John Harrington with HighByte
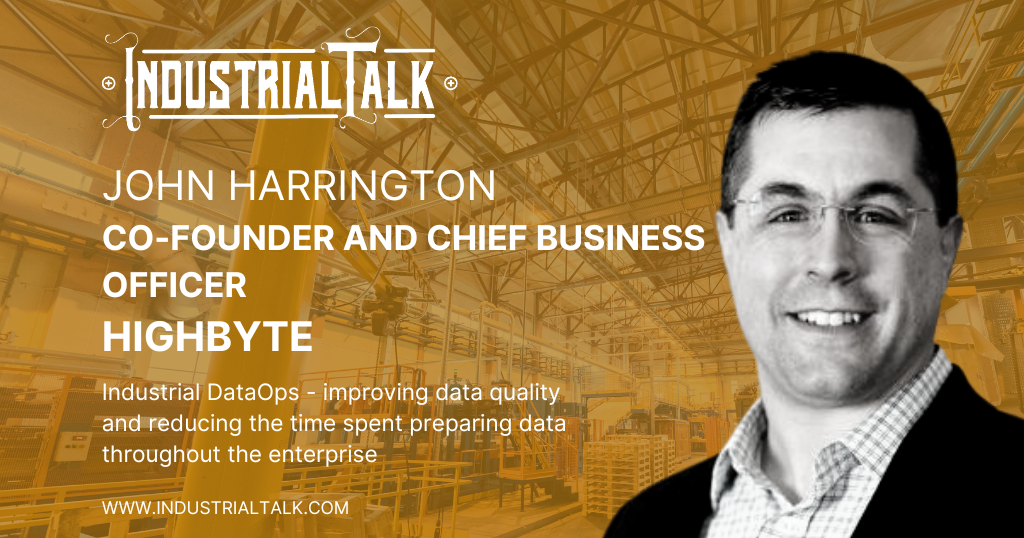
On this week's Industrial Talk we're onsite at the Digital Manufacturing Summit and talking to John Harrington, Co-Founder and Chief Product Officer at HighByte about “Improving operational data quality in less time across your enterprise”. Get the answers to your “DataOps” questions along with John's unique insight on the “How” on this Industrial Talk interview!
Finally, get your exclusive free access to the Industrial Academy and a series on “Why You Need To Podcast” for Greater Success in 2022. All links designed for keeping you current in this rapidly changing Industrial Market. Learn! Grow! Enjoy!
JOHN HARRINGTON'S CONTACT INFORMATION:
Personal LinkedIn: https://www.linkedin.com/in/john-harrington-142906a/
Company LinkedIn: https://www.linkedin.com/company/highbyte/
Company Website: https://www.highbyte.com/
PODCAST VIDEO:
THE STRATEGIC REASON “WHY YOU NEED TO PODCAST”:
OTHER GREAT INDUSTRIAL RESOURCES:
NEOM: https://www.neom.com/en-us
Hitachi Vantara: https://www.hitachivantara.com/en-us/home.html
Industrial Marketing Solutions: https://industrialtalk.com/industrial-marketing/
Industrial Academy: https://industrialtalk.com/industrial-academy/
Industrial Dojo: https://industrialtalk.com/industrial_dojo/
We the 15: https://www.wethe15.org/
YOUR INDUSTRIAL DIGITAL TOOLBOX:
LifterLMS: Get One Month Free for $1 – https://lifterlms.com/
Active Campaign: Active Campaign Link
Social Jukebox: https://www.socialjukebox.com/
Industrial Academy (One Month Free Access And One Free License For Future Industrial Leader):
Business Beatitude the Book
Do you desire a more joy-filled, deeply-enduring sense of accomplishment and success? Live your business the way you want to live with the BUSINESS BEATITUDES…The Bridge connecting sacrifice to success. YOU NEED THE BUSINESS BEATITUDES!
TAP INTO YOUR INDUSTRIAL SOUL, RESERVE YOUR COPY NOW! BE BOLD. BE BRAVE. DARE GREATLY AND CHANGE THE WORLD. GET THE BUSINESS BEATITUDES!
Reserve My Copy and My 25% Discount
PODCAST TRANSCRIPT:
SUMMARY KEYWORDS
data, problem, asset, manufacturing, collect, people, pull, systems, analytics, industrial, case, chief product officer, traceability, create, john, vibration sensor, facilitate, high, solve, scaling
Welcome to the industrial talk podcast with Scott Mackenzie. Scott is a passionate industry professional dedicated to transferring cutting edge industry focused innovations and trends while highlighting the men and women who keep the world moving. So put on your hard hat, grab your work boots, and let's go.
Well, welcome to industrial talk. Thank you very much for joining the number one industrial related podcast in the universe as celebrates industry professionals, all around the world because you're bold, you're brave. You dare greatly you innovate. You solve problems, you're changing my lives, you're changing lives of other people and you're changing the world. Why not celebrate you? We are also broadcasting from the digital manufacturing symposium right here. Chicago, great venue, incredible collection of I want to say professionals that want to solve problems for you. Yeah. They're dedicated to solving your problems. And they're willing to collaborate. Yeah, they're, they're great. They're, they're trailblazers. All right. In the hot seat. We have a gentleman by the name of John Harrington. HighByte is the company. He'll look at him. He's the co founder. I'm looking at his business card. He's the co founder and chief product officer. He is a big week. He's a big cheese. Alright, John, how you doing?
I'm doing great. How you doing?
Huh? No, no complaints? None whatsoever. You wouldn't listen to me if I was complaining. What you? Would you listen to me? We'd love it. We love it. Anyway, it comes. Anyway, anyway, the complaining comes we love it. But that's the content. That's what we want quality. Human content. That's
right. Now that is fluffy stuff. Now know,
why would you? Alright, you having a good conference? It's been a great day. It's been pretty cool. Yeah. Well, Buzz,
lots of exciting discussions.
See, I'm sort of digging it. I don't know why I'm digging it. I was in a big conference. And then I'm, this was sort of more boutique ish.
It is. It's much more if I could use the term intimate. Because you can talk with people, you can have conversations, yes, you can understand what they're doing. It's not you know, I was talking to someone today I said, I've been in a conference of 40,000 people and talked with fewer people than than this conference today. Because people want to talk they want to learn
to that's pretty cool. That's that's that's a testament. Yeah. I agree with you. I think it's it's a must. All right. Before we get into the topic, before we have that conversation, give us a little background on who you are.
Yeah, so John Harrington, I'm the Chief Product Officer and one of the cofounders of high bite. I've been in the manufacturing and manufacturing technology industry, my entire career. First 10 years, I was working in software companies that were in the CAD and procurement space selling into manufacturing. Then I got in spent five years out of manufacture. So really sat in the seat and felt it. And then in the last 15 years, I've been back in software and technology for manufacturing companies.
Clearly, you're smarter than I am. I wouldn't go there. No, yes, I would you got more hair than I do. And I'll go there too.
I do have that.
Much of a high bar right there. All right. So hi, by b y, t YT. Okay, what is the problem you're addressing? Here?
We are addressing data, the data problem? Data is the challenge. You know, it's great. I did a talk at the show at about 230 in the afternoon. In every talk before me talked about the challenges. How do we get data? How do we get the right data? How do we put the right data in the right person's hands? And then I came in and started talking about how you doing? There you go. That's, that's what Hivites looking to do.
It's interesting, because you brought up a good point. It's it's one thing to collect data, I think we can all collect data, I can collect data, collect data all the time we can it is how do you take that data? And and I didn't think through that enough, where I was saying, Well, who gets the data? Where does it go? All of that. That's, that's pretty important.
What problem are we looking to solve? And that's where it has to start. It has to start with what is the problem? Is the problem, a quality problem trying to improve quality on the line? Or is the problem in an asset uptime problem we need to do some predictive asset maintenance so that we can improve our our uptime of an asset so that the line doesn't stop? or is the problem a traceability problem? If we're not collecting data, then we might as well scrap all of the parts. Because it's a regulated approach. It's all about understanding the problem, and then creating solutions to deliver the data to solve that problem.
So, so with that, are you are we talking about an algorithm? Are we talking about? Where does Where does high bike fit into that whole? You know, model?
Yep, yep. No, that's great question and models a great term to, and I'll get to that. But you're welcome. You're setting me
up for success.
So we call it industrial data ops, which is the preparation, curation and delivery of data for analytics visualization for whatever the use case is. So it's not so much the analytic it's, it's everything that needs to happen, but for the analytic gets the data, right. And it's how do we get the data from wherever it is, and often it's not just, Well, there's a PLC on the factory floor i sensor that I say to people, you know, predictive maintenance. One Dotto was about putting a vibration sensor on a motor and when the vibration sensor went too high, we knew there was a problem. Predictive Maintenance to Dotto was about combining the sensor information with what was going on on the line at that time. Yeah. So that if it goes high, but it's supposed to go high, then we know it's a good thing. But if it goes high, and it's supposed to be idle, then we know it's a bad thing. Predictive Maintenance three Dotto is about combining that with who is the customer that we're producing this product for? What product are we producing? What asset are we producing it on? And what's the the asset history, the service history of that, combining all that data together so that we can really start driving knowledge?
Okay, so we've got these, these devices out there, whatever they might be, got it, go out here, pub,
we got a motor and a pump,
or whatever. And, and, and what you're facilitating is I'm collecting data does, do you get rid of some of the bad data? Does does it have you as like, Okay, we want this data, we don't want that data, let's get and be able to manage the volume because it can be a data tsunami. And I still, it's massive,
yes, tsunami is a great word Towards Data noise, noise, just data noise, it's too much. And we what we need is, if we're doing predictive asset maintenance on this pump, we want to pull some vibration sensor that we just put on there to it's, it's, it's a wireless sensor that's pushing back over some other network, we want to pull the industrial automation data, we want to pull some information out of the asset maintenance system. But we need to know that the the pump that we're monitoring is, you know, we need to reference that in the asset maintenance system. We want to pull from the SAP system, what order we're working on while we're producing all this. And we want to pull all that data together into a package of payload. Right? So in real time, we can pull data from different systems, package it together, and then put it up wherever you want. The big. Two big things that people are talking about today are moving data to the cloud. Yeah. Azure, AWS in a data lake. Yeah. The other thing is this concept of a unified namespace. Do you heard about the unified namespace?
No. I mean, I want to I want to now
unified namespace. A lot of people are deploying a protocol called MQ, TT and MQ to broker on premise. And they're publishing data into it in they're organizing that database on the IC 95 specification. So you have some site level data, like maybe profitability, and then you have some area level data, like what's our schedule, and then you have line level data, like what's the OEE. And then you've got work cell level data, like, what's some traceability information on the parts being produced, and then you've got the asset and we have asset level data. So it's creating this definition of all the different types of data and then placing it in there and then allowing the people in your company to be able to access that by subscribing to the data so that it It really democratizes data within a factory environment or within a corporation.
So the picture that's in my humble, feeble mind, is my May, you're able to, and I'll use it correct me if I'm wrong. aggregate the data is from various legacy systems, new systems, it, there's just tons of systems out there collecting data, right, and then be able to pull it into a central location, organize it in a way that makes sense structure it for you, for use case, essentially
transform it, sometimes you need some algorithm on it, simple algorithms, we're not talking to analytics, yet, this no doesn't deliver the data, or the analytic, maybe you need to filter it, or you need to aggregate it for a period of time and then provide like an average or standard deviation. Yeah. So create those data payloads based on what's going to use it and then publish them into a central location for anyone to get access to
that anyone or any systems within the company security, that's right, get access to this data. And then and then from that, because you've already sort of, for lack of a better term, scrubbed it connected up, yep, put it in the right order, read it curated all of that good stuff, so that whatever systems I have, or what I have in place, it's just like, grab, go, analyze, do the data analytics, whatever that might be, and then be able to spit out a, you know, an answer and answer some sort of solution.
Now the key is with manufacturing data, we start out looking at historical, to create analytics, we're not but a lot of time, we're looking at real time data. So it's streaming, it's not like in the IT world, they often will have transactions, but in the manufacturing world, we have streaming data, things are changing in real time. And we need to know you know, some data, we may be capturing once a millisecond other data once a second and other data once every 10 minutes, or when the batch is complete. So it's it's critical to to understand those differences, and it's very different than the IT environment.
Yeah. So that brings up a question of, if I'm collecting data at various rates, and and Do you can you can you take the different sources of data with different time requirements, and paint a picture within your service and okay, I, this motor, to this transformer to this, whatever, pull it together and create a different sort of a different picture. That's the magic that there it isn't.
Yes, in what it is, based off the use case. Yeah, ultimately, you always have to start with what are we trying to solve for we try, again, quality, asset maintenance. traceability, r&d has some needs for for their own system. So you start with the use case. And that often dictates that not only the data, but the frequency that of the update,
which then paints a picture that that how I how I'm processing it, is that I have a high level of confidence in all of this data coming in, I'm not being distracted by noise. I am really, and then it's all being driven by the use case, which is all developed at the beginning. Do you facilitate those conversations? Like okay, we got it, let's let's, what what problem are we solving? Okay, I got it. And then you got this, this source of data, this source of data, this source of data, let's look at that. And then you come up with that plan of attack. And then you pay do that.
So we're a software provider, but we usually facilitate the first few conversations along those lines. So helping them helping the customer understand how to think about this, how to structure it, working with them on the first few use cases. And then, you know, that's actually when the magic happens, because that's when they get excited. Yeah, once they start seeing the data flowing, and they start solving problems. They get excited, and you know, more and more use cases come up and they start using software even more. And you know, it's designed to be highly scalable, because scalability is all this stuff can be done. One use case in simple one device at a time. But when you try to scale that out, do you replicate all of the work the work? The work is a linear scale, in that key is to asset number two should be significantly easier asset number three even easier. And in the same data set should be used, the same analytics should be used if they're like assets. So that's what we really facilitate. That's the benefit of doing this in, in an application versus just custom hacking together some scripts to jam some code up, you know, up into up into Azure and figure it out, best to structure the data, and then deliver it as a payload. And then analysts can understand exactly what they have, and put it to use much faster. So we're, you know, we're saving money, we're saving money in storage, we're saving money and transport, we're saving time projects are scaling faster, and they're actually scaling as opposed to a lot of these projects just haven't scaled the way people had hoped.
And that's a whole nother conversation. I mean, that's that credibility side of what, I don't think that there's any debate on the necessity to collect data. And it's, it's, it's, it's the how, and what's important, and will I just give, you know, will I be drowning? So once you structure the data, then that data is accessible by Oh, T means executive means whatever,
whatever their business, any different department within a corporation, and he has access to it.
Yeah, whatever dashboards, you could probably say, I like red, green and yellow. Green, good, red, bad yellow, better look at it, that type of thing. Yeah, I get it. I get it. I think it's, I never thought about it. But I think that I get it. Because, you know, I've been a part of projects where yeah, we can collect data, then what, what it what are you gonna do with it? What cash? Is it good? And it just sort of implodes on itself? Because, oh, do this anymore. I can't figure it out. Yep.
There's no use case. The other thing that we hear from people is we, we just want to push all the data up to up into the cloud, and we'll let them deal with it. Right? They say in manufacturing, there is no all in data. There are manufacturing plants with millions of data tags. And of course, with the with the automated equipment, you can collect it as fast as you want millisecond resolution. So there's no all it's not like a database, which with a bunch of transactions, you can literally say, alright, let's just lift and shift a database up into the cloud and just, you need to define what you want. You need to be specific, and then that's when you get success.
You're gonna laugh, I think it's cool. I'm Ken, I'm not easily persuaded. Oh, yeah. I just lied to you. All right. So listeners out there saying, yes, he's speaking my language. How do they get ahold?
www.fbi.com or they can email me John dot Harrington high buy.com. Or look me up on LinkedIn.
You are fantastic. smarter than I am. Which is pretty good. Understand, that's a low hurdle. Alright, listeners, we're gonna wrap it up on the other side, you're gonna have all the contact information for John and that incredible company HighByte. So fear not. So you have no excuse for not contacting John. All right, we will be right back with another great conversation. So stay tuned.
You're listening to the industrial talk Podcast Network.
All right. Once again, thank you very much for joining industrial talk, as you could tell by the noise in the background, we were on site at the digital manufacturing symposium. And that was in Chicago. And it's a great event, you got to you got to put that on your calendar for next year. Absolutely make that happen. Because you're able to connect with individuals and companies that are truly committed to this digital transformation of manufacturing. And quite honestly, it's a must if you want to create a build business that is resilient, definitely, digitally transform it. All right. And also thank you to John Harrington and team HighByte that's being y t. E. Put that down on your things to do to connect. And if you go out to high buy.com, you'll find this guy just read guide about data modeling guide book, and it's best practice if you need it. You need to be able to navigate these digital transformation waters. It's important and it starts with insights in this guidebook is absolutely right there right there. So anyway, go out to industrial talk, get engaged, be a part of this expanding ecosystem. It's it. We want to collaborate. We want to innovate, and we definitely want to educate. And that starts with you. Thank you very much. So, a people will be brave, dare greatly hang out with people like John and you're going to change the world. Thank you very much for joining once again, and we're going to have another great conversation shortly. So stay tuned.
Transcript
Welcome to the industrial talk podcast with Scott Mackenzie. Scott is a passionate industry professional dedicated to transferring cutting edge industry focused innovations and trends while highlighting the men and women who keep the world moving. So put on your hard hat, grab your work boots, and let's go.
Well, welcome to industrial talk. Thank you very much for joining the number one industrial related podcast in the universe as celebrates industry professionals, all around the world because you're bold, you're brave. You dare greatly you innovate. You solve problems, you're changing my lives, you're changing lives of other people and you're changing the world. Why not celebrate you? We are also broadcasting from the digital manufacturing symposium right here. Chicago, great venue, incredible collection of I want to say professionals that want to solve problems for you. Yeah. They're dedicated to solving your problems. And they're willing to collaborate. Yeah, they're, they're great. They're, they're trailblazers. All right. In the hot seat. We have a gentleman by the name of John Harrington. HighByte is the company. He'll look at him. He's the co founder. I'm looking at his business card. He's the co founder and chief product officer. He is a big week. He's a big cheese. Alright, John, how you doing?
I'm doing great. How you doing?
Huh? No, no complaints? None whatsoever. You wouldn't listen to me if I was complaining. What you? Would you listen to me? We'd love it. We love it. Anyway, it comes. Anyway, anyway, the complaining comes we love it. But that's the content. That's what we want quality. Human content. That's
right. Now that is fluffy stuff. Now know,
why would you? Alright, you having a good conference? It's been a great day. It's been pretty cool. Yeah. Well, Buzz,
lots of exciting discussions.
See, I'm sort of digging it. I don't know why I'm digging it. I was in a big conference. And then I'm, this was sort of more boutique ish.
It is. It's much more if I could use the term intimate. Because you can talk with people, you can have conversations, yes, you can understand what they're doing. It's not you know, I was talking to someone today I said, I've been in a conference of 40,000 people and talked with fewer people than than this conference today. Because people want to talk they want to learn
to that's pretty cool. That's that's that's a testament. Yeah. I agree with you. I think it's it's a must. All right. Before we get into the topic, before we have that conversation, give us a little background on who you are.
Yeah, so John Harrington, I'm the Chief Product Officer and one of the cofounders of high bite. I've been in the manufacturing and manufacturing technology industry, my entire career. First 10 years, I was working in software companies that were in the CAD and procurement space selling into manufacturing. Then I got in spent five years out of manufacture. So really sat in the seat and felt it. And then in the last 15 years, I've been back in software and technology for manufacturing companies.
Clearly, you're smarter than I am. I wouldn't go there. No, yes, I would you got more hair than I do. And I'll go there too.
I do have that.
Much of a high bar right there. All right. So hi, by b y, t YT. Okay, what is the problem you're addressing? Here?
We are addressing data, the data problem? Data is the challenge. You know, it's great. I did a talk at the show at about 230 in the afternoon. In every talk before me talked about the challenges. How do we get data? How do we get the right data? How do we put the right data in the right person's hands? And then I came in and started talking about how you doing? There you go. That's, that's what Hivites looking to do.
It's interesting, because you brought up a good point. It's it's one thing to collect data, I think we can all collect data, I can collect data, collect data all the time we can it is how do you take that data? And and I didn't think through that enough, where I was saying, Well, who gets the data? Where does it go? All of that. That's, that's pretty important.
What problem are we looking to solve? And that's where it has to start. It has to start with what is the problem? Is the problem, a quality problem trying to improve quality on the line? Or is the problem in an asset uptime problem we need to do some predictive asset maintenance so that we can improve our our uptime of an asset so that the line doesn't stop? or is the problem a traceability problem? If we're not collecting data, then we might as well scrap all of the parts. Because it's a regulated approach. It's all about understanding the problem, and then creating solutions to deliver the data to solve that problem.
So, so with that, are you are we talking about an algorithm? Are we talking about? Where does Where does high bike fit into that whole? You know, model?
Yep, yep. No, that's great question and models a great term to, and I'll get to that. But you're welcome. You're setting me
up for success.
So we call it industrial data ops, which is the preparation, curation and delivery of data for analytics visualization for whatever the use case is. So it's not so much the analytic it's, it's everything that needs to happen, but for the analytic gets the data, right. And it's how do we get the data from wherever it is, and often it's not just, Well, there's a PLC on the factory floor i sensor that I say to people, you know, predictive maintenance. One Dotto was about putting a vibration sensor on a motor and when the vibration sensor went too high, we knew there was a problem. Predictive Maintenance to Dotto was about combining the sensor information with what was going on on the line at that time. Yeah. So that if it goes high, but it's supposed to go high, then we know it's a good thing. But if it goes high, and it's supposed to be idle, then we know it's a bad thing. Predictive Maintenance three Dotto is about combining that with who is the customer that we're producing this product for? What product are we producing? What asset are we producing it on? And what's the the asset history, the service history of that, combining all that data together so that we can really start driving knowledge?
Okay, so we've got these, these devices out there, whatever they might be, got it, go out here, pub,
we got a motor and a pump,
or whatever. And, and, and what you're facilitating is I'm collecting data does, do you get rid of some of the bad data? Does does it have you as like, Okay, we want this data, we don't want that data, let's get and be able to manage the volume because it can be a data tsunami. And I still, it's massive,
yes, tsunami is a great word Towards Data noise, noise, just data noise, it's too much. And we what we need is, if we're doing predictive asset maintenance on this pump, we want to pull some vibration sensor that we just put on there to it's, it's, it's a wireless sensor that's pushing back over some other network, we want to pull the industrial automation data, we want to pull some information out of the asset maintenance system. But we need to know that the the pump that we're monitoring is, you know, we need to reference that in the asset maintenance system. We want to pull from the SAP system, what order we're working on while we're producing all this. And we want to pull all that data together into a package of payload. Right? So in real time, we can pull data from different systems, package it together, and then put it up wherever you want. The big. Two big things that people are talking about today are moving data to the cloud. Yeah. Azure, AWS in a data lake. Yeah. The other thing is this concept of a unified namespace. Do you heard about the unified namespace?
No. I mean, I want to I want to now
unified namespace. A lot of people are deploying a protocol called MQ, TT and MQ to broker on premise. And they're publishing data into it in they're organizing that database on the IC 95 specification. So you have some site level data, like maybe profitability, and then you have some area level data, like what's our schedule, and then you have line level data, like what's the OEE. And then you've got work cell level data, like, what's some traceability information on the parts being produced, and then you've got the asset and we have asset level data. So it's creating this definition of all the different types of data and then placing it in there and then allowing the people in your company to be able to access that by subscribing to the data so that it It really democratizes data within a factory environment or within a corporation.
So the picture that's in my humble, feeble mind, is my May, you're able to, and I'll use it correct me if I'm wrong. aggregate the data is from various legacy systems, new systems, it, there's just tons of systems out there collecting data, right, and then be able to pull it into a central location, organize it in a way that makes sense structure it for you, for use case, essentially
transform it, sometimes you need some algorithm on it, simple algorithms, we're not talking to analytics, yet, this no doesn't deliver the data, or the analytic, maybe you need to filter it, or you need to aggregate it for a period of time and then provide like an average or standard deviation. Yeah. So create those data payloads based on what's going to use it and then publish them into a central location for anyone to get access to
that anyone or any systems within the company security, that's right, get access to this data. And then and then from that, because you've already sort of, for lack of a better term, scrubbed it connected up, yep, put it in the right order, read it curated all of that good stuff, so that whatever systems I have, or what I have in place, it's just like, grab, go, analyze, do the data analytics, whatever that might be, and then be able to spit out a, you know, an answer and answer some sort of solution.
Now the key is with manufacturing data, we start out looking at historical, to create analytics, we're not but a lot of time, we're looking at real time data. So it's streaming, it's not like in the IT world, they often will have transactions, but in the manufacturing world, we have streaming data, things are changing in real time. And we need to know you know, some data, we may be capturing once a millisecond other data once a second and other data once every 10 minutes, or when the batch is complete. So it's it's critical to to understand those differences, and it's very different than the IT environment.
Yeah. So that brings up a question of, if I'm collecting data at various rates, and and Do you can you can you take the different sources of data with different time requirements, and paint a picture within your service and okay, I, this motor, to this transformer to this, whatever, pull it together and create a different sort of a different picture. That's the magic that there it isn't.
Yes, in what it is, based off the use case. Yeah, ultimately, you always have to start with what are we trying to solve for we try, again, quality, asset maintenance. traceability, r&d has some needs for for their own system. So you start with the use case. And that often dictates that not only the data, but the frequency that of the update,
which then paints a picture that that how I how I'm processing it, is that I have a high level of confidence in all of this data coming in, I'm not being distracted by noise. I am really, and then it's all being driven by the use case, which is all developed at the beginning. Do you facilitate those conversations? Like okay, we got it, let's let's, what what problem are we solving? Okay, I got it. And then you got this, this source of data, this source of data, this source of data, let's look at that. And then you come up with that plan of attack. And then you pay do that.
So we're a software provider, but we usually facilitate the first few conversations along those lines. So helping them helping the customer understand how to think about this, how to structure it, working with them on the first few use cases. And then, you know, that's actually when the magic happens, because that's when they get excited. Yeah, once they start seeing the data flowing, and they start solving problems. They get excited, and you know, more and more use cases come up and they start using software even more. And you know, it's designed to be highly scalable, because scalability is all this stuff can be done. One use case in simple one device at a time. But when you try to scale that out, do you replicate all of the work the work? The work is a linear scale, in that key is to asset number two should be significantly easier asset number three even easier. And in the same data set should be used, the same analytics should be used if they're like assets. So that's what we really facilitate. That's the benefit of doing this in, in an application versus just custom hacking together some scripts to jam some code up, you know, up into up into Azure and figure it out, best to structure the data, and then deliver it as a payload. And then analysts can understand exactly what they have, and put it to use much faster. So we're, you know, we're saving money, we're saving money in storage, we're saving money and transport, we're saving time projects are scaling faster, and they're actually scaling as opposed to a lot of these projects just haven't scaled the way people had hoped.
And that's a whole nother conversation. I mean, that's that credibility side of what, I don't think that there's any debate on the necessity to collect data. And it's, it's, it's, it's the how, and what's important, and will I just give, you know, will I be drowning? So once you structure the data, then that data is accessible by Oh, T means executive means whatever,
whatever their business, any different department within a corporation, and he has access to it.
Yeah, whatever dashboards, you could probably say, I like red, green and yellow. Green, good, red, bad yellow, better look at it, that type of thing. Yeah, I get it. I get it. I think it's, I never thought about it. But I think that I get it. Because, you know, I've been a part of projects where yeah, we can collect data, then what, what it what are you gonna do with it? What cash? Is it good? And it just sort of implodes on itself? Because, oh, do this anymore. I can't figure it out. Yep.
There's no use case. The other thing that we hear from people is we, we just want to push all the data up to up into the cloud, and we'll let them deal with it. Right? They say in manufacturing, there is no all in data. There are manufacturing plants with millions of data tags. And of course, with the with the automated equipment, you can collect it as fast as you want millisecond resolution. So there's no all it's not like a database, which with a bunch of transactions, you can literally say, alright, let's just lift and shift a database up into the cloud and just, you need to define what you want. You need to be specific, and then that's when you get success.
You're gonna laugh, I think it's cool. I'm Ken, I'm not easily persuaded. Oh, yeah. I just lied to you. All right. So listeners out there saying, yes, he's speaking my language. How do they get ahold?
www.fbi.com or they can email me John dot Harrington high buy.com. Or look me up on LinkedIn.
You are fantastic. smarter than I am. Which is pretty good. Understand, that's a low hurdle. Alright, listeners, we're gonna wrap it up on the other side, you're gonna have all the contact information for John and that incredible company HighByte. So fear not. So you have no excuse for not contacting John. All right, we will be right back with another great conversation. So stay tuned.
You're listening to the industrial talk Podcast Network.
All right. Once again, thank you very much for joining industrial talk, as you could tell by the noise in the background, we were on site at the digital manufacturing symposium. And that was in Chicago. And it's a great event, you got to you got to put that on your calendar for next year. Absolutely make that happen. Because you're able to connect with individuals and companies that are truly committed to this digital transformation of manufacturing. And quite honestly, it's a must if you want to create a build business that is resilient, definitely, digitally transform it. All right. And also thank you to John Harrington and team HighByte that's being y t. E. Put that down on your things to do to connect. And if you go out to high buy.com, you'll find this guy just read guide about data modeling guide book, and it's best practice if you need it. You need to be able to navigate these digital transformation waters. It's important and it starts with insights in this guidebook is absolutely right there right there. So anyway, go out to industrial talk, get engaged, be a part of this expanding ecosystem. It's it. We want to collaborate. We want to innovate, and we definitely want to educate. And that starts with you. Thank you very much. So, a people will be brave, dare greatly hang out with people like John and you're going to change the world. Thank you very much for joining once again, and we're going to have another great conversation shortly. So stay tuned.