Prasad Naik with Saviant Consulting
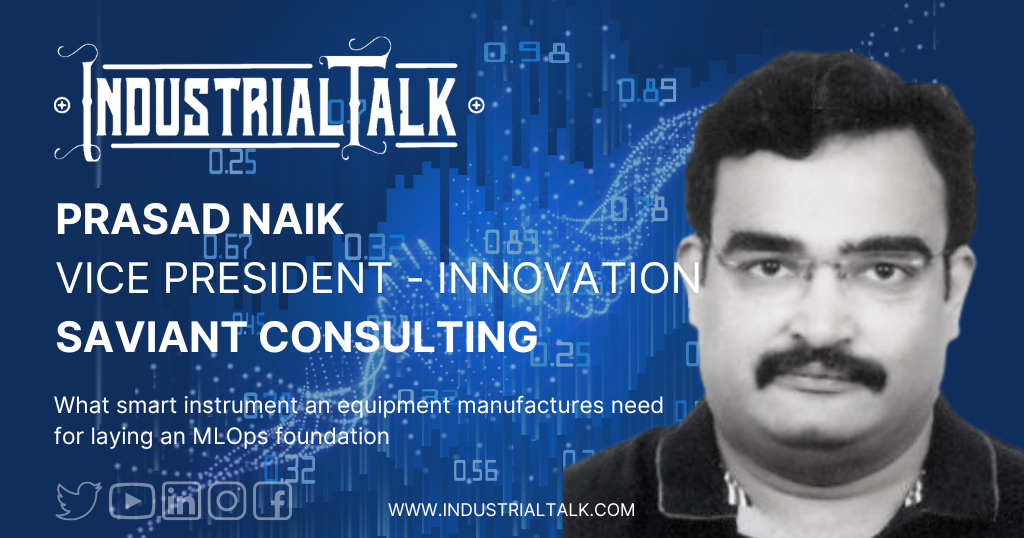
Industrial Talk is speaking with Prasad Naik, Vice President, Technology and Innovation at Saviant Consulting about “What smart instrument an equipment manufactures need for laying an MLOps foundation”. Get the answers to your “MLOps” questions along with Prasad's unique insight on the “How” on this Industrial Talk interview!
Finally, get your exclusive free access to the Industrial Academy and a series on “Why You Need To Podcast” for Greater Success in 2023. All links designed for keeping you current in this rapidly changing Industrial Market. Learn! Grow! Enjoy!
PRASAD NAIK'S CONTACT INFORMATION:
Personal LinkedIn: https://www.linkedin.com/in/prasadnaik/
Company LinkedIn: https://www.linkedin.com/company/saviant-consulting/
Company Website: https://www.saviantconsulting.com/
PODCAST VIDEO:
THE STRATEGIC REASON “WHY YOU NEED TO PODCAST”:
OTHER GREAT INDUSTRIAL RESOURCES:
NEOM: https://www.neom.com/en-us
ArduinoPro: https://www.arduino.cc/
Hitachi Vantara: https://www.hitachivantara.com/en-us/home.html
Industrial Marketing Solutions: https://industrialtalk.com/industrial-marketing/
Industrial Academy: https://industrialtalk.com/industrial-academy/
Industrial Dojo: https://industrialtalk.com/industrial_dojo/
We the 15: https://www.wethe15.org/
YOUR INDUSTRIAL DIGITAL TOOLBOX:
LifterLMS: Get One Month Free for $1 – https://lifterlms.com/
Active Campaign: Active Campaign Link
Social Jukebox: https://www.socialjukebox.com/
Industrial Academy (One Month Free Access And One Free License For Future Industrial Leader):
Business Beatitude the Book
Do you desire a more joy-filled, deeply-enduring sense of accomplishment and success? Live your business the way you want to live with the BUSINESS BEATITUDES…The Bridge connecting sacrifice to success. YOU NEED THE BUSINESS BEATITUDES!
TAP INTO YOUR INDUSTRIAL SOUL, RESERVE YOUR COPY NOW! BE BOLD. BE BRAVE. DARE GREATLY AND CHANGE THE WORLD. GET THE BUSINESS BEATITUDES!
Reserve My Copy and My 25% Discount
Transcript
SUMMARY KEYWORDS
data, furnace, machine learning, industrial, step, insights, prasad, ML, model, parameters, machine learning model, modeling, collect, results, find, talk, good, industry, Ops, predict
Welcome to the Industrial Talk podcast with Scott Mackenzie. Scott is a passionate industry professional dedicated to transferring cutting-edge industry focused innovations and trends while highlighting the men and women who keep the world moving. So put on your hard hat, grab your work boots, and let's go,
ongress is a go for next year:Yeah, everything great. Thanks, first of all, Scott, for having me here for this discussion. I'm sure I'm going to enjoy
you are, I guarantee you're gonna enjoy that I lay that I lay that out for you. Anyway, all right. For the listeners out there, let's get cracking with the conversation. Give us a little background on who you are, and why you're such an incredible professional. And then we're going to start talking a little bit about ML Ops and what that looks like ML meaning machine learning, and then what does that look like? You've given us some, really some insights into some use cases of how that applies. I like that puts a little meat on the bone. So give us a little background on who you are.
Yes, myself, Prasad, and I have about 29 years of experience in IT industry. Whoa,
I saw that out there. And I'm thinking to myself, what did you get started when you were three? Life is good to you.
Yes, I enjoyed this profession quite a lot. I started my career as a programmer, and over the year, I got a opportunity to work with different clients, different industries. And for past few years, I have been designing architectures for solving customer problems. And education wise, I have done Master's in Technology with specialization in data analytics, in software systems.
So here's, here's a side note. So data analytics, just FYI, I love it, I could I could sit there and immerse myself into data, and, you know, slice and dice it and try to make it communicate to me on on what it needs to be and what's it's trying to say that type of thing. But there's a point where back away, Scott, we got it, we're we're there. We're, you know, all? Do you find yourself hot? Is it just hard to pull away? Being in the world of data analytics? Sometimes?
Yes, sometimes it becomes difficult to pull away, as you rightly said, it's quite interesting to get insights from the data. And there are various ways to do that. So various ways and various techniques. Tools are also available these days. So it's quite intriguing. analyzing the data and taking insights from it.
Yeah, you run it through the office with a piece of paper. God, check this out. Oh, my gosh, this is fantastic. It's what it's telling me. All right. Let's talk a little bit about ML Ops. First off, what Yeah, what do you mean by that? What is that?
Yeah, ML Ops stands for machine learning operations. But before going into ML Ops, yeah. Let's talk about what is machine learning, basically. So
you're on it, baby. Yeah, I like that. Even better, let's go forget about that often. Yeah.
Machine learning is a branch of artificial intelligence that basically helps computer systems to learn new things and improve themselves using the past experiences. So, in other words, we have historical data available, because we apply some mathematical techniques and algorithms to train the computers to forecast or predict future events using the historical data. So that is machine learning. So, typically, machine learning lifecycle involves several steps. So, first step is data collection. Second step is data preparation. So, when we collect the data, it may not be in clean format, it may be missing data or maybe invalid data or there might be outliers in the data. So data preparation till a step helps us in cleaning that data and making ready for further processing. Next step is feature engineering, where we try to analyze the different elements of the data and identify which one are useful for our model. So, once features are defined this time, we go to the next step of modeling. So where we identify different algorithms and pick up the most suitable algorithms to solve the problem at our hand, and then we go to the step of modeling. Once model is ready, we need to test it. We need to validate it once we We have desired accuracy once we have good results from the model, then we can take it to the deployment stage. So all these steps
planning Yeah, did you say planning? Okay? Is down, if you're out on the video, you can see that my heads down because this is gold, I want to know, got it.
So all these steps are typically done manually. ML Ops helps us do these steps in an automatic fashion to improve the accuracy of the process and get quality results out of it. So there are very, very techniques.
Let me let me, let me just sort of recap what you said, these are seven steps. Alright, listeners, make sure if he went through it through quickly, here it is, you're going to be collecting. That's number one, two, you're going to be preparing the a god, I cleaned that data, whether you like it or not, it's got to be clean, because you don't want to start putting bad data into your your, your modeling, future engineering, so all of that good stuff that happens. And then we've got our modeling. For we've got our testing, five, we've got our validation six, and then we go into seven planning, then I do it.
The last one is deployment. So that waterfall model is ready. Yes, we need to put it into actual usage.
Okay. Don't listen to me, even though I'm a I'm a smarter person because of you. All right,
yeah. And one important point, I would like to mention here is, even though these tapes look like sequential steps, ML project is often experimental in nature, like, once we define a model, we may not get the results that we want. So we may have to repeat the process of identifying different set of algorithms, modeling, again, testing and validation. So this process may be repeated multiple times. So it's repetitive and experimental in nature by design.
So when you say it's, it's, it's pretty much iterative, you're saying, Okay, we're, we're gonna take, we're gonna take this road, and we're gonna just sort of see how that sort of, you try to do it right the first time, or you try to get as much traction as you possibly can, in your efforts in these steps. But sometimes you're gonna say, hey, let's adjust it. But but with each subsequent efforts, is it? Do you find the time is compressed a little bit more like? Like, it's not as long as you've laid the foundation? It did by looking at that, right?
Once the foundation is there, yes, some time may be reduced. But again, it depends on the problem. Because if you choose now a different set of complex algorithms, you may have to prepare data differently. So there are a lot of factors that drive how much time it will take. In the next iteration.
I'm all in I get it. So, note to self seven is deploy. Six is planning. And then of course, five testing and validation is Step five, that's make a note of that we're going to have an out all Industrial Talk. So if you're not, alright, so with all that said, you're going through this, you're you're working with me, we're having this back and forth conversation on what I am, what my expectations are. Take us through sort of an example of that, like our use case, like so that that let's put let's make it a little real here. Sure.
So, I would like to take an example of a recent ML Ops implementation that we did for a global furnace manufacturing company. So they manufacture industrial furnaces, and these are huge furnaces that are used for melting metals. And these furnaces use a lining inside it to give protection to the operators who are operating the furnace. So, this lining gets damaged over time and as it is used, so, it needs regular inspection to avoid any accidents or any damage to the furnace or to humans operating around the furnace. So, we are we implemented a machine learning based solution for them that collected the data from different sensors attached to the furnaces, and build machine learning models to predict remaining useful life of the lining. So basically, we were able to get pretty accurate results in predicting the remaining useful life and that helped. The manual inspection process was the report not a replacement for the manual process. But it was a aid to the manual process to ensure that lining gets replaced in time without any damage to property or human life.
Yeah, that's probably a pretty good thing. You've got a, you've got an asset that's under tremendous stress, you know, that whole furnace at that? That's, that's serious stuff. Right? So let me let me sort of talk to you back at what you said, Okay, so this, this company, the company came to you and said, Hey, we have this problem. The problem is, is that are our furnaces? We don't have good insights into the furnace, and we want to be able to have greater visibility into that environment, and and the components of the furnace to apply proper maintenance and repair. Got it? Yes. How do we do that? And and so what you do is you, you install these devices, now these devices? Are they in that environment? Or how do they? I mean, that's pretty serious these devices are so how do you how do you collect that data in that environment?
Yeah, there are multiple sensors that are installed inside of the furnaces. So some examples of the data collected is temperature, the current that is flowing, then the leakage to the ground. So there are several parameters, there were about 80 to 90 parameters that were getting collected from the furnace. And we had to churn out these different parameters to find out which were most appropriate for our modeling purpose.
And then from there, you're saying, okay, we can collect the data, we've got the data we've grew and 80 to 90 points of data collection, good. Got it, then you run it through you're you're you're running through those seven steps. And then but it's, it's important that you collect that data, then applying some sort of a machine learn. And does that ML solution continue to just learn more and more and get provide greater value? Is that what we're talking about?
Exactly. So that is the main point here that creating a model one time is not good enough. There are several reasons why we need to train our model again and again. So there might be new data that gets added there might be changed in the operating environment, that is one reason for changing of data, or the data that was used for testing and validation of the model could be different from the actual production environment. So because of these several reasons, we may have to remodel again. So another case is we did the model building for one particular client. So the location and the operating conditions were specific to that client. Now, same thing, if we do for furnace at other location or other client, then operating conditions could be different. So the first model that we develop may not be valid for this client. So again, we have to go through similar steps. So that is, again, an iterative and repeated process. And that is where importance of ML Ops comes into picture.
That brings up a really interesting point that I want to sort of probe a little bit, you have to there's a, there are so many other parameters that can apply to certain set of data. And one is geographic location. It could be material related, you know, how you're melting different material, whatever it might be. And all of that has requirements parameters that have to be modeled. Yeah, can you take that scenario, let's say let's just use the global furnace while they have they have furnaces everywhere, and to be able to pull that data pull it and then draw some sort of overview, look at all of the furnaces together and draw some additional clarifications on what's taking place.
Yes, it is possible to use all the data collected from different sites and different furnaces to build a kind of global model. So, depending on how similar the furnaces and their operating conditions and the geographic geographical conditions are such a global model may give good results, but it is very subjective to the operating conditions, the environments what kind of usage is there. So depending on that, we may have to create separate models or a global model may work. So that is why it is experimental in nature. We can't say that I build a model today and it will work for all the scenarios. No it's not like that. We have to keep experimenting and validating the results.
Yeah, Got it. That makes sense. And I know there's been a number of conversations too Is there a possibility of running simulations like operational simulations? You've got? You've got your ML Ops and what's happening? Is it? Is it a? Do you have the ability to run some simulations and saying, What if we do this? What if we did this and do some what ifs, once you have that information available?
Yes, that is part of the modeling, step modeling and test validation step. We tried different parameters, different features, we tried different algorithms, or to generate the models and the results. And we have to test and validate those results to find out which model is giving us the best results, and then use that model going forward into production environment.
Yeah, and with that said, so you're able to run those simulations, optimize the results, optimize the conclusions, optimize the insights, whatever you want to do it. But then that also begs the question that things change, assets begin to sort of underperform possibly just because it's so you're, you're constantly sort of adjusting. Is that not a part of that too, as well?
Yes, that's true. So we have to keep on validating the model that is declared in the environment. And if we see that accuracy, and the results are not within the defined range, or within the desired range, we may have to go back to the modeling step. Again, we have to collect the data again, do the modeling step again, and redeploy. Once we get the desired results.
So let's, let's talk a bit about okay, we've got it, we're collecting data, we're doing our thing, where, before, we're dealing with the operations, why is it for me as a customer as a furnace manufacturer? Why is that important to me? What what what benefits do I receive as a result of that? Is there some, is there some real tangible financial benefits? Of course, there's safety benefits of that that's a foregone conclusion. Explain to us a little bit about why that's important to me.
Yeah, for messes, if you take the same example, furnaces are expensive piece of equipment. So, if we are able to do the maintenance in time, we may prove we would be able to prevent the damage to the furnaces, so that will avoid any financial losses. If I take another example, let's say an energy producer using wind turbines, for them, there are several applications of machine learning. One is being able to forecast how much energy a particular wind turbine is going to produce on a particular day. Now, that also depends on several parameters like the environmental conditions from that day, the operating conditions of the turbine, so again, machine learning model comes into picture. So, for them, the ability to forecast energy is very important, because they are supplying to the grid. So a lot of things depend on how much energy they are able to produce on a particular day or even a particular our second application is same similar to the furnace use case that we discussed, that is being able to predict the maintenance requirements for the turbine. So, or wind turbine has several assemblies or several components. So if we are able to predict the failures of those components, or remaining useful life of those components, it will help doing the maintenance on time. And typically these turbines are located at remote areas. So when maintaining them is difficult. So if we know in advance what is likely to fail, then we may take precaution to replace those components or repair those components and avoid unnecessary repeat visits to the site. So that is another financial benefit.
Yeah, it's all bottom-line stuff, it makes sense that it's it's operational uptime, it's the ability to be able to take that asset down at the right time when, let's say, in the case of the wind turbines, it's like okay, forecast we're gonna do and it doesn't look like we're gonna have useful wind today. So let's, let's run a, you know, replacement of something, but that only comes through the ability to be able to know exactly the health of that asset. That's how I Yeah, and that's bottom line, and you want to keep those things spin and when they need to be spinning and, you know, doing what you need to do when they're not. So one last use case. I know that we talked about the water utilities give us a use case around that one.
Yeah, so for this water utility, they have smart meters installed in many geographical locations. So these meters keep providing data about the water consumption for a particular customer so it could be domestic customer cars. or commercial customer. So, these meters keep on sending the data which gets analyzed using machine learning model to identify the patterns in the usage of water. So, one important use case was to be able to identify the leakages that are happening or probably usage of water for washing machines or for washing hands. So different parameters and different patterns were identified using machine learning models. And that provided a good insight into the patterns geography buys, and utility company was then able to optimize the usage of the water by providing this information to their end customers.
See, that's so important, even going forward. I just think in general that, that we need to be as an industry as being part of this industry, is that we need to be committed to pulling that information and getting that insights to make real great tactical and strategic decisions. But that's not the case. What are some of the sort of the roadblocks, you're I'm having this conversation? I think it's great. I think it's all doable, and it's only going to get better, right? It's only going to get more nimble. Give us a reason why people are sort of not into it. What's you know, what's the pushback?
Yeah, one thing is, people are concerned that this, is this only a hype? Does machine learning adoption give us real benefits. That is one thing. Second aspect is the necessary skill set and know how to implement machine learning algorithms and modeling part. Third is the effort that is involved and patience that is required, particularly for implementation of machine learning, applications and physics. It's not like a typical application where once you have defined requirements, you just code and test that application and you're done. Machine learning. I'm repeating this, but experimentation is an important aspect. So you need to have patience to get the results that you desire. So that is
that here. Here's the reality. It's happening, whether you like it or not, and I look at it this way. You just accept it. Find the team, like Saviant. Find the team to help you with that journey. And you'll be okay. It's, it'll help you. All right, we have to wrap it up. How do people get a hold of you the same man? Prasad, he's the man I want to talk to him. How do I get a hold of you?
Yeah, you can reach out to me on my email address. It's Prasad dot nine at savvy and consulting.com.
Okay. That's pretty easy. Are you pretty active out there on LinkedIn?
Yes, I am active on LinkedIn.
There you go. I'm going to have that contact information too, as well. I'm going to have his I'm going to have his email as well as his LinkedIn profile, which is probably started, you know, just jacked full of insights and wisdom. But anyway, it's all gonna be out on Industrial Talk. You were absolutely wonderful. Thank you very much.
Thank you, Scott. It was nice talking to you. Thanks a lot.
It's always good, man. I always like it. All right, listeners. We're gonna wrap it up on the other side, you know that. So we're gonna have all the contact information for our friend Prasad, as well as Saviant out on Industrial Talk. So stay tuned, we will be right back.
You're listening to the Industrial Talk Podcast Network.
Another, another great conversation delivered with passion and enthusiasm. Prasad, Saviant Consulting, it's happening. You need Sherpas. You need people to help you on this journey. And you need to find those individuals to make that happen. Teams sabia, Prasad, definitely fall into that category of delivering incredible value insights, and solutions, big time. All right, again, we have events, go out to Industrial Talk.com events that are conference related events that are webcast related. And of course, we have all of the podcasts from just delivered by experts in their industry out there. And then of course, finally, we're talking about all of the marketing stuff that we're going to be producing out there so that you can be successful. Hear from me, I'll be the expert, as much as I possibly can. But anyway, be bold, be brave, daring greatly. Hang out with Prasad and you're gonna change the world we're gonna have another great conversation shortly