site. OMG. This is q1 we're in Reston, Virginia, and it is, again, a collection of people who are truly they're givers. They're givers of solutions, and they really are doing what what we take for granted. So that's what OMG is all about. Find out more, go out to omg.org seeing how you can get engaged. All right, he's been on the hot seat before. He's in the hot seat again. Chuck is in the house. We're going to be talking about AI and a lot of other things. So let's get cracking there. It's it sounds better when the mic works. You know yours work. How are you doing?
That's great. And thanks again for having me. Scott. Was a genuine pleasure talking to you. You're easy to talk to, and I think that the listeners get an excellent chuckle out of all the things we talk about.
There's an action shot coming up there. You gotta give it to you. So before we get into this conversation, because you have a you're a wealth of knowledge, I always want to just curl up by the Fireside and just listen to what you have to say. But nonetheless, give us a little background, just a little bit about who Chuck is,
sure, well, Master's degree in electrical engineering, I taught the computer control and instrumentation class at the University of Wisconsin. I was a Bell Labs Fellow at El Catal Lucent, where I invented some stuff that's probably in your pocket right now. See,
I just don't know.
It's hard to say, yeah, oh, my favorite invention, little digression is, is the thing that that street says that everybody, when there's a tornado coming, that they Shut the front door, yeah, based on, I have a US patent on a thing that figures out where you are and alerts you to approaching badness. So that's, I'm not sure that Alcatel Lucent ever made a whole lot of royalties off of that. It's not the kind of thing you'd enforce, right? But I guess I feel like I've saved somebody's bacon somewhere along the line gone. Yeah, so cool. So when you're sitting in one of those, you know, meetings conference rooms, and everybody's phone goes off at once, and everybody dives for the shelter. That's my pet. See, so anyway, I did that stuff. I worked at Cisco for 10 years on computer control instrumentation stuff, as well as lots of Internet of Things and edge computing stuff and a few drone things. And lately, I've been the chief technical officer of the industry IoT Consortium, which is one of the OMG programs that just recently, in the last couple of months, has been integrated into the digital twin Consortium, and that makes a lot of sense. The digital twin consortium is really about pairing simulation models in real time with physical systems and then using one to sort of snowball and predict the other. The industry IoT consortium is really about sensors and actuators, the plumbing and the sensors and all of the computation necessary to take a look at those physical systems and and the union of those two consortium really represents a very powerful combination of having all of the technology to sense and Network and actuate the physical world into the digital world, and then all the simulation stuff that uses that sensing and actuation to sort of run time out into the future and predict, and if I tweak that oil refinery a little bit, is my yield going to be better? You don't want to do the experiment on the oil refinery, but if you got all the sensors in the oil refinery telling you what the parameters are, then you run a bunch of simulations of the physical processes in that refinery, you come back with one good answer that says, Turn that pressure up 14% you'll get a better yield. That sort of thing is the sort of thing that digital twins, plus the Internet of Things, is going to enable us to really augment and really help. See,
I don't know how you cannot, if you're listening out there, how you cannot be involved or engaged in an organization like OMG, because I just think that there's a velocity that exists out there within all of these systems, and the conversations are constantly I think it's always a challenge for OMG and the staff of OMG and the people of OMG to try to wrangle these cats in a way that you produce something that is so meaningful and helpful?
Yeah, we've got an excellent track record of doing things that I think are really important to society. And as the planet gets more digitized, the stuff that we do. Two is the fundamental nervous system of that digitization,
one of the things that has been, and I've been to a number of conferences, and a broadcast from a number of conferences, and it you cannot avoid the conversation that involves AI and then, because it's such a it's a wild west out there when it comes to this AI stuff, nobody really knows what it they might have an idea, but it's still just, it's a wild west. How do you begin to sort of wrangle that bad boy in and and strive to create some standards trustworthiness around all of that?
Yeah, that's
because I don't
that is an ongoing challenge, for sure. And the first thing you got to realize is there's lots of different flavors and degrees of AI. Machine Vision is one that's not that hard to wrangle. I mean, you're looking at a bunch of pixels and you're pulling features out of them and saying, Yeah, that looks like a pickup truck bashing through my front gate or not. You know, that sort of thing is one type of AI, and it's deployed everywhere, and it's hugely useful and occasionally problematic. But the thing that I think has got the world crazy these days is what's called generative AI using a technology called large language models, and that's basically trying to figure out what's new, what it basically does is it takes the whole internet and it kind of digests it into hundreds of billions of parameters, and then it applies them to a technical thing called a neural network, which basically you give it an input, like, write me a term paper on the Russian Revolution. And what it basically does is it uses these billions, hundreds of billions of parameters to figure out what the first word ought to be, and then figure out what all the words after it follows. And if you go to chat GTP, or you go to the Microsoft 365, their co pilot stuff, you go to those and that's basically what they're doing, is they have a pre trained large language model. That's what GPT means, generative, pre trained transformer. That's what that acronym stands for. And basically, what you basically do is is you figure out what the next thing ought to be, string a bunch of those together, and you end up with an answer to that term paper problem. Or, how do I write a Python script to do XYZ, or or, How do I make a picture to represent a certain thing for my my next PowerPoint presentation, I've been experimenting with GTP for, oh geez. Over a year, it came out in November. It was like
a light switch. It was there. All of a sudden. It's like, oh yeah, there it is. Boom.
And I, and I've, I've tried some things that it's like no way in heck it's going to be able to do anything about this. And it just knocked it out of the park. And then I asked it other things that ought to be simple, and it just Bumbles stupidly, right? Yeah. And, and the problem is, is when it Bumbles stupidly, it does so with exactly the same authority and fervor as it did when it gave you the best result you could have possibly imagined. So knowing the difference between those two really has to do with a couple of parameters. One is, is the so called prompt engineering? Do you ask it the right question in a very deliberate, very precise way, so that it won't go off any of 10,000 different tangents that it could have gone off because you didn't rein it in correctly. That's an important thing. And then the second thing is, is, can you independently fact check it? Does, it does the result of the generative AI pass the sniff test. And after a while, you kind of know what a bias or hallucination result starts to look like. And then you basically do you know, kind of like what all those political pundits have been doing all along is you go off and you fact check the thing, did it hallucinate that? Or is there some independent validation on the internet or elsewhere that the result sort of seems reasonable, and when we start to hook generative AI onto the Internet of Things, mission critical and life critical systems, so generative, AI, for example, is starting to control my reactor, my locomotive, my implantable insulin pump, those kinds of things, that's the place where a hallucination or a bias starts to kill people. And we could argue that those problems already exist in self driving cars and all kinds of other systems. But as we start, as we start putting more and more of the Internet of Things directly into this digital twin model, where there's a sensor and a network and generative AI and a network and an actuator and a closed loop where there's no humans doing any fact checking, that's the place where we're potentially starting to look at challenges, and we've got some ideas in a newly formed AI task group across all of the OMG consortiums on ways that we might be able to at least detect when something isn't as trustworthy as it needs to be, and ideally
do that that I don't understand because it. Yeah, you know, we've all, everybody's used it in and you're absolutely right. The prompt is really the the part where you start, then I'm always, it's sort of weird, because here's the thing, I'll watch somebody else do it, and I'll, and I'll, I will be very keen on their prompt to see how they're writing.
There are, there are college courses on prompt engineering. Yeah, they see, there it is. Yes. So to answer your question is, how do you how do you rein it in? How do you make sure that that a typical system is behaving itself correctly? There's one answer to that, and the answer is almost as ugly as the problem, and that is what I sometimes call a meta AI, where you have, let's say that I have a really important result that I want to use AI to get that result, and I'm going to apply that result to a million dollar problem, life critical problem. What I might do is ask three or four independent AI systems, the open AI, the facehugger, the Microsoft one, the Google one, ask them all to generate an answer, but using the same prompt, using exactly the same prompt, but a different training corpus, right? Because, because open AI is not training the same stuff that Google is. And what I can then do is use a meta AI, an engine that takes the results from those four AIS and tries to vote on them in some way, to let off the things that don't seem like they're consistent across the multiple AIS and amplify the things that are really similar across those AIs. So the idea is that the bias and hallucination is pre filtered, because unless the result happens multiple times across multiple AIS, it might not be trustworthy. But if it happens in three of those four AIS sort of the same way, giving you a result that's pointing you towards the same direction, then you can probably have confidence that there wasn't a bias or hallucination that happened in the composite answer. So that would be one way that you might be able to do that. There's
a there's a, a platform, I think it's called h2, o.ai, and that that product does the same thing. It sort of just queries multiple engines, or, you know, whatever, and and then sort of displays it in such a way. It doesn't do the it doesn't say, Oh, yeah. Everybody's sort of leaning you still have to do that with your own eyes, yes, but it doesn't, but it does pull up. It's like, Okay, this one gave a lot. This one didn't give as much. When I don't know why you know that type of thing, right?
And that's a that's a step in that direction, yeah, but that still has a human in the loop, and the human themselves could be biased or, you know, untrustworthy for some reason, yeah? So, so the the panacea of all this stuff is to try to figure out a way to automate it so that I can get those results in less than a second and turn around. That's the key, the tuning of the real time systems. That's one of the things that the Object Management Group is particularly strong on, is all these real time systems, systems that are able to do things with sensors, closing the loop of computation and doing an actuation before the results that are that are causing that actuation become too stale to be useful in the physical system.
You cannot have this conversation, this computation conversation, as we you know, like you said, you know, chat, GPT, year in its life, maybe a little longer. Who knows? But it's, it's, it's exploded, yes, and so there's this computation reality that if we don't, if we don't address that in some way, meaningful way, then all of this is just sort of
take us through that. Where are the compute cycles coming from? Yeah, is it coming from an Amazon Web Services server room somewhere and and the answer is, the servers that we've been using in the Big Five cloud service providers, Google, Amazon, Facebook, slash meta, Apple and AWS, Microsoft, excuse me, those are they all sort of rack up these servers, 40 standard computers using Intel Core i nine kind of processors. They rack those up, and then they put in 10,000 of those racks into an acre data center, and then they pour 50 megawatts of electrical power into it, and they can do a pretty decent job at, you know, for example, e commerce, or trying to, you know, maybe serve up a Netflix program for you, something like that. But if you're trying to build a generative AI model, I've got some statistics on the chat. GTP, 3.5 model, the one that open AI, debuted in November of 23 or 22 excuse me, and is the one that sort of kick started all this. It took 3.1 times 10 to the 23rd floating point operations to train that model. And that's basically a measure of ridiculously large computing power, and what you need to do is to build an infrastructure. And that took them $48 million I think was the quote, and about nine months worth of computation. I think they used the Microsoft servers for that. That's a thing that is not sustainable, because it gets ugly fast. Yeah. So what you have to do is get on to a better computing platform, and that today tends to be graphics processing units, the same GPUs that you see, similar to the ones in your Playstation or your Xbox 360 or in the special Accelerator card that you put into your laptop PC because you want to play games, or maybe you've got a more sophisticated application, like you want to render videos. Those are what you use GPUs for. Turns out that NVIDIA in particular has, they've pioneered a thing called GPGPU, General Purpose Graphics Processing Units, where the GPUs have some special architectural tweaks to them, so that not they're pretty good at managing images and rendering textures and all the things that GPUs normally do, but they're also really good at doing a whole bunch of multiply and accumulate operations, you know, trillions of those operations in order to start to whittle down on that three times 10 to the 23rd operations that are necessary to chain to train the GPT three model. And I did some some calculations, but I can share with you about yes please, if you It turns out that it's important to train that model kind of quickly, because, well, I can give you an example of why I asked chat GTP to write me two page paper on the dangers of Chinese balloons, and it wrote me this really excellent paper about heavy metal poisoning and choking hazards for babies and wildlife problems, but it didn't know anything about balloons floating over Lake Erie. That's right, because it was trained before that. That's right. So the the staler you allow your training corpus to get, the lousier the results of these things will be in the more danger you'll have of missing something really important in the in that trained model. So I said sort arbitrarily, well, let's retrain those three times 10 to the 23rd operations in a week. Let's, let's figure out how much computation power is necessary to do that in a week. And I can use a particular type of server, which is called an NVIDIA DGX, h, h1, 100 server, and it's got eight of these big old GPUs with about 15,000 processor cores in each of them, 150,000 some processor cores. So it's two or three orders of magnitude fatter than the the one you Intel servers. And let's just rack a bunch of those up and keep going. Make some assumptions about how many of them you need to train the GTP three model in a week. And the answer is 195 of those servers. No kidding, yes. So that's and there's a lot of footnotes on that about efficiency, yeah. And it turns out that one of the problems with those servers is they all kind of want to talk to each other. All of the GPUs in that architecture, eight per server times 195 they need to talk to each other because they get to a place where they've calculated all they can with the memory that they've got in that GPU, and then they got to reach out to an adjacent GPU and fetch other stuff to finish the calculation. And in the meantime, the first GPU is stalled, waiting for the return of that so if there's takes too much time to get that result back, then your system is lie. Is likely to be less efficient. So you make assumptions about that, put it all all in place. What do you think 195 of those servers cost? Right? They can't. It's about $80 million and it uses around two and a half million watts of electrical power, which is the equivalent of 10,000 houses or something like that. It's ugly, fast, but the model that it creates is something that is really, really useful, because it's doing the basis for a very modern, very current, high performing generative AI system. Then once you compute the model, you spit it out to a bunch of perhaps less costly servers to do what's called the inference. That's where you give it the input from the user, the question from the locomotive, and it crunches on all those parameters in the model, and then in 20 or 30 seconds, it spits you back the answer, if you if you have a really fast set of GPUs like the one I was describing, instead of taking. 30 seconds for it to write that term paper. For you, maybe it would take one second to write that term paper, and that starts to become really useful in these real time Internet of Things situations.
Yeah, but the power that it's still it's still difficult, just that, that scenario that you provided, still, listen, we're not, we've just scratched the surface. Yeah,
where does the energy come from here? How do you cool it? Those are all really interesting,
just because it's going to continue to proliferate, and they're going to create more use cases to, hey, let's use it here. Let's do it here in this manufacturing process, you know, and it's all it's all necessary.
It's up to engineers like me to make it as efficient as possible. And the ways you make it efficient is you figure out a way to get the energy from a place that isn't going to provide a lot of carbon pollution, like renewables, generally wind or photovoltaic solar. There's a new technology called small modular reactors, back to nuclear, and those are really very promising, a 50 megawatt electrical, smart Modular Reactor. It's about the size of a mobile home, and you can sort of bury it in this little bunker out behind your data center. It's pretty down, gone, yeah, that's pretty good. That would run quite a few of these model building engines, and what energy you don't use there, you just send to the truck stop across the street to charge all those electric semis that are going to be sitting around wondering where their energy is coming from. So there's a lot, a lot to be said about that. Then you got to figure out how to cool it. And it turns out that those GPUs want to all be sort of tightly coupled, tightly packed in with each other, because the amount of time it takes for the electrons to go out a couple of meters of cable will screw up that inner process communication bandwidth. So if you squish more and more of them into a smaller and smaller package, you get to the point rapidly where you can't blow enough air through it to keep the thing from melting. So that means you got to take that whole server and dip it in dielectric fluid immersion cooling. It's called, and that's a very important trend in the next generation data centers. They're going to not use hurricanes full of air, where you have to have headphones on when you go into those data centers, because OSHA says that the sound level is too loud from all those fans these things, well, they're 70 decibels. They're basically about as loud as an aquarium pump, and they can cool, you know, a tub, maybe the size of half of a bathtub that you can cool 300,000 watts into a thing that size, using immersion cooling. It's, it's, it's full of lots of interesting technological innovation yet to be done, but it is my opinion that it will likely be the data center of the second half of the decade. It will have certain types of very of distributed power distribution on the thing. It will have next generation GPUs, Nvidia, AMD and a few other companies are working on those already. It'll have, it'll be full immersion cooled. It'll have lots of fiber optic interconnect. It'll be physically compact, and it'll cost 10s of millions of dollars per each of those tanks. You need quite a few of them in your data center. So, so that's what the human race is. Collective brain is likely to look like at the end of the decade, and it's somewhat different, wow, mechanically than today's data center, but really what it is is a bunch of processors that know how to plunk digital numbers out of memory, do things to them, put them back into memory, and repeat trillions of times per second. That's what it's about, and that's what AI is going to need in abundance.
Well, I'm glad you're thinking through this, because I I see it and it gives me hope, because we have to succeed. We have to succeed at it. So delivery of a solution, but it requires organizations like OMG and to to help sort of define that, to create that those standards so I can trust it, so I can I feel good about it, yep,
yep. We want it to be trustworthy. We want to be sustainable. We want it to be reasonably affordable. We want to be future proof. We want there to be lots of capabilities that lots of people will find interesting for lots of different sets of applications. And I think, I think that OMG, is in a position to work on all of those fronts easily.
You come, you come to this event, man, my goodness, yeah, you got yourself a lot of a lot of intellectual horsepower roaming around here. Excellent, yeah. How do people get a hold of you? Chuck, well, you
can certainly find me at buyers, b, y, E, R, s@omg.org, and I'd be happy to help you if you're interested in joining the consortium or talking more about AI and where the future is heading. Excellent. Thank you. Scott, Yeah, appreciate it. All
right, we're gonna have all the contact info. Information for chuck out on industrial talk. You got to check out his other conversations he's had on industrial talk. He's every bit as impressive back then too. He's he's learned a lot in that short period of time. I'm always amazed at how the conversation just changes so drastically, just so so drastically.
It's the way technology is moving. Man, nothing standing still. And we are really in we're in the golden age. We are,
and I'm an old guy now. I don't like it all right. We're broadcasting from OMG S q1 meeting here in western Virginia. You got to get engaged. Go out to omg.org find out more. We're going to be right back.
You're listening to the industrial talk Podcast Network. You
feel another, another incredible conversation delivered to you by Chuck. It is an exciting time in technology and innovation. It is you just, you just need to educate as much as you possibly can. Listen to chuck go out to industrial talk, find all his conversations, because you're gonna be better off. Always wonderful. OMG. That was the OMG quarterly meeting. And again, I can't stress them out, the amount of individuals that just go to that event and just truly would like to help. It's a great event, great organization. Find out more. All right, we're building the platform, platform dedicated to you industrial professionals. You have a podcast, you have technology, you need to put it out on in industrial talk. Let's get some traction for you. Let's do that all right, I say it all the time. Be bold. Be brave. Dare greatly. Hang out with people like Chuck and you will be changing the world. We're going to have another great conversation shortly. Stay tuned.