Bhushan Sardesai with Saviant Consulting
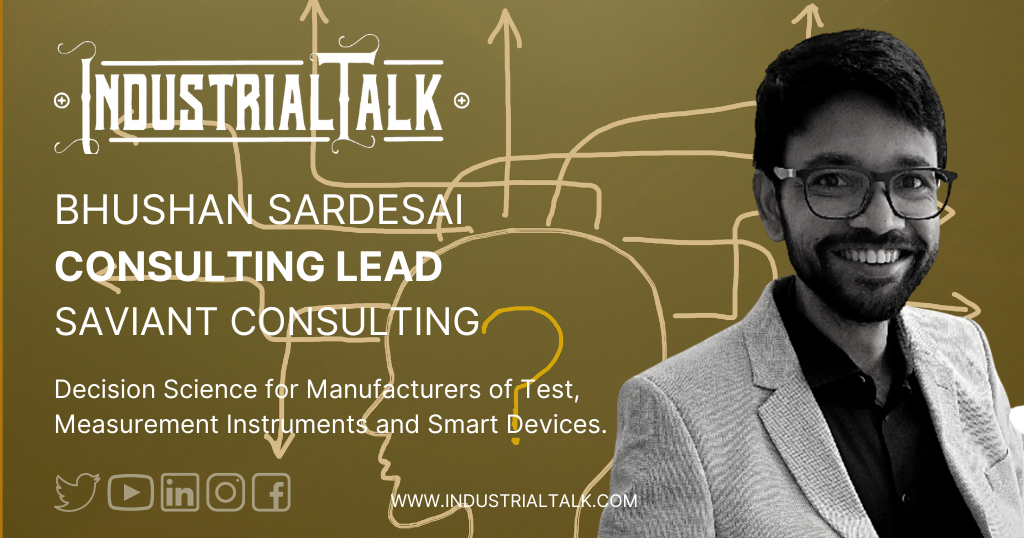
Industrial Talk is speaking with Bhushan Sardesai, Consulting Lead – Saviant Consulting about “Decision Science for Manufacturers of Test, Measurement Instruments and Smart Devices”. Get the answers to your “Decision Science” questions along with Bhushan's unique insight on the “How” on this Industrial Talk interview!
Finally, get your exclusive free access to the Industrial Academy and a series on “Why You Need To Podcast” for Greater Success in 2023. All links designed for keeping you current in this rapidly changing Industrial Market. Learn! Grow! Enjoy!
BHUSHAN SARDESAI'S CONTACT INFORMATION:
Personal LinkedIn: https://www.linkedin.com/in/bhushansardesai/
Company LinkedIn: https://www.linkedin.com/company/saviant-consulting/
Company Website: https://www.saviantconsulting.com/
PODCAST VIDEO:
THE STRATEGIC REASON “WHY YOU NEED TO PODCAST”:
OTHER GREAT INDUSTRIAL RESOURCES:
NEOM: https://www.neom.com/en-us
ArduinoPro: https://www.arduino.cc/
Hitachi Vantara: https://www.hitachivantara.com/en-us/home.html
Industrial Marketing Solutions: https://industrialtalk.com/industrial-marketing/
Industrial Academy: https://industrialtalk.com/industrial-academy/
Industrial Dojo: https://industrialtalk.com/industrial_dojo/
We the 15: https://www.wethe15.org/
YOUR INDUSTRIAL DIGITAL TOOLBOX:
LifterLMS: Get One Month Free for $1 – https://lifterlms.com/
Active Campaign: Active Campaign Link
Social Jukebox: https://www.socialjukebox.com/
Industrial Academy (One Month Free Access And One Free License For Future Industrial Leader):
Business Beatitude the Book
Do you desire a more joy-filled, deeply-enduring sense of accomplishment and success? Live your business the way you want to live with the BUSINESS BEATITUDES…The Bridge connecting sacrifice to success. YOU NEED THE BUSINESS BEATITUDES!
TAP INTO YOUR INDUSTRIAL SOUL, RESERVE YOUR COPY NOW! BE BOLD. BE BRAVE. DARE GREATLY AND CHANGE THE WORLD. GET THE BUSINESS BEATITUDES!
Reserve My Copy and My 25% Discount
Transcript
Welcome to the Industrial Talk podcast with Scott Mackenzie. Scott is a passionate industry professional dedicated to transferring cutting edge industry focused innovations and trends while highlighting the men and women who keep the world moving. So put on your hard hat, grab your work boots, and let's get Alright, what's
good. Thank you very much for joining Industrial Talk and your continued support. We are building a juggernaut of industry professionals in this ecosystem, that want to collaborate, want to solve problems, and help everyone succeed, because we need you. That's what's Industrial Talk. That's why you need to be a part of it go out to Industrial Talk.com Find out more, we will be more than happy and subscribe to the podcast as well as, of course, all the videos, it never ends. We're just trying to do our part, with your help to continue to educate. But shot is in the hot seat, sabia consulting, we're going to be talking about decision science, let's get cracking. Does decision science? Yeah, it's all great to have to data analytics pulling that data. It's all good. What do we do with it? What kind of decisions are we going to be making? That to me is a real important question. And that's why Bhushan and Team Saviant is out there and trying to do the best they can in being able to deliver insights and solutions for not just manufacturing, but for all of the other industries that exist out there that are all engaged with this connected, you know, IoT world, which is important. It's important, because you need to be more efficient. And we're going to be doing a series on F1 versus er, where industry wants to get in with F1. Because F1 I'm a real big time, passionate, F1 guy and the reason I'm a big passionate F1 Guys, because I find that that is like the the zenith right at the top. So they have these assets, the cars, they're able to see every aspect of that particular vehicle and make real time adjustments to optimize those assets. And that's where we need to get to, it's hard, don't get me wrong. And sometimes it's a point of diminishing returns. But the reality is, is that in F1, they can see everything in manufacturing, you need to see everything. That's what we're going to be doing a series on. And I hope you enjoy that. All right. Again, I'm going to continue to harp on the fact that you need to get engaged. We have conferences that are coming up, if you're going to be at some conferences, and we're going to be there, you need to look me up, we've got four on the calendar right now probably will expand, probably get a couple more in there. And we just broadcast from those locations. So make a point, go out to Industrial Talk, find the conferences. We're doing FABTECH we're doing Hexagon, SMRP, which is a pretty and of course, a crew of at this particular time, go check that out. All right, let's get on with the decision science conversation with Bhushan, and tell you you're going to enjoy his passion. So let's get cracking. With Sean, welcome to Industrial Talk. Thank you very much for joining all the way from India. How are you doing today?
Yeah, I am very excited. Thanks so much for inviting me.
What time is there?
It's 11pm.
So that listeners That's commitment right there. That's commitment. Yeah. More than I am. Congratulations, a live VM. That's fast. Fantastic. All right. For the listeners out there. Give us a little background on who Bhushan is, please.
Yeah, so I am Bhushan Sardesai, they say I am a consulting lead at Saviant. But I think before delving deep into that, a little bit of background. So I have been working in tech for almost 1112 years. Most of it is in data analytics. So development as well as consulting. Interestingly, I've also worked in manufacturing setup and there's there's a good story which I can tell how did I get there? Apart from my works in terms of education, I've done an engineering in it and an MBA in operations. And overall, I think in terms of education as well as in terms of work ethics, I have that amalgamation of tech and manufacturing. And beyond this professional career. I am also a former national level tennis player, and you will find me in the tennis court in my free time
theater show. That's pretty cool. Now that's, that's a piece of information that is really interesting. You know, when I play tennis, I just chase the ball around. It's, it's, I miss it, and then I chase after it. And that's sort of the, the game I play. And if you're looking out on the video, if you're out on YouTube, you'll say that, my gosh, he must have started his career at age five, because he is quite youthful. Bring it a level of skills that are second to none. All right. So let's level set here, let's let's, let's make sure we understand some stuff. I've had conversations that are wrapped around data analytics, the collection of data, the storage of data, data, data is clearly the, the the holy grail of anything that's dealing with manufacturing industry, if I can see the data, I can make some decisions on that data. For the listeners, we got to the difference between data analytics, and decision size that get that right. data science, data analytics can help us so clarify, because I want to go down that road of really decision a science.
Cool, yeah, since we keep mentioning data science, right. I think this is an important topic. Whenever we talk about manufacturing, or IoT, industrial IoT, most of the talk is surrounding data science, right? However, I feel decision sciences of equal or even more importance, let's really try to break it down, right? Data science is analysis of the data that you have to bring out insights. And it's as simple understanding, from a simple understanding purpose, what we can do is we can assume data science to be a bottom up approach. So there is data, we analyze it, and we get some insights out of this right. Decision Science, on the other hand, is a top down approach where our priority is to focus on the business problem at hand, and then come up with multiple alternatives which can solve that business problem. And these alternatives, then get validated by data science, and then tell us maybe what is the valuable or most valuable path to resolve
this problem? Can you decouple data science from decision science? Or are they? Because I get it? Because here's, here's sort of the typical conversation I have. Yeah, so we're collecting data, then that data goes into some sort of an algorithm of some sort that spits out some sort of information. It's at that point that somebody has to make a decision on what to do. Like there's a, there's a point of action or no action, but you still need that ability to be able to use the data in a way to make that decision. Science, right.
Yeah, so I think let's, in other words, let's try to understand this with simple equations, right? Yeah, data plus analysis, analysis of that data is equal to insights. Ready? Question number one, if we want to assume all that comes under this gamma tea, which should be called data science. Now pick those insights, attach context to those insights, that will give you a decision. So all that comes under this is decision science, and you take that decision and implement it, that will give you a business value. So that is the most important term, which is the holy grail, which we're trying to achieve at all times. I think, yeah, I can give you a simple example.
Yeah. Give me something. Yeah, please provide that example.
Right. So I mean, I'm currently working with a company a fashion brand focused on eyewear a North American company, they have almost four to 5000 asset entities, let's say no entities, by entities, I mean that there are stores that sell those eyewear, there are laboratories, where they do their research, there are manufacturing plants and there are offices corporate, regional, and so on and so forth. So, overall, there are 4000 to 5000 Odd entities and their objective is to cut down the spend, they are doing on electricity, right. So there are meters in smart meters of meters are installed. And they're measuring the consumption of electricity and the overall objective is to cut down that spend. Now, if you take a look at the objective, from a decision science perspective, we can really have multiple options right? Decision Science, the output of Decision Science will be well, can you really Have or migrate something to green energy or renewables a part of your spend? Can it be renewables? Can you have some efficient or energy efficient devices in your stores? Can you maybe negotiate with your vendors? So there are multiple options, which are available to really achieve the objective of reducing the energy spent that they're currently
doing? So yeah, so the objective is to reduce the energy spent, but there are multiple avenues to to consider and to deploy, to achieve whatever that is. It's not just it's not that the data, it's, it's, it's a holistic view of how you're going to make that decision, based off of your objective, whatever that might be. Is that Is that how you sort of wrap it
up? Yeah, absolutely. So there is a 360 degree view that has to be taken. And you need to understand what are the options? Right, somebody can deploy before getting into the data. Now, the the third part is the data now, which vendors maybe are more likely to give us some discount rate that that can be told by data, right? What is the fixed cost versus the variable cost if we move to renewables, that can be told by data? So after a certain point, to evaluate the options and to backer decision, their data science comes into play?
See, I see it, I do. Because if there are a lot of people who just think that data is it, I'm gonna just get the data, I just if I get the data, then I can make those decisions that are meaningful to whatever I'm trying, but I just need the data. But that's not that's not the the whole picture of of an organization or a business. And in fact, one can argue that if you're not looking at it holistically, then you're definitely missing out on potential opportunities. Right. Yeah. So okay, so with that said, in the case of the eyewear, right, when you're saying I mean, they, they appear to be vertically integrated, so they've got retail, they've got the whatever the other than the manufacturing and all of that stuff, and, and all of those that that vertically integrated model has to be able to communicate your data and all that stuff. But there's a lot more to it. How do you? How do you take something that, from my perspective, appears to be complex? Right, that vertical appears to be complex, and then be able to begin to take me through the journey of being able to know what to do and where to go and how to do it and tie it all together? Tell us talk to us about that.
So that I think is the journey of data science, right? We have lots of data coming in, we'll try to see if the data is clean, we'll try to improve the quality of the data.
By the way, just interrupt the data isn't clean, FYI, it's always dirty. Yeah.
Right. That's why we also have a validation layer that typically goes into checking whether data is clean or not. But as you mentioned, yes, the cleaning process itself, decides whether you're going to get anything meaningful out of that or not,
right, scrub it. Scrub that data, man, go continue. I digress, because I've been on many data cleaning initiatives, and none of them have been
flipped salutely Right. And I think in terms of utilities especially, so,
utilities, continue I'm sorry go.
So, obviously, once you have the clean data and whatever is not clean users have to throw it out because it should not skew our decision making right. Yes. So, once you have a certain level of data, which can be termed acceptable, what you actually do is build a data platform where you build context right you build a master data set where you know, which are the labs, which are the manufacturing plants, which are the stores and then tied to the factual data, which is essentially what is the consumption. So now, you will have a view which can be seen from multiple hierarchies, it can be aggregated, it can be disaggregated, you can project certain things you can say that okay, this year, my expenditure is x or usage is x and next year, my usage can be y. So all the decision science really comes after you have a clean data set. You have a connected data set, and you have a contextualized data set.
here and I run simulations. If I have clean data, let's just sort of assume the fact that my data has been scrubbed. And it's, it's squeaky clean and all of that good stuff, and it's meaningful. I want to run simulations. Do you allow that to happen within these, these engagements? Because I want to know, what if I do this? What if I do this? How can you do that?
Yeah, absolutely. I think that is very fundamental. And I think what we would like to call it as a what, what if analysis, where we run multiple scenarios, and we are also able to, we're able to tweak whatever inputs we want. And this, we can say, let's say if I want to open X number of stores in the next year, in a particular region, so then what would be my spend? And what would be my bargaining power? If I were to negotiate with the vendor who is providing me that particular energy in that particular region? So the what if scenarios are very, very important? And I think that's the crux really, of data science, or the starting point issues.
Yeah, and I agree, I absolutely agree with that. And in the case of the eyewear company that were chirping on, when when you deploy a and correct me from the terminology, I see this map, this, this integrated map of being able to sort of pull it all together. And it's, it can be simple, complex, whatever, but I see a map. Do you ever go back and analyze or real time look at where you're at, in reference to your, what you, you, you indicate in the data?
Yeah, so real time is essential in most IoT, industrial IoT projects. But in this particular case, I think it's not necessary because the spend is really track maybe weekly, sometimes daily. But for the senior guys, it's only about a monthly spend, even though it's 10s. of millions. True, it's it's Strack monthly in this case. But yes, as you said, if there are sensors, if there are things that are getting affected in real time, for example, solar energy generation rate, so somebody generating solar energy, needs to know what is the energy available to me, so that I can sell it at the best possible price to the grids. So that's a different topic. Again,
I'll tell you, these are these are not, this is not easy. In and I would imagine, and again, I see the spider diagram, this thing exists, it's all over. Do you ever approach clients? From an incremental perspective, you're saying, Hey, I glaspie, you've got a gazillion stores and you're vertically integrated. And we're trying to pull this that the other thing out of it. But we can start here? Do you ever get some wins here as opposed to Big Bang? And everybody? Everybody hates you?
Yeah, that's a I think, very relevant question. So, I think I always wanted to touch upon that. What typically happens is when we talk of IoT or data, we only see a single dimension that will have data will have analytics will have visualization will have machine learning and will move up that maturity curve. But recent experience that I had I had with one of the companies, right, they are into non destructive testing. And they obviously, that is through sonar, where they test automobiles in all the other sectors. Now, typically, although their machines are smart, their challenge is really how can we quickly get our machine up and running? How can we register our machine fast? How can we reboot or establish that bi directional communication where we can unlock certain packages for our users to use. So let's say they want to access certain functionality, I should not push my person into their premise and activate that device or activate that package and then they will start to use it. So it might not be related to a very data centric use case. But this is a smart machine, which really needs to focus on quick activation, Quick Calibration, and all that stuff, which is related to the setup.
That's a whole Yeah, that's a whole nother conversation and in especially within industry, where, you know, many of these engagements can sort of fester and carry on for some time. Know I want I want action I want I want speed to implementation and seeing, you know, results in solutions being deployed. I like that. We used the eyeglass example, are there other industries that same sort of the same thing? It's not just manufacturing, it can be other industries, right?
Yeah. So maybe I can touch on a very different example. It's not related to revenue. So a water meter company we are working with, and they sell water meters to the water meter utility company. Now, the focus of utility companies to encourage people to use water responsibly, right, so that's a typical objective or business value for them. Now, again, how decision science comes into play in that context is you can educate people to use water responsibly, that is maybe option number one, you can enforce laws and say that you cannot use water beyond this point. That is another option. Or you can also with the data science or data that we have, you can also give them access to their own data, and let them see what the usage is, the pattern is, and then allow them to learn from that and then encourage them to use water responsibly. So again, it's a holistic view of the problem. And there are, let's say, these are the top three options. Now we can go to data science and tell that what is the percentage when it or percentage of successful projects when we try to enforce law? What is the success percentage when we try to educate customers? And maybe what is the success percentage when we really try to give them their own data? And then ask them to
add it? Have you done that? Because, you know, I know me as a consumer Don't, don't not don't want Don't give me data? Don't don't? I mean, because I'm not gonna look at it. It's my house. Right? So what were there any results from that? Those? Yeah,
absolutely. So like, what third approach was finally implemented. The last level of granular data is available through the household users through an app. And based on the flow of water, which is monitored by water meter, we have applied machine learning on that. And we are able to guess, whether this water is from Bath, or this water is from a sink, and so on, and so forth.
Wow. And then people wanted to get that data and that information to make these decisions.
Yeah, and Decision Science is not only incorporated from a 360 degree view of the problem, rather, we have also incorporated it in design. So what that means is, if it is incorrectly marked as bad, the user has an ability to go there and change that entry, and mark it as sync. So that turns out good data and gives us great accuracy.
With that, with that said via the the this water meter company sort of begin to sort of collect all of the data and saying, Hey, there's a lot of waste going down the sink or a lot of whatever it is that that what happens
when that happens, and the utilities get benefit out of it. Obviously, yeah, they can infer certain laws, and they can take certain action in case things go was
Wow, Brave New World don't come don't come to Louisiana because well, we're always in water in some way, shape, or form. Sorry to say, and I don't use that water. All right. So I'm a big fan of that. I see it I understand what what you are doing. What are the roadblocks? What are the resistance? Why Why would people push back on something just FYI, listener, video watcher, pointing at you? It's, it's gonna happen. So you figure this out. So what what would be the real resistance in the pushback?
So I think I would have would have to use a cliched statement. So technology is changing at a rapid pace. And I think it's difficult to keep a track of it. I mean, seriously, it's changing so fast. So what typically happens is, maybe we misjudged or we don't really judge what is possible with a technology standpoint, and then companies tend to really focus on operational or day to day issues over this strategic technology issues. And they remain on the back burner. That is probably one issue, which I think exists.
Another always people, it's always people, by the way, it's always the human side and the technologies, the technologies it's always He's just sort of, I prioritize this way. And I do that, but I'm sorry, I interrupted you.
No, no, that is maybe the important part. But second, maybe reason I generally find is everybody's interested, right. Everybody wants to know, technology. Everybody wants to implement it in their business. But there is an interested versus committed dilemma, right. I am very interested in implementing something that benefits my organization. But does it come from the top? Is my top management fully committed to this? There? It's no maybe it's annoyed to me. Yeah.
Yeah. It's squiffy. Whatever, yeah, no, you're absolutely right. Well, I hear you. Let's wrap it up here. How do people get a hold of you? If they want to say, hey, I want to know more. I want to know more about you. And what you can do?
Yeah, I mean, on a personal level, obviously, you can reach out on LinkedIn. I'm sure you will have a link in the description of this video. Yeah, and I'm shameless. At an our level of this, the they can visit the website of a company where we have lots of case studies. And there is a lot of information and knowledge around IoT and this space.
You are wonderful tennis man. You are absolutely great. All right, listeners. We're gonna wrap it up on the other side, we're going to have all the contact information for Bhushan boosh shot out on Industrial Talk as well as everything that you need got to reach out to him. All right, we'll be right back.
You're listening to the Industrial Talk Podcast Network.
All right, ladies and gents, that was Bhushan Saviant Consulting, reach out to them, they are all going to all the contact information will be out on Industrial Talk. So if you're not reach out, decision science is where it's at. It's one thing to be able to collect all that data Absolutely. Spot on, must do. Take the next step. What do we do with that information? How do we make those tactical decisions that benefit your manufacturing process, and so on and so forth? All right, again, building an ecosystem, an industrial ecosystem of problem solvers. You need to be a part of it. You need to be a part of it and make that a priority. Just go out to Industrial Talk.com Subscribe to the channels, subscribe to the podcast, and you'll get the latest and greatest because you need to succeed. Be bold, be brave, dare greatly hang out with Bhushan and change the world. We're gonna have another great conversation shortly so stay tuned.