Bill Schmarzo with Hitachi Vantara and Kirk Borne with Booz Allen Hamilton talk Data Analytics And Impact to Business and Culture
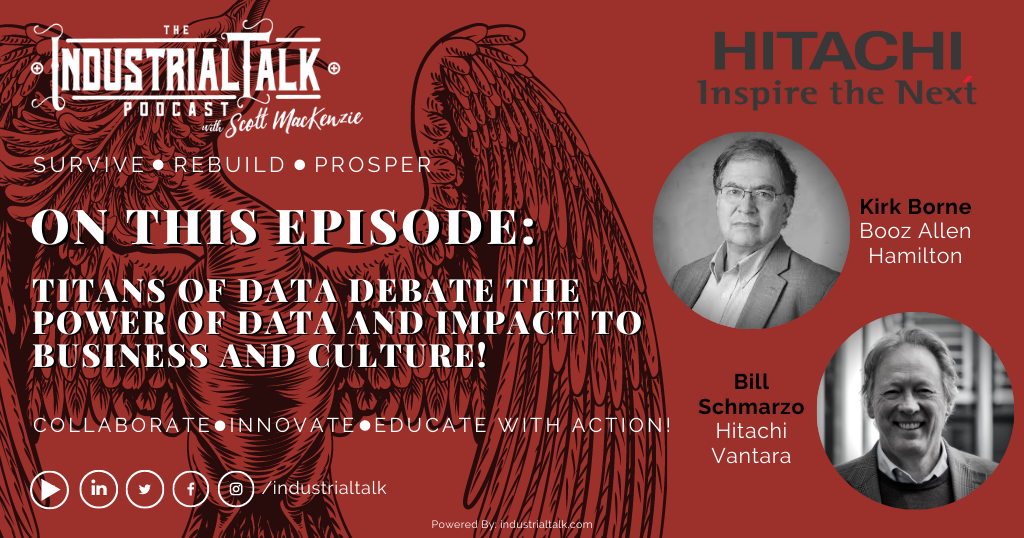
In this week's Industrial Talk Podcast we're talking to the Titans of Data, Bill Schmarzo, Chief Innovation Officer with Hitachi Vantara and Kirk Borne, Data Science Fellow and Executive Advisor at Booz Allen Hamilton about “The Power of Data and Impact to Business and Culture”. Get your answers to the real power behind data analytics along with Bill's and Kirk's unique insight on the “How” on this Industrial Talk interview!
You can find out more about Bill and Kirk and the wonderful team at Hitachi Vantara and Booz Allen Hamilton at the links below. Finally, get your exclusive free access to the Industrial Academy and a series on “Why You Need To Podcast” for Greater Success in 2020 and beyond. All links designed for keeping you current in this rapidly changing Industrial Market. Survive! Rebuild! Prosper!
BILL SCHMARZO'S CONTACT INFORMATION:
Personal LinkedIn: https://www.linkedin.com/in/schmarzo/
Company LinkedIn: https://www.linkedin.com/company/hitachi-vantara/
Company Facebook: https://www.facebook.com/HitachiVantara
Company Website: https://www.hitachivantara.com/en-us/home.html
Company Twitter: https://twitter.com/HitachiVantara
Personal Twitter: https://twitter.com/schmarzo
KIRK BORNE'S CONTACT INFORMATION:
Personal LinkedIn: https://www.linkedin.com/in/kirkdborne/
Company LinkedIn: https://www.linkedin.com/company/booz-allen-hamilton/
Company Facebook: https://www.facebook.com/boozallen
Company Website: https://www.boozallen.com/
Company Twitter: https://twitter.com/BoozAllen
Personal Twitter: https://twitter.com/KirkDBorne
EVENTS TO PUT ON YOUR CALENDAR:
Deliver on bottom line, Realize the economic value of data: Register Here!
Speed Discovery, Comprehension and Trust in Data at Scale: Register Here!
Adopting to Corona Virus Supply Chain Disruptions: Register Here!
PODCAST VIDEO:
THE STRATEGIC REASON “WHY YOU NEED TO PODCAST”:
OTHER GREAT INDUSTRIAL RESOURCES:
Safeopedia: https://www.safeopedia.com/
Industrial Marketing Solutions: https://industrialtalk.com/industrial-marketing/
Industrial Academy: https://industrialtalk.com/industrial-academy/
Industrial Dojo: https://industrialtalk.com/industrial_dojo/
Safety With Purpose Podcast: https://safetywithpurpose.com/
YOUR INDUSTRIAL DIGITAL TOOLBOX:
LifterLMS: Get One Month Free for $1 – https://lifterlms.com/
Active Campaign: Active Campaign Link
BombBomb: BombBomb Link
Social Jukebox: https://www.socialjukebox.com/
Industrial Academy (One Month Free Access And One Free Licence For Future Industrial Leader):
Business Beatitude the Book
Do you desire a more joy-filled, deeply-enduring sense of accomplishment and success? Live your business the way you want to live with the BUSINESS BEATITUDES…The Bridge connecting sacrifice to success. YOU NEED THE BUSINESS BEATITUDES!
TAP INTO YOUR INDUSTRIAL SOUL, RESERVE YOUR COPY NOW! BE BOLD. BE BRAVE. DARE GREATLY AND CHANGE THE WORLD. GET THE BUSINESS BEATITUDES!