Ralf Haller with NNAISENSE
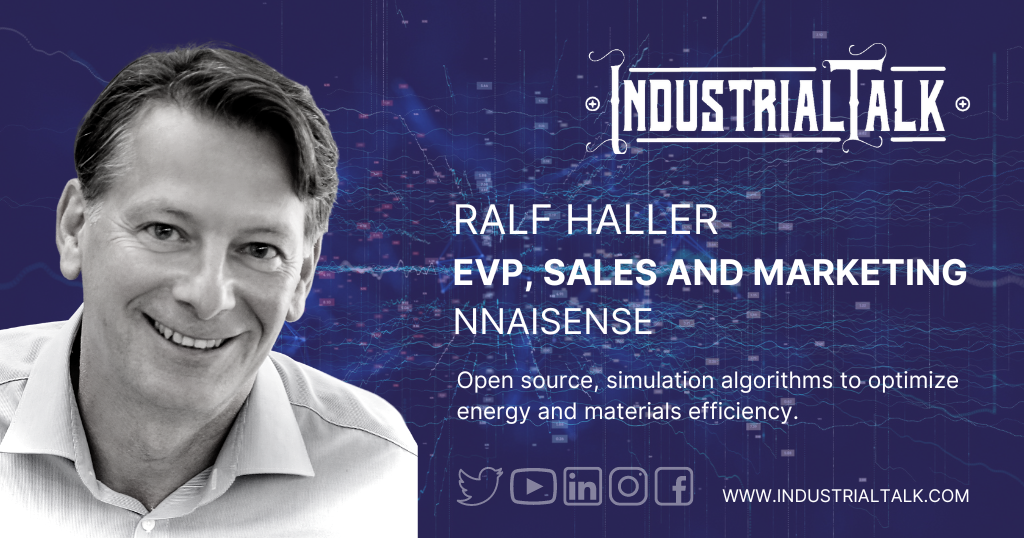
On this week's Industrial Talk we're talking to Ralf Haller, SVP of Sales and Marketing at NNAISENSE about “AI Based Manufacturing Simulations to Lower Cost and Improve Industrial Automation”. Get the answers to your “Simulation” questions along with Ralf's unique insight on the “How” on this Industrial Talk interview!
Finally, get your exclusive free access to the Industrial Academy and a series on “Why You Need To Podcast” for Greater Success in 2022. All links designed for keeping you current in this rapidly changing Industrial Market. Learn! Grow! Enjoy!
RALF HALLER'S CONTACT INFORMATION:
Personal LinkedIn: https://www.linkedin.com/in/ralfhaller/
Company LinkedIn: https://www.linkedin.com/company/nnaisense/
Company Website: https://nnaisense.com/
PODCAST VIDEO:
MORE VALUABLE INDUSTRIAL CONTENT FROM RALF:
Free PDF download on EvoTorch: https://industrialtalk.com/wp-content/uploads/2022/08/EvoTorch-primer.pdf
Past Podcast: https://agi-arc.com/resources/
Past Videos: https://agi-arc.com/resources/
Upcoming Events: March 2023, 3rd International Industrial AI Applications Summit
THE STRATEGIC REASON “WHY YOU NEED TO PODCAST”:
OTHER GREAT INDUSTRIAL RESOURCES:
NEOM: https://www.neom.com/en-us
Hitachi Vantara: https://www.hitachivantara.com/en-us/home.html
Industrial Marketing Solutions: https://industrialtalk.com/industrial-marketing/
Industrial Academy: https://industrialtalk.com/industrial-academy/
Industrial Dojo: https://industrialtalk.com/industrial_dojo/
We the 15: https://www.wethe15.org/
YOUR INDUSTRIAL DIGITAL TOOLBOX:
LifterLMS: Get One Month Free for $1 – https://lifterlms.com/
Active Campaign: Active Campaign Link
Social Jukebox: https://www.socialjukebox.com/
Industrial Academy (One Month Free Access And One Free License For Future Industrial Leader):
Business Beatitude the Book
Do you desire a more joy-filled, deeply-enduring sense of accomplishment and success? Live your business the way you want to live with the BUSINESS BEATITUDES…The Bridge connecting sacrifice to success. YOU NEED THE BUSINESS BEATITUDES!
TAP INTO YOUR INDUSTRIAL SOUL, RESERVE YOUR COPY NOW! BE BOLD. BE BRAVE. DARE GREATLY AND CHANGE THE WORLD. GET THE BUSINESS BEATITUDES!
Reserve My Copy and My 25% Discount
PODCAST TRANSCRIPT:
Ralf Haller Conversation
Thu, 8/25 9:40AM • 32:27
SUMMARY KEYWORDS
imts, evolutionary algorithms, industrial, simulation, open source, company, running, world, problem, objective, environment, manufacturing, experts, EvoTorch, solution, optimize, Ralf, years
SPEAKERS
Scott MacKenzie
Hey industrial Talk is brought to you by CAP Logistics. You want to minimize downtime. Absolutely. increase reliability, you bet ensure operational profitability. Yes you do. That means you need 24/7 365 insights into your supply chain, look no further cap Logistics, go to cap Logistics.com Or just call them. They're great people 800-227-2471 Also, AiDash. AiDash is on a mission to create a greener, cleaner, safer planet from space. AiDash helps core industries become more resilient, efficient, and sustainable through the power of satellites and AI. Go out to AiDash.com Find out more
welcome to the industrial talk podcast with Scott Mackenzie. Scott is a passionate industry professional dedicated to transferring cutting edge industry focused innovations and trends while highlighting the men and women who keep the world moving. So put on your hard hat, grab your work boots, and let's go
Alright, welcome to industrial talk a platform, a global platform and an ever expanding, it does really ecosystem that celebrates industry heroes, you all around the world because you're bold, you're brave, you dare greatly. You solve problems, you collaborate, you innovate, and you make my life and many around the world better. Thank you. That's why we celebrate you. This episode, we're talking simulation, we're talking about the technology, the solution that can simulate action. And so you can hone in more. It's exciting. We're talking with Ralf Haller, Executive Vice President with NNAISENSE. So let's get cracking. I'm always into simulation. And it's getting better. Plug in some data, create the defined your parameters, keep on going keep on refining, keep that asset up and running more and more each and every day. And and, and be able to simulate solutions before you even invest in them. I mean, look into solutions, such as what Nathan has out there. And what Ralf was talking about. It's, it's, it's important to do that. All right. Before we get into that conversation, we have 18 days, 18 days for IMTS. And you're saying to yourself, Scott, why? Why should I attend IMTS? Outside of the fact that it is the largest manufacturing show in this country? It's got multiple floors? It does. It's an amazing time to be able to collaborate. That's IMTS. Well, the reason is, and I'm looking at their website, which is@imts.com and it is powerful bought by a company called AMT. What we have is you got 90% of the visitors found new products and ideas. It's right there on our website. I'm not I'm not pulling this out of my ear here. Inspiration. 88% of the visitors found new solutions. Yes, you need new solutions. You need to figure that out. And a community 93% of visitors met their goals at IMTS. That means job shopping, women making manufacturing moves investor forum. It's all there. IMTS and the date set September 12th. Through the 17th. It is in Chicago. How can you complain about that food? Because everything wraps around food IMTS go there. If you're in manufacturing, and you're not there, you're missing out? That's the bottom line. All right, back to simulation of back to data back to the ability to be able to assimilate what is going on? nascent Ralf do an excellent job. Open, open architecture. It is it's a it's an amazing time. So therefore, let's get cracking with the conversation. Get your paper and pencil out because this is what it's all about. So enjoy the conversation with Ralf. Ralf. Welcome to industrial talk. Thank you very much all the way from Geneva. Geneva, right?
Actually, I mean storage for the companies in Lugano in the south of Switzerland. Hi, Scott. Nice. Nice to be here. And thanks for having
It still sounds great. And that whole part of the country or the world is absolutely spectacular. All right. Listeners, we're going to be talking a little bit about evolutionary algorithms. Don't, don't zero out on this. This is an important conversation. It is an important solution. And it is open source. And it is it's just needed for many applications and And NNAISENSE, just launch a product called Evo torch.ai. You can go out there, but it'll be out there on industrial talk, and you can find out more. But the best part about it is open source, which is pretty doggone cool. Before we get into that conversation, Ralf, give us a little background, give the listeners a little background on who you are.
Yeah, I'm in charge of Sales and Marketing at NNAISENSE. Now about two years, the company exists since eight years. Still a startup I would say although we have many customers also very large customers in the industrial space. I myself, nearly 30 years in high tech also been in Silicon Valley for six, seven years helped to launch one of the first Gigabit Ethernet switch routers long time ago. And since now, nearly also 18 years in Switzerland, and reset my own company. But NNAISENSE is a super interesting startup very much Silicon Valley style that attracted me and since about two years, I'm I'm there and helped to develop the customers and market the company. So I'm by training an electrical engineer, have a master's degree from German, very respected, respectable University called through and also studied in Silicon Valley. data engineering.
So let's, let's dive right in NNAISENSE. We talked about evolutionary algorithms, what? What's the problem? What's, what's the issue that we're, we're addressing in this conversation?
Yeah, so ultimately, it's it's complex. But let's try to maybe first start with an analogy. Nearly only about 10 years ago, everyone was talking about starting to talk about the application of deep neural networks. And they were very promising to solve lots of nonlinear problems, literally, in any industry you can think of right? But the problem at that time was you didn't have any compute environment to really run this and, and to do it, and then they came the GPU. Actually, it's a technology that was used for, for something different. So graphic graphic cards. But the notion there was that you had to paralyze things. And it turns out these deep neural networks, they work fantastically well and is paralyzed compute environments, GPUs as well. And actually, the founders of the company, they were one of the first if not the first to run these deep neural networks on GPUs. And we're even thanked by the leader in this space, the CEO a few years ago, for for doing so in front of 1000s of their employees, they were never paid for that there was one of the reasons survived. And we started eight years ago, the company to monetize that, and not just be scientists, we're doing things for for the, for the broader community. So anyway, but so now we have a situation where, of course the problems get more evolve, get get more complex, we have situations where you don't have to solve just for one objective, but you have to solve for multiple objectives. So multiple goals like in practically speaking, it could be that you try to say, improve the quality at the same time, you try to also do this, of course, very low cost, but these might be different angles, that you cannot have both, but you want to have to many, many other objectives that you can think of, and at the same time, you have lots and lots of knobs to to change based off of input features and parameters. So these problems are called multi objective, large scale optimization problems. And to solve that, there is actually deep neural networks are pretty bad, if not even impossible, and will take you ages to do this will be very, very expensive. Compute expensive. You literally have to spend hundreds of 1000s of computer which only the very big tech firms can can afford. An open AI has spent millions on just for example, for the shadow hand training that they had brought out two, three years ago. These guys well funded by the richest person in the world and one of the big IT company, they can afford it. But of course, the normal companies out there cannot and don't want to. So there is a compute environment and new compute environment needed. And this is exactly where evolutionary algorithms and evolutionary computing comes in. They're also highly paralyzed. And it's very important that you can do this conveniently. This is exactly where it would torch our platform has evolved over now. Literally eight years we have worked on that have and lots of projects in real world projects, I can talk to them in the minute. And of course, the desire was to now after this is field tested to also make this available to the world, the whole machine learning community and industrial is, is one is one area where this can be used, but it's literally infinite areas where this applies, because these optimization problems that just about anywhere, right, you can think of,
let me let me put on my, for lack of a better example here, my manufacturing hat, I am a small to mid size manufacturer, I have a desire to be more competitive, have greater insights into what's happening on my line, or whatever it might be what my objective needs to be. And then of course, with that I desire a greater resilience in my business. So I have some long term, and I'm, I'm competitive and whatever all of the drivers that that I'm so passionate about. Were to why me small to mid size manufacturer, where do I even begin? I'd say I hear you I understand sort of understand what do I do? Where? How would I proceed forward? What's my steps?
Yeah. So, in order to make this work, you must have a simulation environment to start with. And this is very obvious, because you can of course not try to play around with an existing running industrial processing plant or manufacturing facility. And your customers in your you would ruin your company by doing so right? Because for sure things will go wrong. So So you must be able to put this in some simulation. This could be either on a more high level system where you have your machines, it could be per machine that each machine is being simulated. So you must have the simulation environment. So I will show it real quick. writing something down optimizers.
Let me let me jump in real quick. So I have a machine. You're saying I can take that machine, whatever it is, and then put it into a simulation environment, and then run it. You know, this stays the same? Because I'm running. I'm running and running. And then I can put it over here. Simulation. Okay. I just want to make sure that that's that. Did I get that? Right? Did I paint that right picture? Yeah, more or
less? Yes. Okay. It's basically not physical anymore.
Okay, continue. I'm sorry, I want to I want to get this right. Because I think it's exciting stuff.
So the most important thing, of course, is that you have to you, you need to know, what is your objective? Right. So what you want to achieve what you want to actually do, yeah, you also you want to, let's say optimize for cost for energy usage. for it, which could be the same. years ago, it was maybe not, but now it's getting more and more, of course, literally the same, because energy is getting expensive, or, of course optimized for quality whatnot. And so, you, you have to define the objective to start with this pretty much also how evil torch works. So, you have to import the system, you have to define the objectives, then the third one is to define your problem. So what you want to actually so what is the problem environment, and then after that, and actually included with that, you also need to define on what compute you want to then run this, this optimization algorithm. So multiple CPU cores, or multiple GPUs could be hundreds of GPUs. And then you press the the button to launch and then the system is cranking out your, your optimized solution. So in a nutshell, that's more or less how it works. Of course, in reality, it's, it's, it's a bit more complicated, but but we need a simulation environment, and in the simulation environment, and you you run this evolutionary algorithms to, to optimize for problem solutions.
And then once that runs that simulation runs and reveals results, right? Let's do then you can take those results and put it into your real world environment, right? And say, okay, yeah, it's saying this, that and you see, we've got these simulations, and we're running these, this is what you need to do over here in the real world to optimize. Do you ever that makes sense? Now, do you ever go back and say, Okay, I'm in the real world, I've taken the results. Does this continue to learn over here? Right,
yeah. So of course, once you have done that, then the environment might change. So ideally, you want to set up a one to automate this in some way. This is where it actually a different term that comes into the play is something that we We have built as well at NNAISENSE. These are digital twins. So purely data driven digital twins, to be to be clear here, which goes far beyond the simulation. But of course, we work with simulation environments and use their input as well. And they, they basically help each other as well. So a hybrid solution between simulation and the digital twin is often what you need. So the EvoTorch is sorry, the the EvoTorch running on the pytorch framework is then optimizing on this digital twin. And it's basically then providing you the optimized solution, depending on what criteria that you're looking at. So maybe make this very practical. years ago, we got invited by the German automaker Audi, to build a self parking system was a very, yeah, it was a very innovative project. At that time, we didn't have all these tools that now it's available. And we only had a few months extra to realize to implement that. And so the the job was to train an Audi car model to self Park. And of course, do this better than any human being and not just you know, rectangular parking, but you very complex parking situations like you go in the field, right and lots of cars accordingly parked and I want to park back there, log it in, and then the car does it by itself. And we have done this by basically running this evolutionary algorithms in simulation, we had to build our own car simulators instead. But Audi provided was not sufficiently good, although they had something that the further improve that and then we had car simulator. And in this car simulator environment, we basically trained, then using these evolutionary algorithms, car parking over 500 years or so. And we're running this in just one day condensed because you can do this, right. So if you if a human being would have done that, it would have taken 500 years. But it's not very practical to wait 500 years for for training a machine. So we had to do this in virtual that shows you that you have to do this in virtual, of course, otherwise, it makes no sense. So once after 500 years, the system was converged was stable, and they couldn't further improve, then we transferred it back onto the real world environment of this IoT model car. So it was there needed to basically Park by itself, just using the onboard sensors, which was a camera and an infrared sensor and other things in the car get actually better than any human being in an aircraft. So that's an example where it is worked very well. And you can now take the Audi car and put their machine my friend manufacturing facility and industrial process at the end is a similar concept, you train this in simulation, once it works there, to transfer it back. Of course, first run it in some tests in this case was a model car and maybe you have some other tests facility of your plant, right and if it works developed, then give it a go. And the system is running this optimized, much, much better than any human being because we human beings are very good in cup in certain things. But we are very bad. For example, in the crunching lots hundreds of 1000s of of inputs data, right for us to get to do to do this is completely impossible. And and of course machine learning. And these algorithms, they do this fantastically well, if they have the right tools and the right environment to do it. And ever torch is now exactly this. It's field tested, it's super easy to use. And it's highly, highly scalable, much more than anything else you find out there. And the launch was super, super successful. within 24 hours, we got more than 400. GitHub stars, so more than big tech firms got in years. It's very exciting for us. So obviously, there's a big need for for this out there. Which doesn't totally surprise us. Because we see this of course. And yeah, we you know, pursuing this more and more
points of clarification Ralf when you did that Audi, that you provided that Audi example. Yeah, for parking. How quick. So somebody Audi comes knocking on your door, hey, now we're interested in this. And then you say, hey, that's great. Give us a timeline on when you achieved well, from our perspective, success.
Yeah. Well, this was before I joined the company, but other folks told me this was a six month project, because they wanted to demonstrate the outcome at the biggest Machine Learning Conference in the world at the time with an actually I think 5000 People watch this demo then live. And then it was shown that at the store, we had a six month hard date to do it. Today. It would be much faster to do because So we have, of course, this expertise, we have this platform, we have done many, many other projects that I could speak about. So say that of course helps, right? So and So six months before something like this is super fast. And I say, but today, you can do it much faster, quicker arm. That's, that's for sure.
So the other question I have when we start talking about a simulation environment, you define objective, there has to be a discussion around the parameters like, you know, in the case of the parking analogy, the car will travel at this rate and do this and this, you have to sort of define a little bit of the parameters before really the machine starts to learn and say, No, we can do, we can do this than the other. But there has to be a baseline understanding. Right? Right. Yeah,
that's exactly the job of the simulator. And you basically need to define what, what what in what environment, you actually are, right? What is your space, they call it, that you're looking for optimal solutions. And what I know is that has to be defined. Totally Correct, right. And, of course, it's a different thing for an oil refinery or class production or a car. But the principle is the same. You need experts, top experts in this field to basically write such simulations, such simulation environments, typically, that is needed, we can do it, of course, as well for them. Since we were looking at it's purely data driven, so we don't really need to understand how class or how metal or how oil is refined, you're looking at is purely data driven. So you give us hundreds of sensor data control data, right. And with that, we fill our refill our platform, and then we understand just based on this input data, what's going on. And we don't have to be expert in that that is the beauty of this approach as well. So you don't have to hire very, very expensive experts. To do that. It's doable by you still need to have, of course, an understanding of data, data and machine learning. In this case, that's clear. But with this ABL torch tool, at least the optimization part is something you don't even need to be a top expert at all. It's very easy to do. So
see, this was interesting, because this has piqued my interest. So now I'm a manufacturer got my manufacturing hat on, I hear what you're saying, I go out to EVO ta torch.ai. I'm looking at it right. I don't have the skill sets. I don't, let's just lay that out right now. But I'm able to connect with somebody that NNAISENSE or because I've got to find somebody to trust, I got the value proposition, I see the need for it. But I need to find somebody to help me with this journey. Can I just go to NNAISENSE? And say, Hey, I see your EvoTorch, I just I like it, I'm interested in trying it out do whatever, where do I start? Is that is that? Can I come to you guys?
Yeah, B, B are originally and still are. And machine learning engineering service provider. So we have built these projects for large industrial customers mainly also in other industries, but mainly there and have investors from that space, as well. So we certainly do such projects. That's, that's correct. But you could, of course, also hire other other folks that I can do it. Now we have, of course, the unique understanding of all the details of evil torch, it is open source, you can look at the code. So if you're a big company, and and you have, you know, dozens of data scientists, they can look at each line of code will understand that. And then if they still need support from us, yeah, we can we can provide that that is something we are still doing. But theoretically, practically, you could give such people know, after you hire them, to research projects by yourself, and you know, or we help you to get started. And then you maintain it by yourself this of course also a possibility. So it's you're not dependent on this. Yeah. Because just like any open source, yes, yeah. Just like any neural net, right?
I if I, if I find somebody that I can work with, and then I learn, then I have that internal capability to be able to just say, evil source, like, we did it over here. We ran through we achieved whatever our objectives and improvement efficiency, whatever it might be. I think we could do it over here now. And we just do the same process and we got the code and we do, you can begin to scale it within within your organization to you know, achieve the objectives that you sort of lay out I think it's exciting. I like the fact that It's open source.
Yeah, yeah, sure, it's a good approach in general, by applying machine learning or other new technologies by trying it out in, in a small problem and small problem space and settings. And if it works there, then expand on this guy. And, and, and yeah, so that's, that's very important. So you maybe don't want to start with the Audi project that I just mentioned, it was super, super complex deal by itself. But if you have to team why not? I mean, it's
alright, it's, well, at least it's doable. And, and when we start talking about open source, let's say I go down the road, and I do my thing. How do I loop back with you and say, hey, you know, what I did over here, I learned this I did open sources or to buy bilateral, right. It's like going back and forth. I can share my insights, my wisdoms, my whatever, is exactly the community.
Yeah, we are building a community that says that's probably the number one reason to go open source we want to share with you have done it. Now, we hope, of course, that many other smart experts out there will be able to take this and provide it and it was very exciting to see in our own slack. Communication, when we launched this, when they had the press release, launch, and when they you know, published it in GitHub and read it in other communication channels, suddenly, all these top top highly respected, machine learning experts came on. And then our guys were like, oh, let's, let's, let's say hi to him, right? Because we know them, of course, or no of them, maybe may have spoken. And then they congratulated us, they said, thanks a lot for bringing this out. This is super exciting stuff, right? So of course, we hope that this and quite sure that these folks now they bring their own solutions in you know, apply this and then publish this. And with that, we bring the whole community forward. So that's, that's, of course, the whole the whole point of having this open source that we don't think now our company, we, we are smart enough to think about all the applications absolutely impossible. So the world out there is too complex into the many, many smart people and trying to leverage this and at the start was super, super exciting. So lots of people are excited, and are now looking at this and start to apply it. So that's exactly what we're seeing. Yeah,
I think it's great. I think it's exciting. I think it's definitely in the direction that that many industry organizations need to go. I like the fact that one it's open source to, it allows the small to midsize companies, whatever, let's say manufacturers to participate in this digital transformation world, this machine learning opportunity, this AI whatever it might be, and be able to do it in in a way that helps them be competitive, more efficient, whatever it is, I I'm all in Ralf, how does somebody get a hold of you, if they want to talk to you about what we just discussed,
I can just send an email at NNAISENSE.com, or my first name dot last name@NNAISENSE.com. Of course, you will go to the website of either NNAISENSE.com You know, and so then or go to evil torch website, go to the community slack there, then you immediately have our experts in there, if you have specific things for that, you know, they are noticed much, much better, of course than me. And they have built this. And we have actually 20 years of expertise in the company for this evolutionary algorithm stuff. So we are total experts and pioneers out there as well. I've invented lots of things prior to starting the company. And now we, of course have to solve many industry projects. We're applying this and as a last step we now open sourced is this, this library is this tool box that allows people to do this very efficiently. And scale scale is the most important thing as I tried in the beginning. Because without that it's just simply too expensive to do it. But the example of the OpenNI eyeshadow hand, it's cost belt. I heard numbers of beyond a million dollars to train this algorithm. Possibly you can do this for maybe a couple of 100 bucks or so with if you completely paralyze this. And this makes it appear suddenly extremely affordable. For literally anyone.
Yes, see, I love it. And I just think that it's so excited because we as a community. When I say community, industry, people around the world, we benefit from this. And because it's approachable and it's doable. Then we all benefit. Excellent job Ralf Thank you very much, sir. Well for being on industrial talk, thank you, I'm about to start talking to you. Excellent. All right, listeners, we're gonna have all the contact information for Ralf NNAISENSE, Evo touch all of the stuff that we've talked about neural net, and so on and so forth out on industrial talk. So don't come crashing down my wall saying I can't get a hold of him. Because it's all on the website. It's right there. And you have all of the the ability to be able to do what you need to do. So anyway, so stay tuned, we will be right back.
You're listening to the industrial talk, Podcast Network.
All right. Again, thank you very much for joining industrial talk, this ever expanding ecosystem of problem solvers, leaders, right here. You need to educate, collaborate, and definitely innovate. It starts here. Go out to industrial talk.com. Find out more definitely, this is where it's at. And also, thank you to Ralf Haller. NNAISENSE. Is the company, the solution? The open source solution? Is EVO torch, I'll have the PDF all the information about that. You need to simulate, you need to begin thinking about that. The your competition, how do you optimize your assets? How do you run those simulations? How do you create those parameters, all their open source, all of the contact all of the the websites that are necessary for you to be successful? Again, let's make a quick little reminder. This is going to be September 12. Through the 17th. I'll be broadcasting on site. If you are in manufacturing, yes, if you are in manufacturing, you need to be at IMTS 2022, September 12 through the 17th. It is a must. It has technology. It has connections, because you're all about education got to did. You're all about collaborating. They're all they're multi for you find them. And of course innovation. No doubt about it. It's at I am TS All right. Thank you very much. Appreciate you joining me this day on industrial talk and talking about simulation. Excellent job NNAISENSE, all of that contact and reach out to him. I'll have a stat guard out there. So thank you again for supporting industrial talk. Remember, subscribe, please subscribe. We're gonna have another great conversation shortly. So do not go away. Stay tuned.
Transcript
welcome to the industrial talk podcast with Scott Mackenzie. Scott is a passionate industry professional dedicated to transferring cutting edge industry focused innovations and trends while highlighting the men and women who keep the world moving. So put on your hard hat, grab your work boots, and let's go
Alright, welcome to industrial talk a platform, a global platform and an ever expanding, it does really ecosystem that celebrates industry heroes, you all around the world because you're bold, you're brave, you dare greatly. You solve problems, you collaborate, you innovate, and you make my life and many around the world better. Thank you. That's why we celebrate you. This episode, we're talking simulation, we're talking about the technology, the solution that can simulate action. And so you can hone in more. It's exciting. We're talking with Ralf Haller, Executive Vice President with NNAISENSE. So let's get cracking. I'm always into simulation. And it's getting better. Plug in some data, create the defined your parameters, keep on going keep on refining, keep that asset up and running more and more each and every day. And and, and be able to simulate solutions before you even invest in them. I mean, look into solutions, such as what Nathan has out there. And what Ralf was talking about. It's, it's, it's important to do that. All right. Before we get into that conversation, we have 18 days, 18 days for IMTS. And you're saying to yourself, Scott, why? Why should I attend IMTS? Outside of the fact that it is the largest manufacturing show in this country? It's got multiple floors? It does. It's an amazing time to be able to collaborate. That's IMTS. Well, the reason is, and I'm looking at their website, which is@imts.com and it is powerful bought by a company called AMT. What we have is you got 90% of the visitors found new products and ideas. It's right there on our website. I'm not I'm not pulling this out of my ear here. Inspiration. 88% of the visitors found new solutions. Yes, you need new solutions. You need to figure that out. And a community 93% of visitors met their goals at IMTS. That means job shopping, women making manufacturing moves investor forum. It's all there. IMTS and the date set September 12th. Through the 17th. It is in Chicago. How can you complain about that food? Because everything wraps around food IMTS go there. If you're in manufacturing, and you're not there, you're missing out? That's the bottom line. All right, back to simulation of back to data back to the ability to be able to assimilate what is going on? nascent Ralf do an excellent job. Open, open architecture. It is it's a it's an amazing time. So therefore, let's get cracking with the conversation. Get your paper and pencil out because this is what it's all about. So enjoy the conversation with Ralf. Ralf. Welcome to industrial talk. Thank you very much all the way from Geneva. Geneva, right?
Actually, I mean storage for the companies in Lugano in the south of Switzerland. Hi, Scott. Nice. Nice to be here. And thanks for having
It still sounds great. And that whole part of the country or the world is absolutely spectacular. All right. Listeners, we're going to be talking a little bit about evolutionary algorithms. Don't, don't zero out on this. This is an important conversation. It is an important solution. And it is open source. And it is it's just needed for many applications and And NNAISENSE, just launch a product called Evo torch.ai. You can go out there, but it'll be out there on industrial talk, and you can find out more. But the best part about it is open source, which is pretty doggone cool. Before we get into that conversation, Ralf, give us a little background, give the listeners a little background on who you are.
Yeah, I'm in charge of Sales and Marketing at NNAISENSE. Now about two years, the company exists since eight years. Still a startup I would say although we have many customers also very large customers in the industrial space. I myself, nearly 30 years in high tech also been in Silicon Valley for six, seven years helped to launch one of the first Gigabit Ethernet switch routers long time ago. And since now, nearly also 18 years in Switzerland, and reset my own company. But NNAISENSE is a super interesting startup very much Silicon Valley style that attracted me and since about two years, I'm I'm there and helped to develop the customers and market the company. So I'm by training an electrical engineer, have a master's degree from German, very respected, respectable University called through and also studied in Silicon Valley. data engineering.
So let's, let's dive right in NNAISENSE. We talked about evolutionary algorithms, what? What's the problem? What's, what's the issue that we're, we're addressing in this conversation?
for for doing so in front of:let me let me put on my, for lack of a better example here, my manufacturing hat, I am a small to mid size manufacturer, I have a desire to be more competitive, have greater insights into what's happening on my line, or whatever it might be what my objective needs to be. And then of course, with that I desire a greater resilience in my business. So I have some long term, and I'm, I'm competitive and whatever all of the drivers that that I'm so passionate about. Were to why me small to mid size manufacturer, where do I even begin? I'd say I hear you I understand sort of understand what do I do? Where? How would I proceed forward? What's my steps?
Yeah. So, in order to make this work, you must have a simulation environment to start with. And this is very obvious, because you can of course not try to play around with an existing running industrial processing plant or manufacturing facility. And your customers in your you would ruin your company by doing so right? Because for sure things will go wrong. So So you must be able to put this in some simulation. This could be either on a more high level system where you have your machines, it could be per machine that each machine is being simulated. So you must have the simulation environment. So I will show it real quick. writing something down optimizers.
Let me let me jump in real quick. So I have a machine. You're saying I can take that machine, whatever it is, and then put it into a simulation environment, and then run it. You know, this stays the same? Because I'm running. I'm running and running. And then I can put it over here. Simulation. Okay. I just want to make sure that that's that. Did I get that? Right? Did I paint that right picture? Yeah, more or
less? Yes. Okay. It's basically not physical anymore.
Okay, continue. I'm sorry, I want to I want to get this right. Because I think it's exciting stuff.
So the most important thing, of course, is that you have to you, you need to know, what is your objective? Right. So what you want to achieve what you want to actually do, yeah, you also you want to, let's say optimize for cost for energy usage. for it, which could be the same. years ago, it was maybe not, but now it's getting more and more, of course, literally the same, because energy is getting expensive, or, of course optimized for quality whatnot. And so, you, you have to define the objective to start with this pretty much also how evil torch works. So, you have to import the system, you have to define the objectives, then the third one is to define your problem. So what you want to actually so what is the problem environment, and then after that, and actually included with that, you also need to define on what compute you want to then run this, this optimization algorithm. So multiple CPU cores, or multiple GPUs could be hundreds of GPUs. And then you press the the button to launch and then the system is cranking out your, your optimized solution. So in a nutshell, that's more or less how it works. Of course, in reality, it's, it's, it's a bit more complicated, but but we need a simulation environment, and in the simulation environment, and you you run this evolutionary algorithms to, to optimize for problem solutions.
And then once that runs that simulation runs and reveals results, right? Let's do then you can take those results and put it into your real world environment, right? And say, okay, yeah, it's saying this, that and you see, we've got these simulations, and we're running these, this is what you need to do over here in the real world to optimize. Do you ever that makes sense? Now, do you ever go back and say, Okay, I'm in the real world, I've taken the results. Does this continue to learn over here? Right,
he crunching lots hundreds of:points of clarification Ralf when you did that Audi, that you provided that Audi example. Yeah, for parking. How quick. So somebody Audi comes knocking on your door, hey, now we're interested in this. And then you say, hey, that's great. Give us a timeline on when you achieved well, from our perspective, success.
time with an actually I think:So the other question I have when we start talking about a simulation environment, you define objective, there has to be a discussion around the parameters like, you know, in the case of the parking analogy, the car will travel at this rate and do this and this, you have to sort of define a little bit of the parameters before really the machine starts to learn and say, No, we can do, we can do this than the other. But there has to be a baseline understanding. Right? Right. Yeah,
that's exactly the job of the simulator. And you basically need to define what, what what in what environment, you actually are, right? What is your space, they call it, that you're looking for optimal solutions. And what I know is that has to be defined. Totally Correct, right. And, of course, it's a different thing for an oil refinery or class production or a car. But the principle is the same. You need experts, top experts in this field to basically write such simulations, such simulation environments, typically, that is needed, we can do it, of course, as well for them. Since we were looking at it's purely data driven, so we don't really need to understand how class or how metal or how oil is refined, you're looking at is purely data driven. So you give us hundreds of sensor data control data, right. And with that, we fill our refill our platform, and then we understand just based on this input data, what's going on. And we don't have to be expert in that that is the beauty of this approach as well. So you don't have to hire very, very expensive experts. To do that. It's doable by you still need to have, of course, an understanding of data, data and machine learning. In this case, that's clear. But with this ABL torch tool, at least the optimization part is something you don't even need to be a top expert at all. It's very easy to do. So
see, this was interesting, because this has piqued my interest. So now I'm a manufacturer got my manufacturing hat on, I hear what you're saying, I go out to EVO ta torch.ai. I'm looking at it right. I don't have the skill sets. I don't, let's just lay that out right now. But I'm able to connect with somebody that NNAISENSE or because I've got to find somebody to trust, I got the value proposition, I see the need for it. But I need to find somebody to help me with this journey. Can I just go to NNAISENSE? And say, Hey, I see your EvoTorch, I just I like it, I'm interested in trying it out do whatever, where do I start? Is that is that? Can I come to you guys?
Yeah, B, B are originally and still are. And machine learning engineering service provider. So we have built these projects for large industrial customers mainly also in other industries, but mainly there and have investors from that space, as well. So we certainly do such projects. That's, that's correct. But you could, of course, also hire other other folks that I can do it. Now we have, of course, the unique understanding of all the details of evil torch, it is open source, you can look at the code. So if you're a big company, and and you have, you know, dozens of data scientists, they can look at each line of code will understand that. And then if they still need support from us, yeah, we can we can provide that that is something we are still doing. But theoretically, practically, you could give such people know, after you hire them, to research projects by yourself, and you know, or we help you to get started. And then you maintain it by yourself this of course also a possibility. So it's you're not dependent on this. Yeah. Because just like any open source, yes, yeah. Just like any neural net, right?
I if I, if I find somebody that I can work with, and then I learn, then I have that internal capability to be able to just say, evil source, like, we did it over here. We ran through we achieved whatever our objectives and improvement efficiency, whatever it might be. I think we could do it over here now. And we just do the same process and we got the code and we do, you can begin to scale it within within your organization to you know, achieve the objectives that you sort of lay out I think it's exciting. I like the fact that It's open source.
Yeah, yeah, sure, it's a good approach in general, by applying machine learning or other new technologies by trying it out in, in a small problem and small problem space and settings. And if it works there, then expand on this guy. And, and, and yeah, so that's, that's very important. So you maybe don't want to start with the Audi project that I just mentioned, it was super, super complex deal by itself. But if you have to team why not? I mean, it's
alright, it's, well, at least it's doable. And, and when we start talking about open source, let's say I go down the road, and I do my thing. How do I loop back with you and say, hey, you know, what I did over here, I learned this I did open sources or to buy bilateral, right. It's like going back and forth. I can share my insights, my wisdoms, my whatever, is exactly the community.
Yeah, we are building a community that says that's probably the number one reason to go open source we want to share with you have done it. Now, we hope, of course, that many other smart experts out there will be able to take this and provide it and it was very exciting to see in our own slack. Communication, when we launched this, when they had the press release, launch, and when they you know, published it in GitHub and read it in other communication channels, suddenly, all these top top highly respected, machine learning experts came on. And then our guys were like, oh, let's, let's, let's say hi to him, right? Because we know them, of course, or no of them, maybe may have spoken. And then they congratulated us, they said, thanks a lot for bringing this out. This is super exciting stuff, right? So of course, we hope that this and quite sure that these folks now they bring their own solutions in you know, apply this and then publish this. And with that, we bring the whole community forward. So that's, that's, of course, the whole the whole point of having this open source that we don't think now our company, we, we are smart enough to think about all the applications absolutely impossible. So the world out there is too complex into the many, many smart people and trying to leverage this and at the start was super, super exciting. So lots of people are excited, and are now looking at this and start to apply it. So that's exactly what we're seeing. Yeah,
I think it's great. I think it's exciting. I think it's definitely in the direction that that many industry organizations need to go. I like the fact that one it's open source to, it allows the small to midsize companies, whatever, let's say manufacturers to participate in this digital transformation world, this machine learning opportunity, this AI whatever it might be, and be able to do it in in a way that helps them be competitive, more efficient, whatever it is, I I'm all in Ralf, how does somebody get a hold of you, if they want to talk to you about what we just discussed,
I can just send an email at NNAISENSE.com, or my first name dot last name@NNAISENSE.com. Of course, you will go to the website of either NNAISENSE.com You know, and so then or go to evil torch website, go to the community slack there, then you immediately have our experts in there, if you have specific things for that, you know, they are noticed much, much better, of course than me. And they have built this. And we have actually 20 years of expertise in the company for this evolutionary algorithm stuff. So we are total experts and pioneers out there as well. I've invented lots of things prior to starting the company. And now we, of course have to solve many industry projects. We're applying this and as a last step we now open sourced is this, this library is this tool box that allows people to do this very efficiently. And scale scale is the most important thing as I tried in the beginning. Because without that it's just simply too expensive to do it. But the example of the OpenNI eyeshadow hand, it's cost belt. I heard numbers of beyond a million dollars to train this algorithm. Possibly you can do this for maybe a couple of 100 bucks or so with if you completely paralyze this. And this makes it appear suddenly extremely affordable. For literally anyone.
Yes, see, I love it. And I just think that it's so excited because we as a community. When I say community, industry, people around the world, we benefit from this. And because it's approachable and it's doable. Then we all benefit. Excellent job Ralf Thank you very much, sir. Well for being on industrial talk, thank you, I'm about to start talking to you. Excellent. All right, listeners, we're gonna have all the contact information for Ralf NNAISENSE, Evo touch all of the stuff that we've talked about neural net, and so on and so forth out on industrial talk. So don't come crashing down my wall saying I can't get a hold of him. Because it's all on the website. It's right there. And you have all of the the ability to be able to do what you need to do. So anyway, so stay tuned, we will be right back.
You're listening to the industrial talk, Podcast Network.
uring, you need to be at IMTS: